Towards a Non-Intrusive Context-Aware Speech Quality Model
2020 31st Irish Signals and Systems Conference (ISSC)(2020)
摘要
Understanding how humans judge perceived speech quality while interacting through Voice over Internet Protocol (VoIP) applications in real-time is essential to build a robust and accurate speech quality prediction model. Speech quality is degraded in the presence of background noise reducing the Quality of Experience (QoE). Speech Enhancement (SE) algorithms can improve speech quality in noisy environments. The publicly available NOIZEUS speech corpus contains speech in environmental background noise babble, car, street, and train at two Signal-to-noise ratio (SNRs) 5dB and 10dB. Objective Speech Quality Metrics (OSQM) are used to monitor and measure speech quality for VoIP applications. This paper proposes a Context-aware QoE prediction model, CAQoE, which classifies the speech signal context (i.e., noise type and SNR) in order to allow context-specific speech quality prediction. This paper presents experiments conducted to develop the speech context-classification component of the proposed CAQoE model. Speech enhancement algorithms are used in conjunction with an OSQM to estimate Mean Opinion Score (MOS) of noisy and enhanced samples in order to train Machine Learning (ML) classifiers to classify the speech signal context (i.e., noise type and SNR). Results demonstrate that a Decision Tree (DT) classifier has better classification accuracy for the noise classes tested. We present the associated components of the CAQoE model, namely; Voice Activity Detection (VAD) and Speech Quality Model (SQM).
更多查看译文
关键词
non-intrusive,speech quality,noise,speech enhancement,P.563,MOS,classifier,VAD,VoIP,QoE
AI 理解论文
溯源树
样例
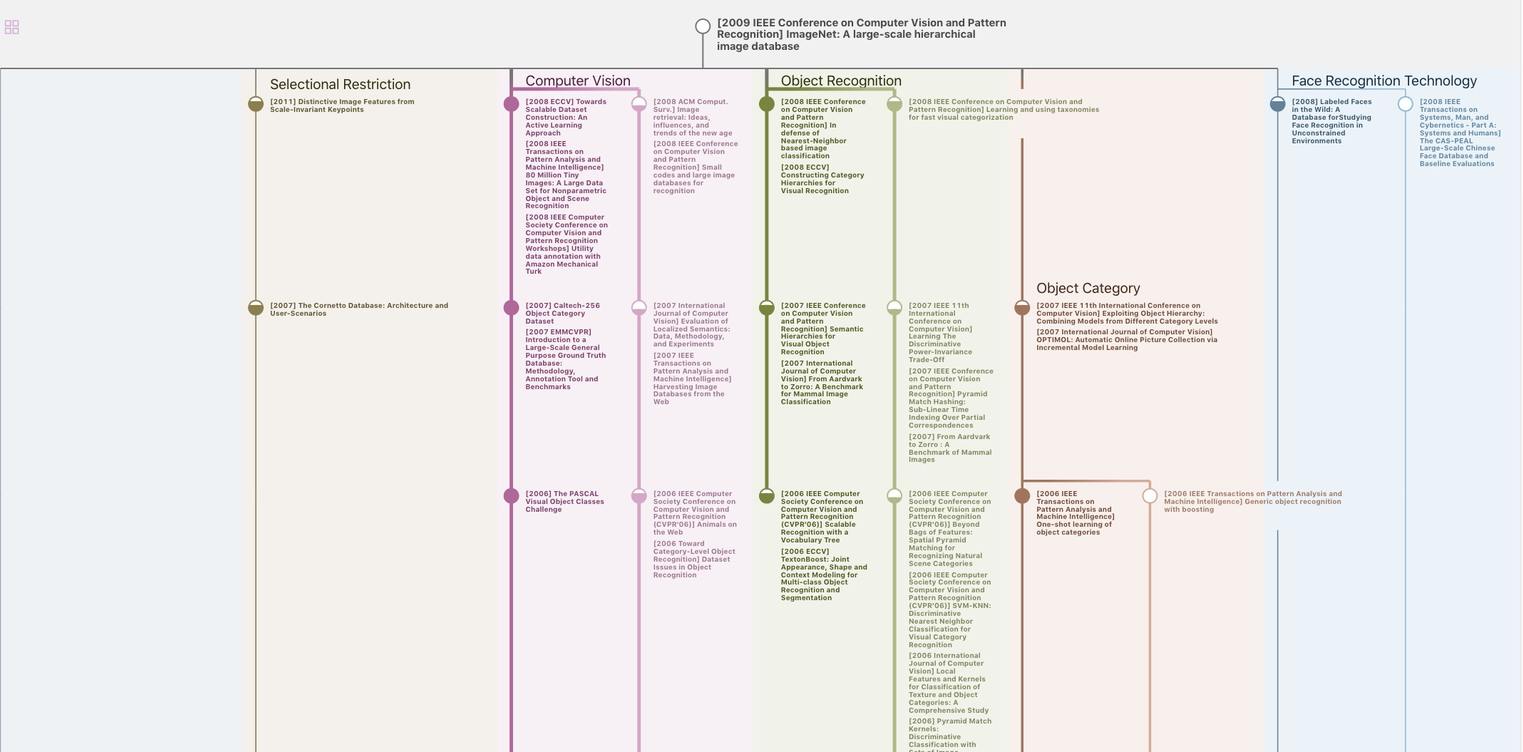
生成溯源树,研究论文发展脉络
Chat Paper
正在生成论文摘要