Age-Related Differences In Healthy Adults Walking Patterns Under A Cognitive Task With Deep Neural Networks
IEEE SENSORS JOURNAL(2021)
摘要
We investigated cognitively demanding tasks on patterns of human gait in healthy adults with a deep learning methodology that learns from raw gait data. Age-related differences were analyzed in dual-tasks in a cohort of 69 cognitively healthy adults organized in stratified groups by age. A novel spatio-temporal deep learning methodology was introduced to effectively classify dual-tasks from spatio-temporal raw gait data, obtained from a unique tomography floor sensor. The approach outperformed traditional machine learning approaches. The most favorable classification F-score obtained was of 97.3% in dual-tasks in a young age group experiment for a total of 12 users. The deep machine learning methodology outperformed classical machine learning methodologies by 63.5% in the most favorable case. Finally, a 2D manifold representation was obtained from trained deep learning models' data, to visualize and identify clusters from features learned by the deep learning models. This study demonstrates a novel approach to dual-task research by proposing a data-driven learning methodology with stratified age-groups.
更多查看译文
关键词
Task analysis, Machine learning, Legged locomotion, Sensor systems, Data models, Databases, Deep learning, dual task, floor sensor system, age-related differences
AI 理解论文
溯源树
样例
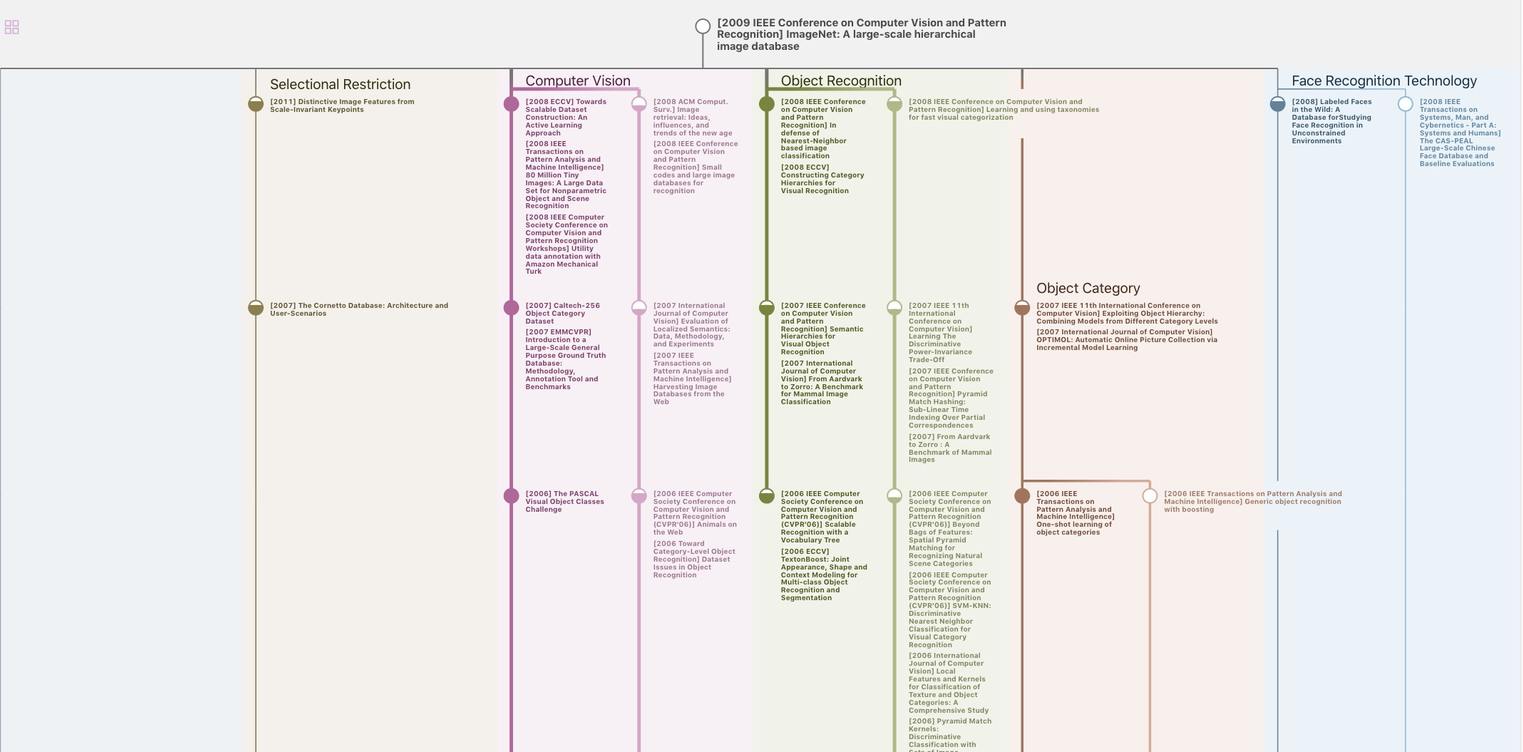
生成溯源树,研究论文发展脉络
Chat Paper
正在生成论文摘要