Possibilistic Clustering Enabled Neuro Fuzzy Logic
FUZZ-IEEE(2020)
摘要
Artificial neural networks are a dominant force in our modern era of data-driven artificial intelligence. The adaptive neuro fuzzy inference system (ANFIS) is a neural network based on fuzzy logic versus a more traditional premise like convolution. Advantages of ANFIS include the ability to encode and potentially understand machine learned neural information in the pursuit of explainable, interpretable, and ultimately trustworthy artificial intelligence. However, real-world data is almost always imperfect, e.g., incomplete or noisy, and ANFIS is not naturally robust. Specifically, ANFIS is susceptible to over inflated uncertainty, poor antecedent (fuzzy set) data alignment, degenerate optimization conditions, and hard to interpret logic, to name a few factors. Herein, we explore the use of possibilistic clustering to identify outliers, specifically typicality degrees, to increase the robustness of ANFIS; or any fuzzy logic neuron/network. Experiments are presented that demonstrate the need and quality of the proposed solutions in the pursuit of robust interpretable machine learned neuro fuzzy logic solutions.
更多查看译文
关键词
trustworthy artificial intelligence,ANFIS,data alignment,fuzzy set,possibilistic clustering,neuro fuzzy logic solutions,artificial neural networks,data-driven artificial intelligence,adaptive neuro fuzzy inference system,optimization,fuzzy logic neuron
AI 理解论文
溯源树
样例
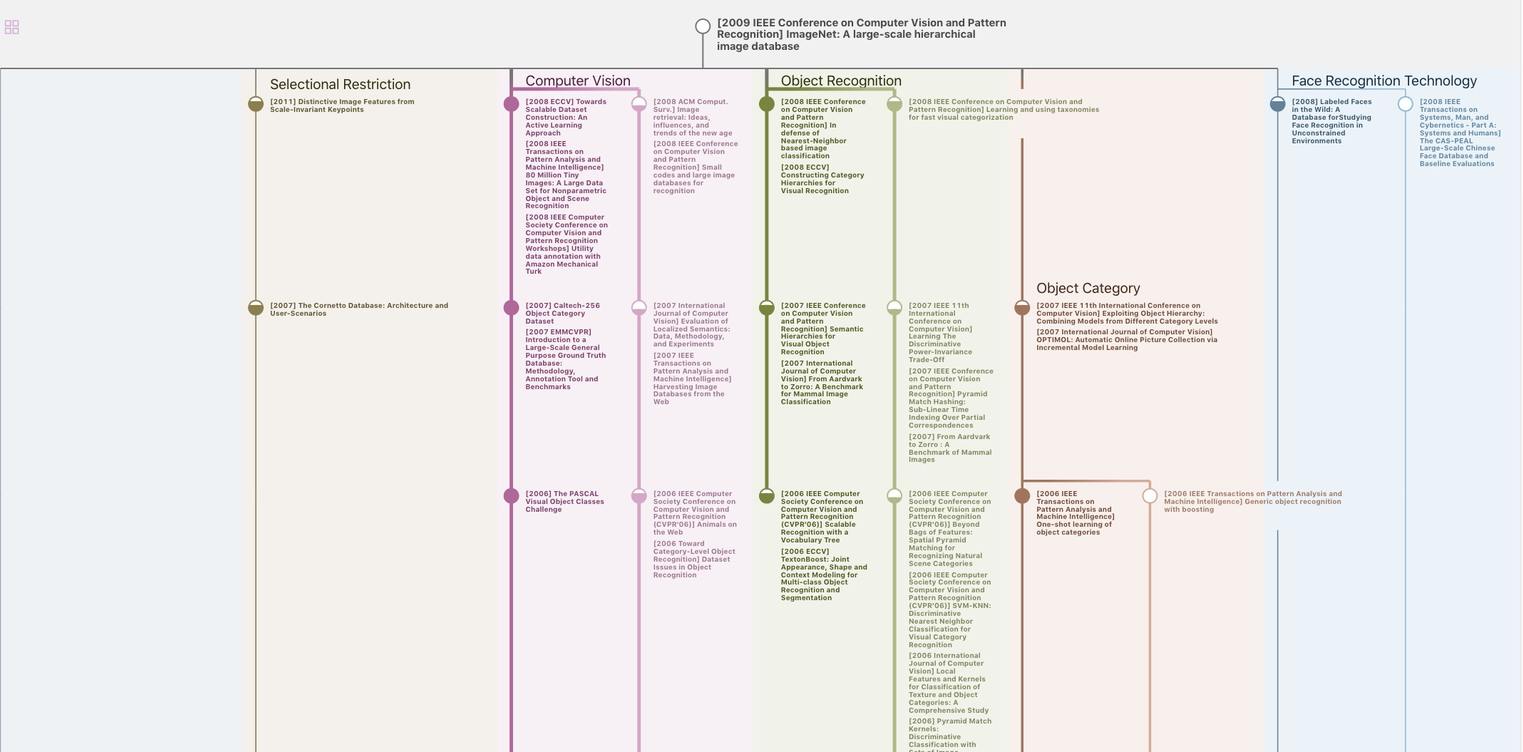
生成溯源树,研究论文发展脉络
Chat Paper
正在生成论文摘要