Two-branch encoding and iterative attention decoding network for semantic segmentation
NEURAL COMPUTING & APPLICATIONS(2020)
摘要
Deep convolutional neural networks(DCNNs) have shown outstanding performance in semantic image segmentation. In this paper, we propose a two-branch encoding and iterative attention decoding semantic segmentation model. In encoding stage, an improved PeleeNet is used as the backbone branch to extract dense image features, and the spatial branch is used to preserve fine-grained information. In decoding stage, the iterative attention decoding is employed to optimize the segmentation results with multi-scale features. Furthermore, we propose a channel position attention module and a boundary residual attention module to learn different position and boundary features, which can enrich the target boundary position information. Finally, we use SegNet as the basic network and conduct some experiments to evaluate the effect of each component in the proposed model with accuracy and mIOU on CamVid dataset. Furthermore, we verify the segmentation performance of the proposed model with comparable experiments on CamVid, Cityscapes and PASCAL VOC 2012 dataset. In particular, the model has achieved 91.7% segmentation accuracy and 67.1% mIOU on the CamVid dataset respectively, which verify the effectiveness of our proposed model. In the future, we can combine target detection with semantic segmentation to further improve the semantic segmentation effect of small objects. We also hope to further optimize the model structure and reduce its time complexities and parameters under the guarantee of effectiveness.
更多查看译文
关键词
Semantic segmentation,Two-branch encoding,Improved PeleeNet,Iterative attention decoding,Channel position attention,Boundary residual attention
AI 理解论文
溯源树
样例
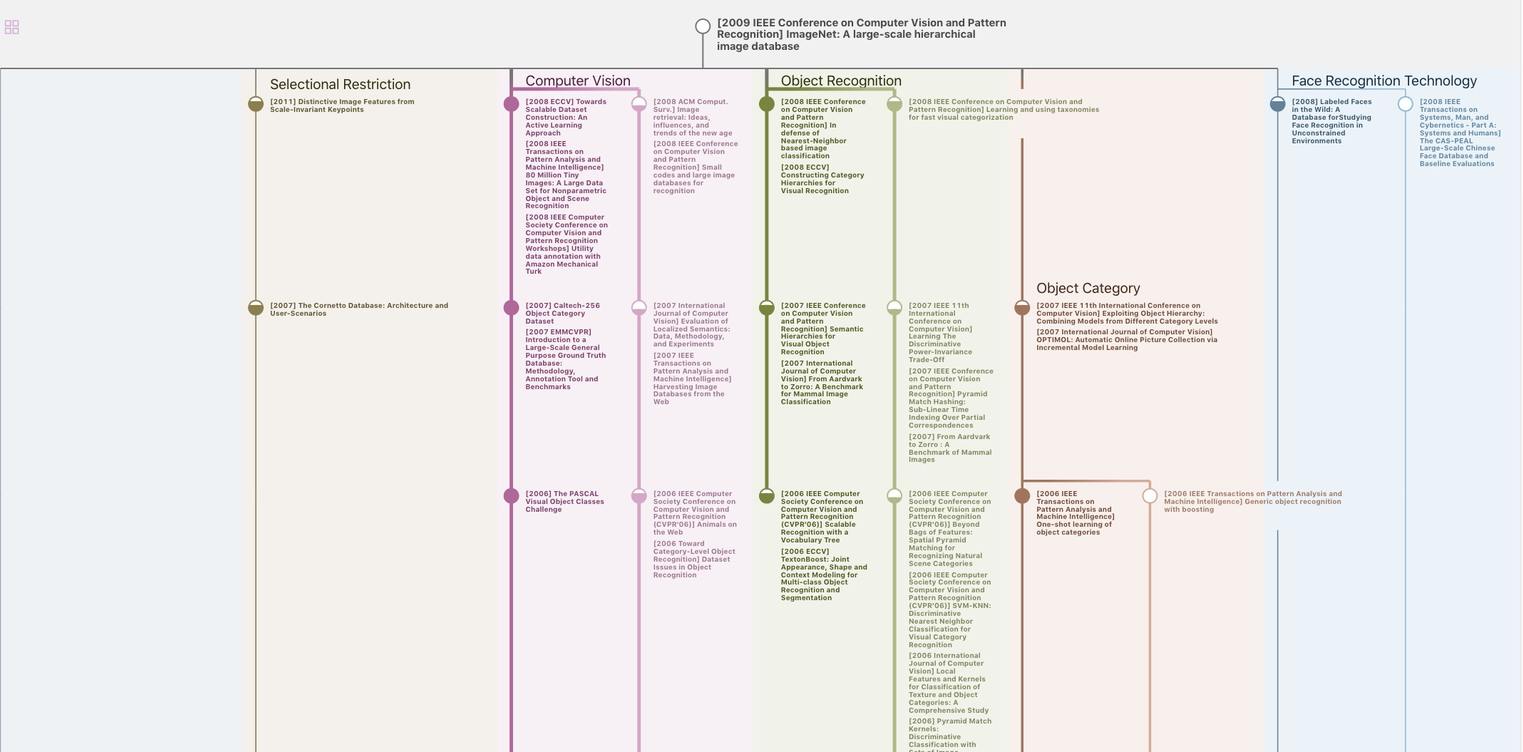
生成溯源树,研究论文发展脉络
Chat Paper
正在生成论文摘要