A Study of Population Diversity Using an Enhanced Brain Storm Optimization.
ICCAI(2020)
摘要
In evolutionary algorithm, population diversity is a vital factor for solving problems. To avoid the premature convergence, it is imperative to preserve the population diversity during the evolution. The population diversity ensures avoiding the premature convergence. Population diversity is a measure that has been used extensively in studies to measure premature convergence. Population diversity is convenient for measuring and dynamically adjusting an algorithm's ability of exploration or exploitation. Brain Storm Optimization (BSO) is a new kind of swarm intelligence method inspired by the cooperative behavior of human beings in the problem-solving process. BSO suffers the premature convergence which happens partly due to the solutions getting clustered. The solution set clustered after a few iterations which indicate that the diversity level decreases rapidly during the search. In order to enhance the computational efficacy of the original BSO algorithm and maintain the population diversity, we propose two ways to re-cluster the original BSO. We introduce a BSO in objective space with a Cauchy distribution with the knowledge that Cauchy distribution infers a faster rate. In addition, we introduce a new step size equation as a parameter to balance the exploration and exploitation in the search space. A good algorithm maintains population diversity in both spaces (objective and parameter spaces). Our goal is to investigate why the enhanced BSO performs efficiently from the perspective of population diversity on a set of five benchmark functions. Experimental figures show that the performance of the proposed algorithms performs better from the population diversity measurement.
更多查看译文
AI 理解论文
溯源树
样例
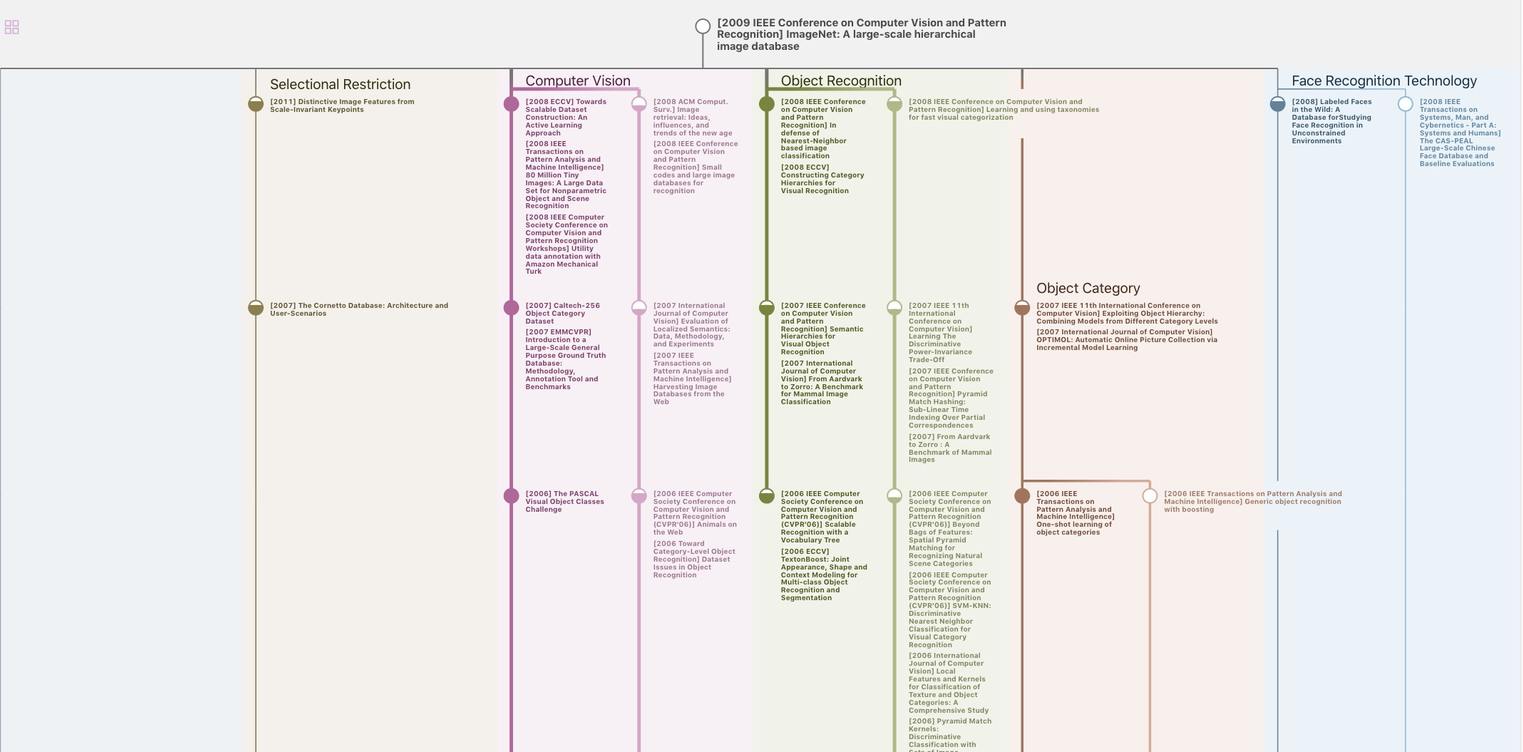
生成溯源树,研究论文发展脉络
Chat Paper
正在生成论文摘要