Deterministic And Probabilistic Wind Speed Forecasting With De-Noising-Reconstruction Strategy And Quantile Regression Based Algorithm
RENEWABLE ENERGY(2020)
摘要
Wind energy has become a kind of attractive alternative energy in power generation field due to its nonpolluting and renewable properties. Wind speed forecasting acts an important role in programming and operation of power systems. However, achieving high precision wind speed forecasts is still consider as an arduous and challenging issue with the randomization and transient exist in wind speed time series. For this reason, this paper proposed two novel de-noising-reconstruction-based hybrid models which consist of novel signal decomposed methods, feature selection approaches and predictors based on quantile regression and optimization algorithm to achieve more accurate short term wind speed forecasting. The developed hybrid models firstly eliminate inherent noise from the wind speed sequences via decomposed method and subsequently construct the appropriate datasets for the forecasting engines by adopting the feature selection method; finally, establish the predictors for the forecasting task. To verify the effectiveness of proposed forecasting models, 1-h and 2-h wind speed data collected from Yumen, Gansu province of China mainland is used as case studies. The computational results demonstrated that the developed hybrid models yield better performance contrast with those of other models involved in this research in terms of both wind speed deterministic and probabilistic forecasting. (c) 2020 Elsevier Ltd. All rights reserved.
更多查看译文
关键词
Renewable energy, Complete empirical mode decomposition with adaptive noise, Quantile regression neural network, Wind speed forecasting, Distance correlation
AI 理解论文
溯源树
样例
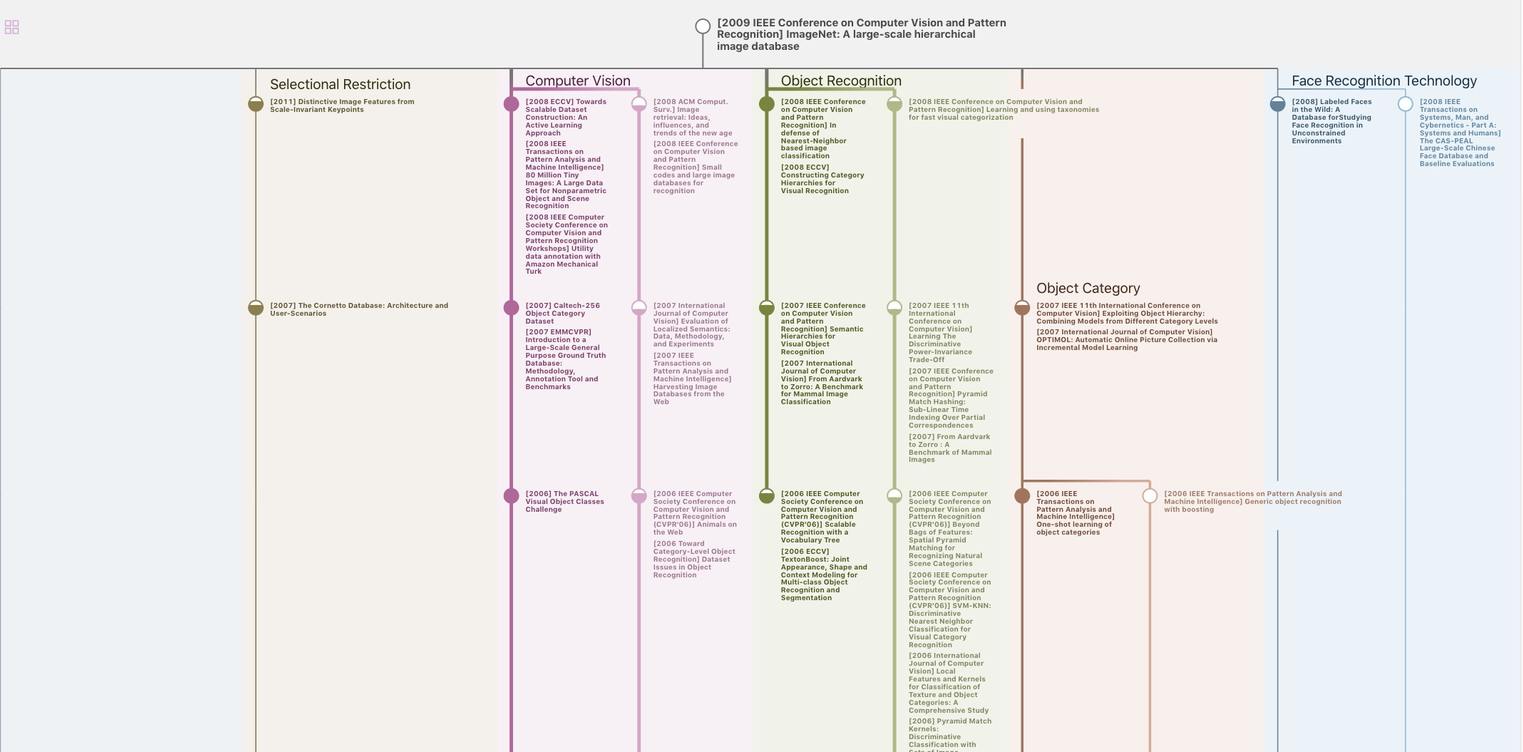
生成溯源树,研究论文发展脉络
Chat Paper
正在生成论文摘要