A Demand-driven, Proactive Tasks Management Model at the Edge
FUZZ-IEEE(2020)
摘要
Tasks management is a very interesting research topic for various application domains. Tasks may have the form of analytics or any other processing activities over the available data. One of the main concerns is to efficiently allocate and execute tasks to produce meaningful results that will facilitate any decision making. The advent of the Internet of Things (IoT) and Edge Computing (EC) defines new requirements for tasks management. Such requirements are related to the dynamic environment where IoT devices and EC nodes act and process the collected data. The statistics of data and the status of IoT/EC nodes are continuously updated. In this paper, we propose a demand- and uncertainty-driven tasks management scheme with the target to allocate the computational burden to the appropriate places. As the proper place, we consider the local execution of a task in an EC node or its offloading to a peer node. We provide the description of the problem and give details for its solution. The proposed mechanism models the demand for each task and efficiently selects the place where it will be executed. We adopt statistical learning and fuzzy logic to support the appropriate decision when tasks’ execution is requested by EC nodes. Our experimental evaluation involves extensive simulations for a set of parameters defined in our model. We provide numerical results and reveal that the proposed scheme is capable of deciding on the fly while concluding the most efficient allocation.
更多查看译文
关键词
Edge computing,Tasks management,Probabilistic model,Kernel Density Estimator
AI 理解论文
溯源树
样例
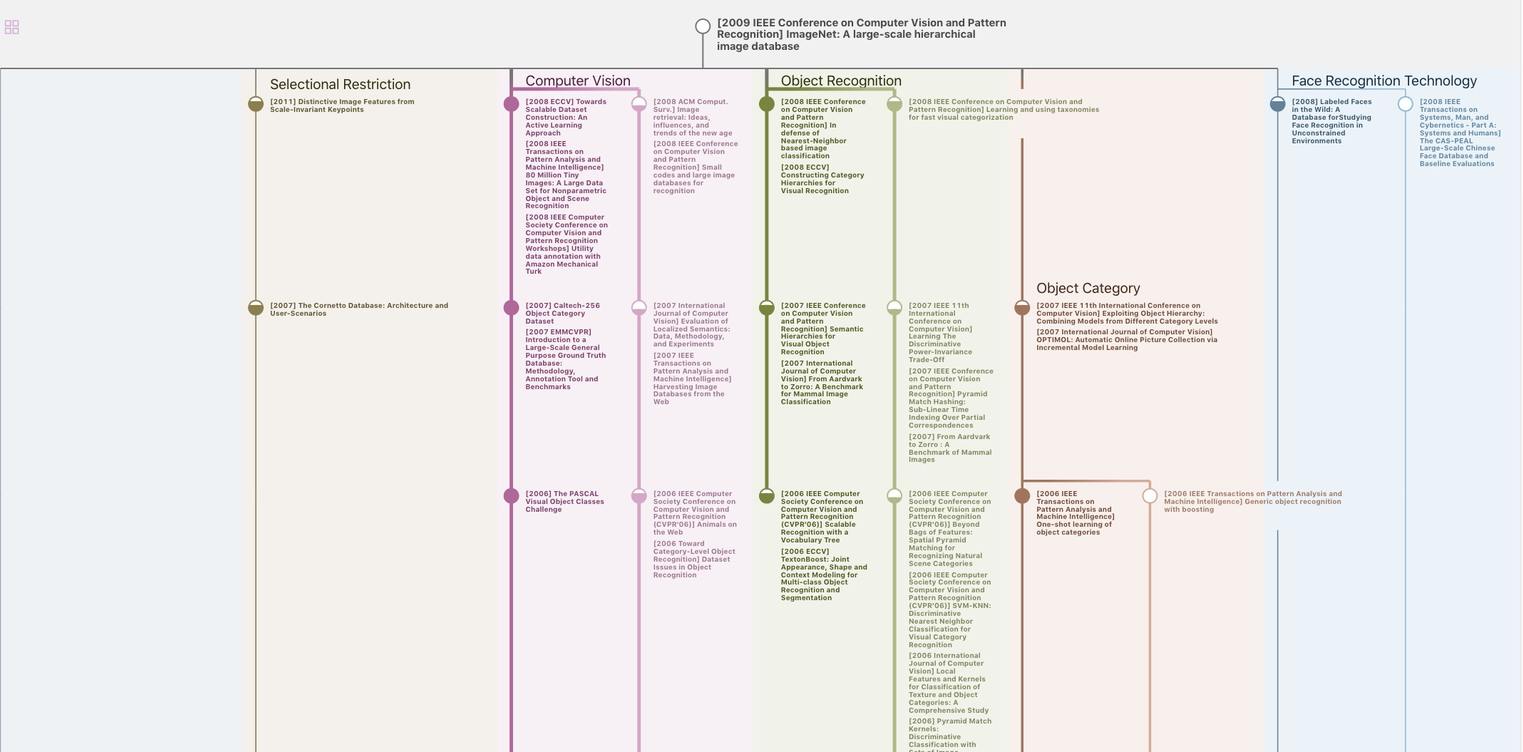
生成溯源树,研究论文发展脉络
Chat Paper
正在生成论文摘要