Using the rotation and random forest models of ensemble learning to predict landslide susceptibility
GEOMATICS NATURAL HAZARDS & RISK(2020)
摘要
Ensemble learning methods can be used to evaluate landslide susceptibility when combined with remote sensing (RS) and geographic information systems (GIS). In this study, the rotation forest (ROF) and random forest (RF) ensemble learning models were applied to evaluate landslide susceptibility. The experiments selected the factors by analysing the linear relationship between the factors, explored the optimal proportions of non-landslide samples and landslide samples based on an unbalanced sample dataset, and used the factors before and after the selection to generate landslide susceptibility maps (LSMs) in the Zigui-Badong area. The results show that a suitable ratio between the sample types in the training set can achieve good results for both sensitivity and specificity. The RF models of the study area with 21 factors and 16 factors had sensitivities of 94.22% and 93.59%, respectively. The ROF models with 21 factors and 16 factors had sensitivities of 90.63% and 88.84%, respectively. Although both the RF and ROF models exhibited high accuracy, the RF model achieved a more reasonable and accurate LSM.
更多查看译文
关键词
Landslide susceptibility,rotation forest,random forest,three gorges
AI 理解论文
溯源树
样例
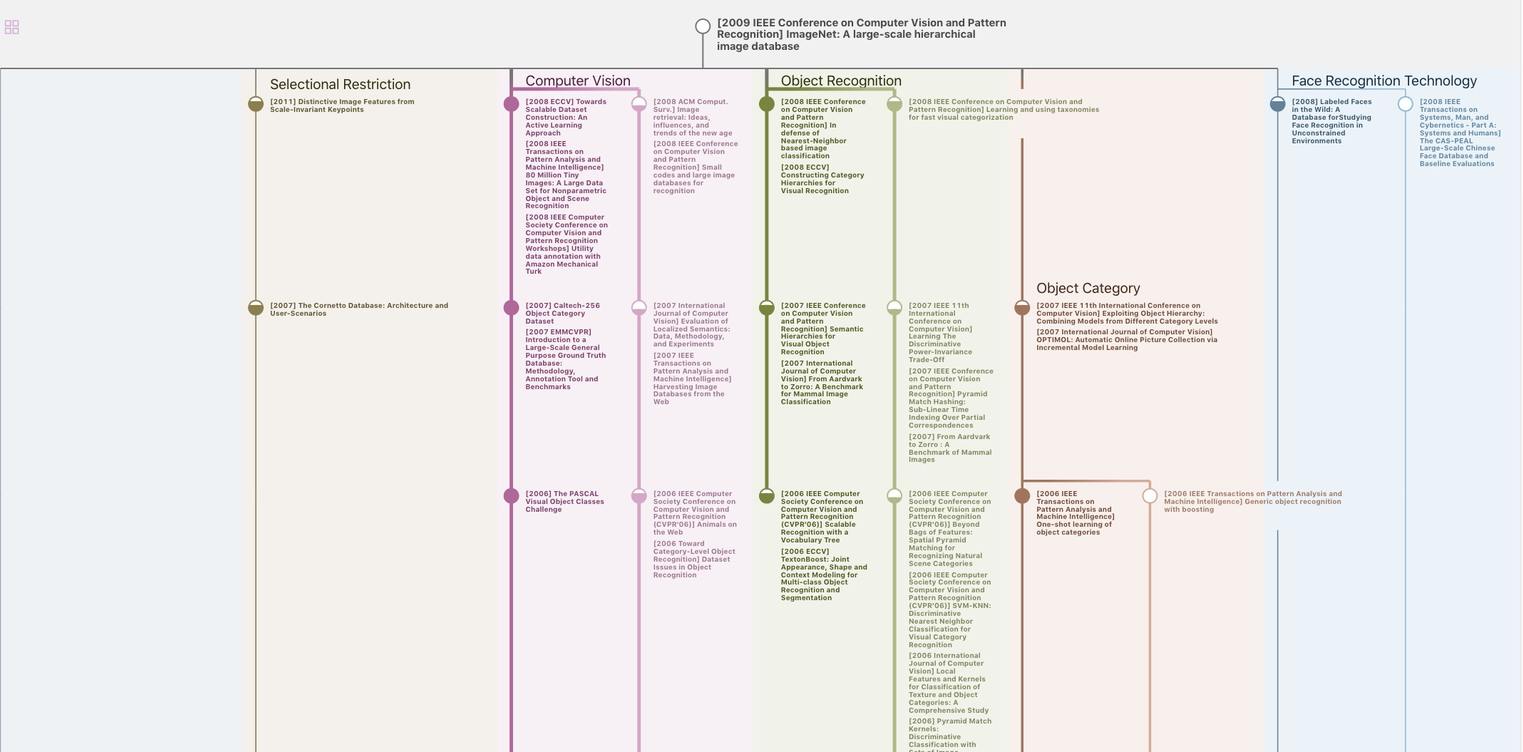
生成溯源树,研究论文发展脉络
Chat Paper
正在生成论文摘要