Framework for Mining Hybrid Automata from a Constrained Machine Learning Architecture
2020 IEEE International Conference on Fuzzy Systems (FUZZ-IEEE)(2020)
摘要
As learning enabled cyber physical systems become more prolific, modeling for the purposes of verification and validation (V&V) becomes a primary barrier. A key challenge is to model cyber physical systems at the appropriate level of abstraction while maintaining a clear and understandable linkage between the human designer and the system under design. Fuzzy logic is a framework to synthesize linguistic, natural language requirements into machine learning models. It has been shown that fuzzy logic is a suitable method to learn the behavior of a nonlinear system when a model is not present. The implementation of a constrained fuzzy inference system presented in this paper is a specific class of machine learning architecture that provides a mechanism to relate a system model to human specified requirements. It is not at all dissimilar to more popular neural network architectures. The goal of this paper is to introduce a framework for constrained learning that enables both fast approximation of real valued systems while simultaneously enabling the modeling and analysis of safety properties. This paper focuses on a foundational constrained intelligent learning framework. The framework describes an alternative and constrained machine learning architecture capable of producing highly efficient structures that are also amenable to verification. This paper will focus on the efficiency of the computational structure while future papers will demonstrate the verifiability.
更多查看译文
关键词
fuzzy logic,nonlinear system,constrained fuzzy inference system,neural network architectures,constrained learning,hybrid automata,cyber physical systems,natural language requirements,mining,constrained machine learning architecture,verification and validation,linguistic requirements,safety properties
AI 理解论文
溯源树
样例
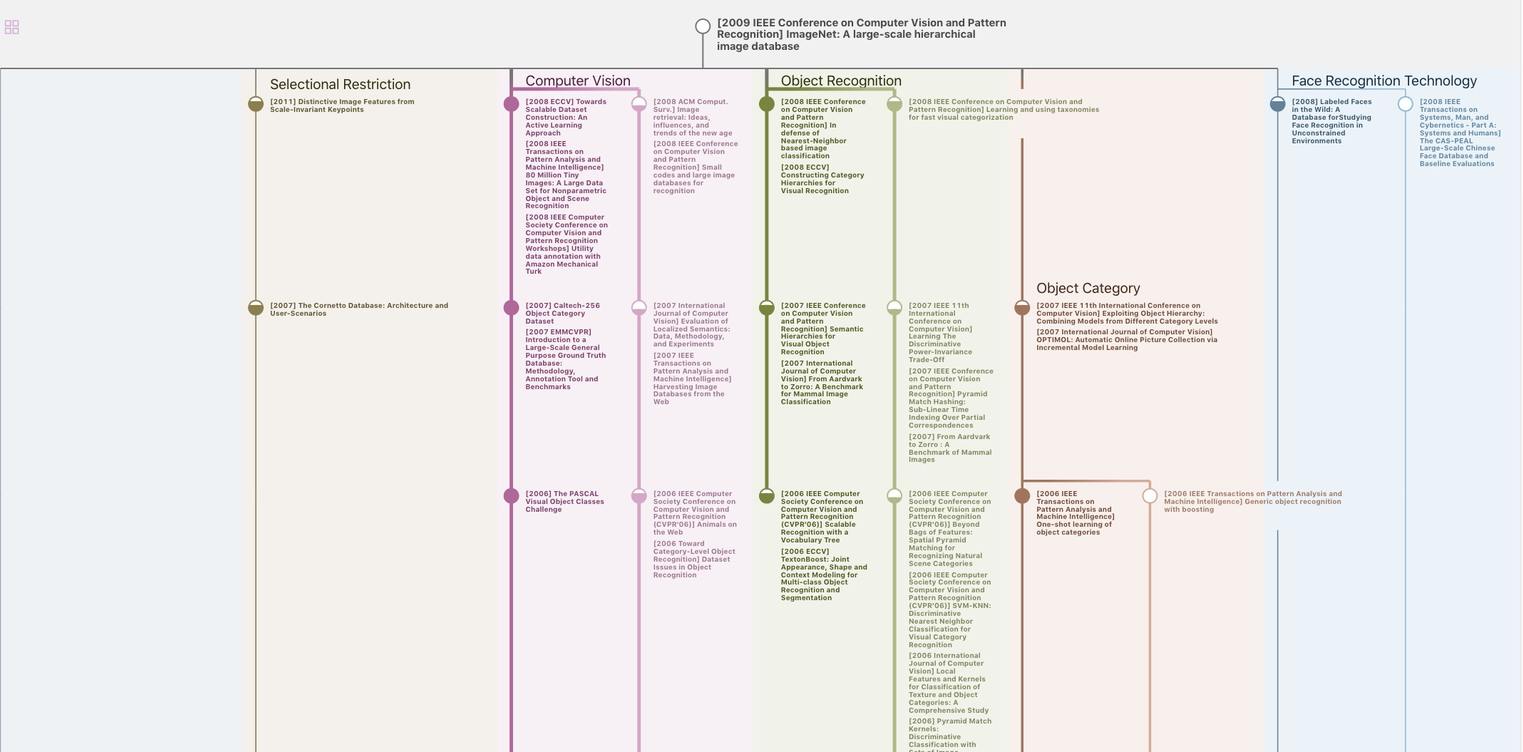
生成溯源树,研究论文发展脉络
Chat Paper
正在生成论文摘要