On Estimation of Modal Decompositions
ISIT(2020)
摘要
A modal decomposition is a useful tool that deconstructs the statistical dependence between two random variables by decomposing their joint distribution into orthogonal modes. Historically, modal decompositions have played important roles in statistics and information theory, e.g., in the study of maximal correlation. They are defined using the singular value decompositions of divergence transition matrices (DTMs) and conditional expectation operators corresponding to joint distributions. In this paper, we first characterize the set of all DTMs, and illustrate how the associated conditional expectation operators are the only weak contractions among a class of natural candidates. While modal decompositions have several modern machine learning applications, such as feature extraction from categorical data, the sample complexity of estimating them in such scenarios has not been analyzed. Hence, we also establish some non-asymptotic sample complexity results for the problem of estimating dominant modes of an unknown joint distribution from training data.
更多查看译文
关键词
associated conditional expectation operators,modal decomposition,unknown joint distribution,singular value decompositions,random variables,orthogonal modes,information theory,divergence transition matrices,joint distributions,DTM,machine learning applications,nonasymptotic sample complexity,training data
AI 理解论文
溯源树
样例
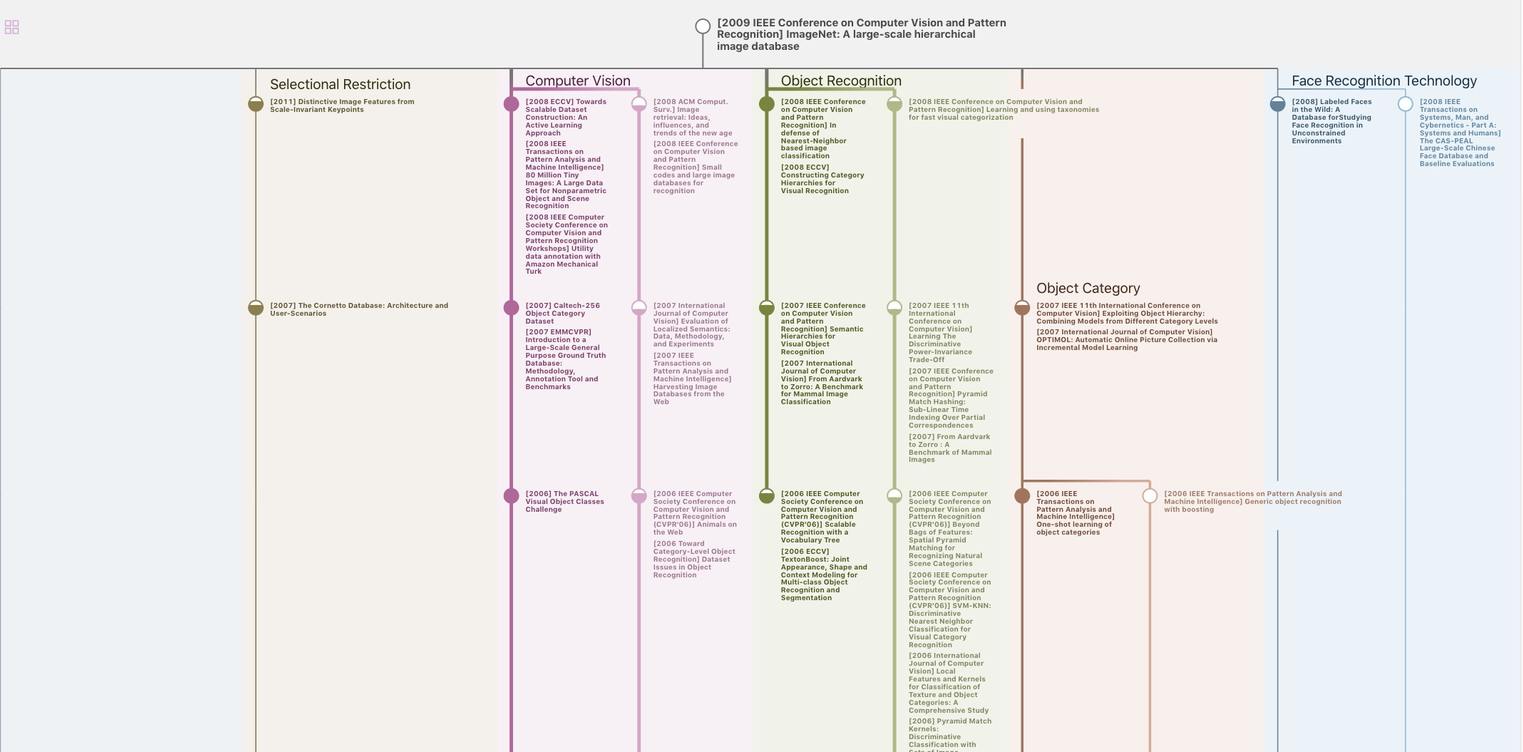
生成溯源树,研究论文发展脉络
Chat Paper
正在生成论文摘要