Gathering Effective Information for Real-Time Material Recognition
IEEE ACCESS(2020)
摘要
Material recognition is a fundamental problem in the field of computer vision. Material recognition is still challenging because of varying camera perspectives, light conditions, and illuminations. Feature learning or feature engineering helps build an important foundation for effective material recognition. Most traditional and deep learning-based features usually point to the same or similar material semantics from diverse visual perspectives, indicating the implicit complementary information (or cross-modal semantics) among these heterogeneous features. However, only a few studies focus on mining the cross-modal semantics among heterogeneous image features, which can be used to boost the final recognition performance. To address this issue, we first improve the well-known multiset discriminant correlation analysis model to fully mine the cross-modal semantics among heterogeneous image features. Then, we propose a novel hierarchical multi-feature fusion (HMF2) model to gather effective information and create novel yet more effective and robust features. Finally, a general classifier is employed to train a new material recognition model. Experimental results demonstrate the simplicity, effectiveness, robustness, and efficiency of the HMF2 model on two benchmark datasets. Furthermore, based on the HMF2 model, we design an end-to-end online system for real-time material recognition.
更多查看译文
关键词
Semantics,Image recognition,Correlation,Robustness,Benchmark testing,Visualization,Analytical models,Material recognition,hierarchical multi-feature fusion,discriminant correlation analysis,cross-modal semantics
AI 理解论文
溯源树
样例
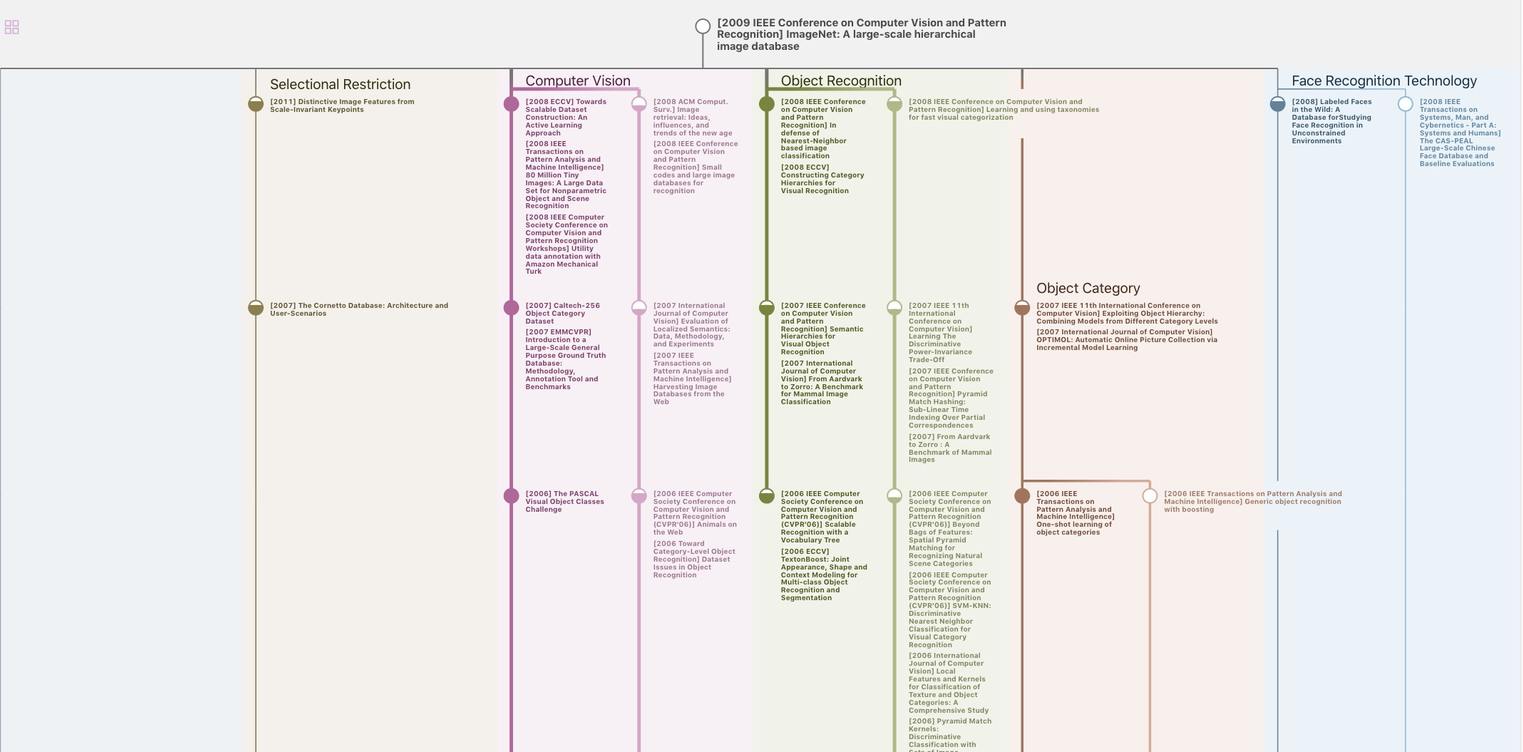
生成溯源树,研究论文发展脉络
Chat Paper
正在生成论文摘要