A Local Characterization for Wyner Common Information
ISIT(2020)
摘要
While the Hirschfeld-Gebelein-Renyi (HGR) maximal correlation and the Wyner common information share similar information processing purposes of extracting common knowledge structures between random variables, the relationships between these approaches are generally unclear. In this paper, we demonstrate such relationships by considering the Wyner common information in the weakly dependent regime, called ϵ-common information. We show that the HGR maximal correlation functions coincide with the relative likelihood functions of estimating the auxiliary random variables in ϵ-common information, which establishes the fundamental connections these approaches. Moreover, we extend the ϵ-common information to multiple random variables, and derive a novel algorithm for extracting feature functions of data variables regarding their common information. Our approach is validated by the MNIST problem, and can potentially be useful in multi-modal data analyses.
更多查看译文
关键词
local characterization,data variables,feature functions,MNIST problem,information processing,auxiliary random variables,HGR maximal correlation functions,ε-common information,common knowledge structure extraction,Wyner common information,Hirschfeld-Gebelein-Rényi maximal correlation,multiple random variables
AI 理解论文
溯源树
样例
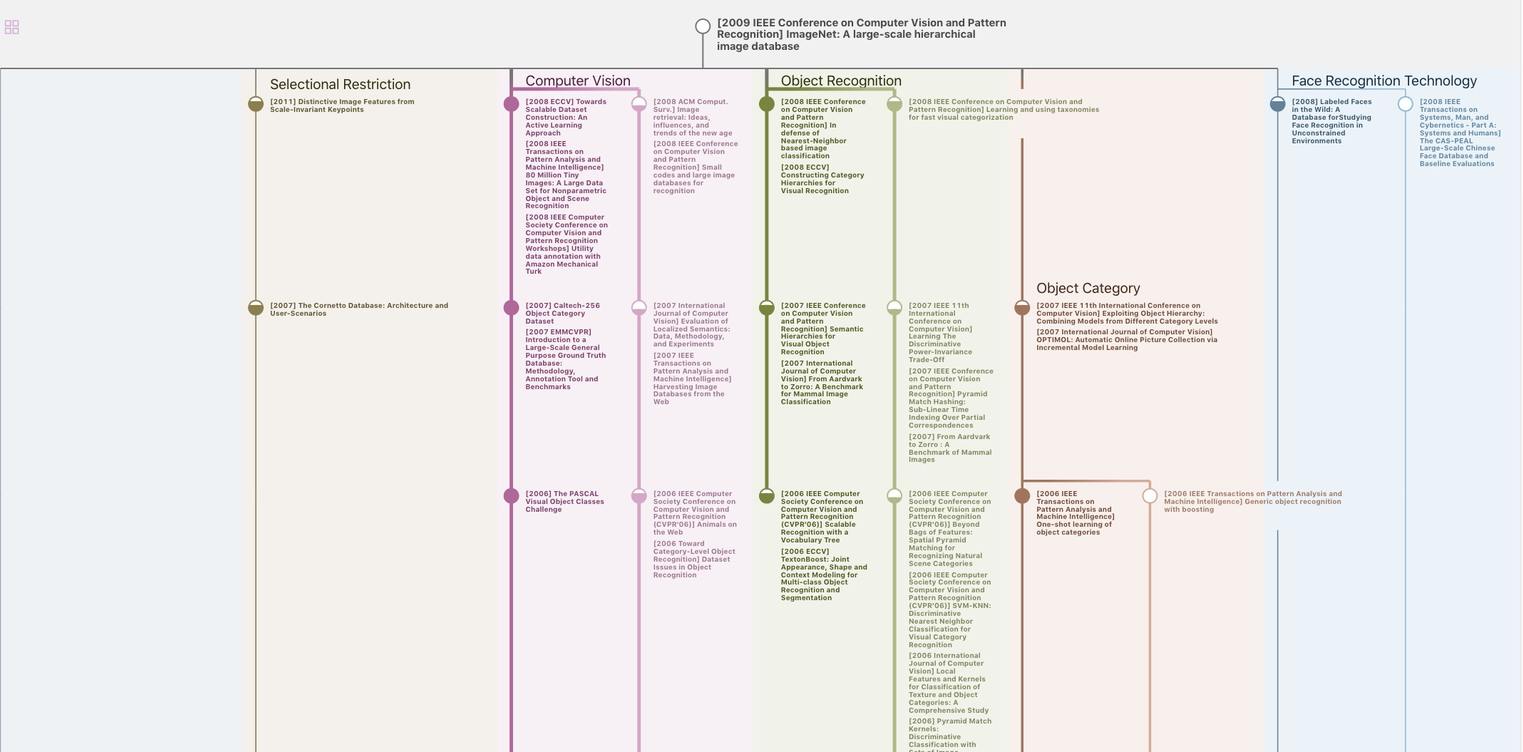
生成溯源树,研究论文发展脉络
Chat Paper
正在生成论文摘要