A multi-constraint failure-pursuing sampling method for reliability-based design optimization using adaptive Kriging
Engineering With Computers(2020)
摘要
Using surrogate models to substitute the computationally expensive limit state functions is a promising way to decrease the cost of implementing reliability-based design optimization (RBDO). To train the models efficiently, the active learning strategies have been intensively studied. However, the existing learning strategies either do not individually build the models according to importance measurement or do not completely relate to the reliability analysis results. Consequently, some points that are useless to refine the limit state functions or far away from the RBDO solutions are generated. This paper proposes a multi-constraint failure-pursuing sampling method to maximize the reward of adding new training points. A simultaneous learning strategy is employed to sequentially update the Kriging models with the points selected in the current approximate safe region. Moreover, the sensitive Kriging model as well as the sensitive sample point are identified based on the failure-pursuing scheme. A new point that is highly potential to improve the accuracy of reliability analysis and optimization can then be generated near the sensitive sample point and used to update the sensitive model. Besides, numerical examples and engineering application are used to validate the performance of the proposed method.
更多查看译文
关键词
Reliability-based design optimization, Adaptive Kriging modeling, Failure-pursuing sampling, Simultaneous learning
AI 理解论文
溯源树
样例
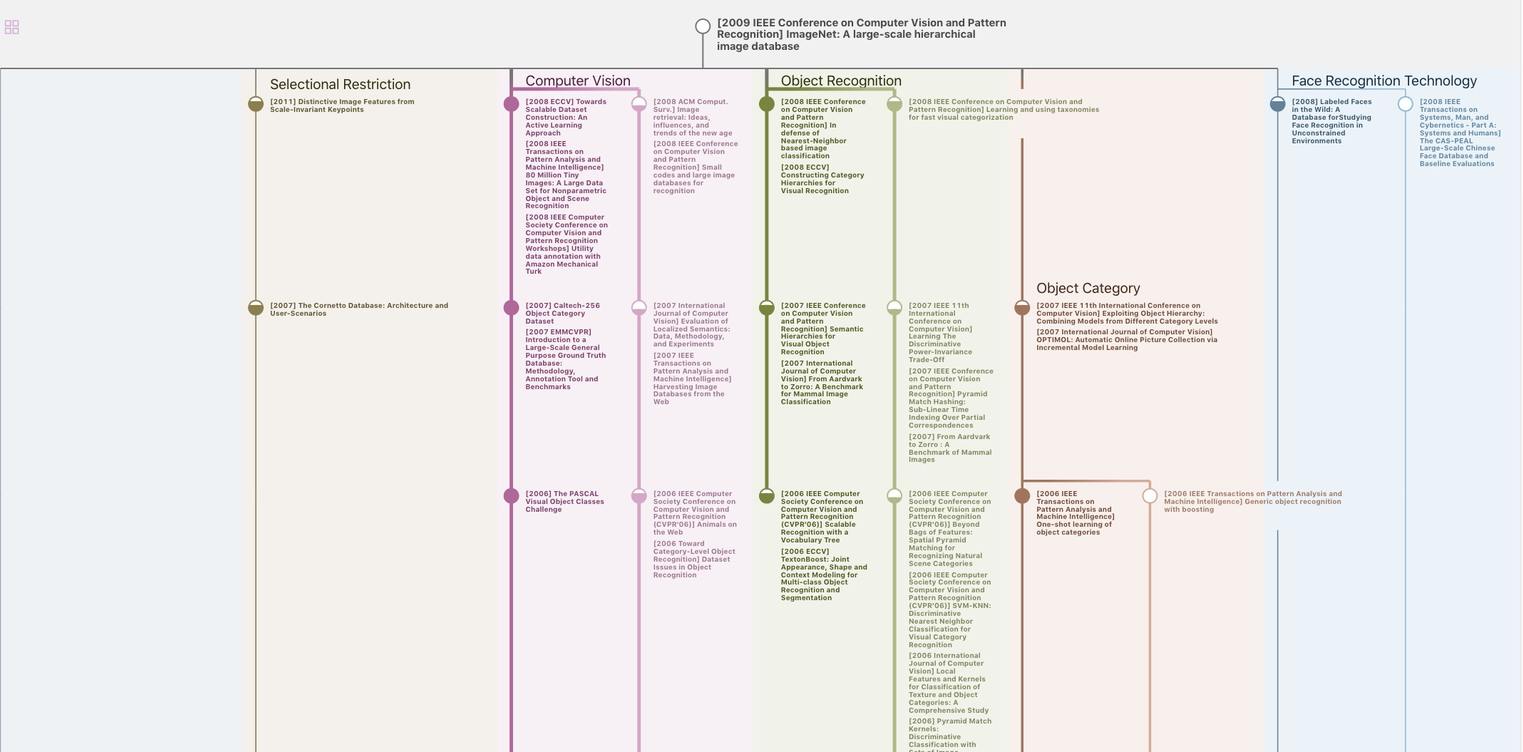
生成溯源树,研究论文发展脉络
Chat Paper
正在生成论文摘要