Feature selection for improved classification accuracy targeting riverine sand mapping
SPATIAL INFORMATION RESEARCH(2020)
摘要
Regular monitoring of riverine sand is crucial for its sustainable management. Towards readily generating spatial information regarding the extent of riverine sand, this study utilizes satellite-based remote sensing data as input features while applying Support Vector Machine (SVM) classifier to discern the inherent land cover classes. The input features comprised of spectral bands, derived spectral indices, derived textural features and ancillary information such as elevation, slope and aspect. The objective of this study was to identify a set of features that help improve the SVM classification accuracy with respect to the benchmark accuracy achieved by using only the spectral bands. Apart from testing a few commonly used logical combinations of features, the study focused on employing the Correlation-based Feature Selection (CFS) method along with the best-first search algorithm to generate a feature-set that comprised of most relevant and least correlated features. This feature-set was found to improve the SVM classification accuracy by 2.9% with respect to the benchmark value. Further, when tested with the limited number of training samples, similar results were achieved. This study proves that it is beneficial to utilize the CFS method for feature selection prior to SVM classification and recommends a set of 19 remote sensing derived features that are relevant towards riverine sand mapping.
更多查看译文
关键词
Riverine sand mapping, Sentinel-2, SVM, Correlation-based feature selection (CFS), Google Earth Engine (GEE), Waikato environment for knowledge analysis (WEKA)
AI 理解论文
溯源树
样例
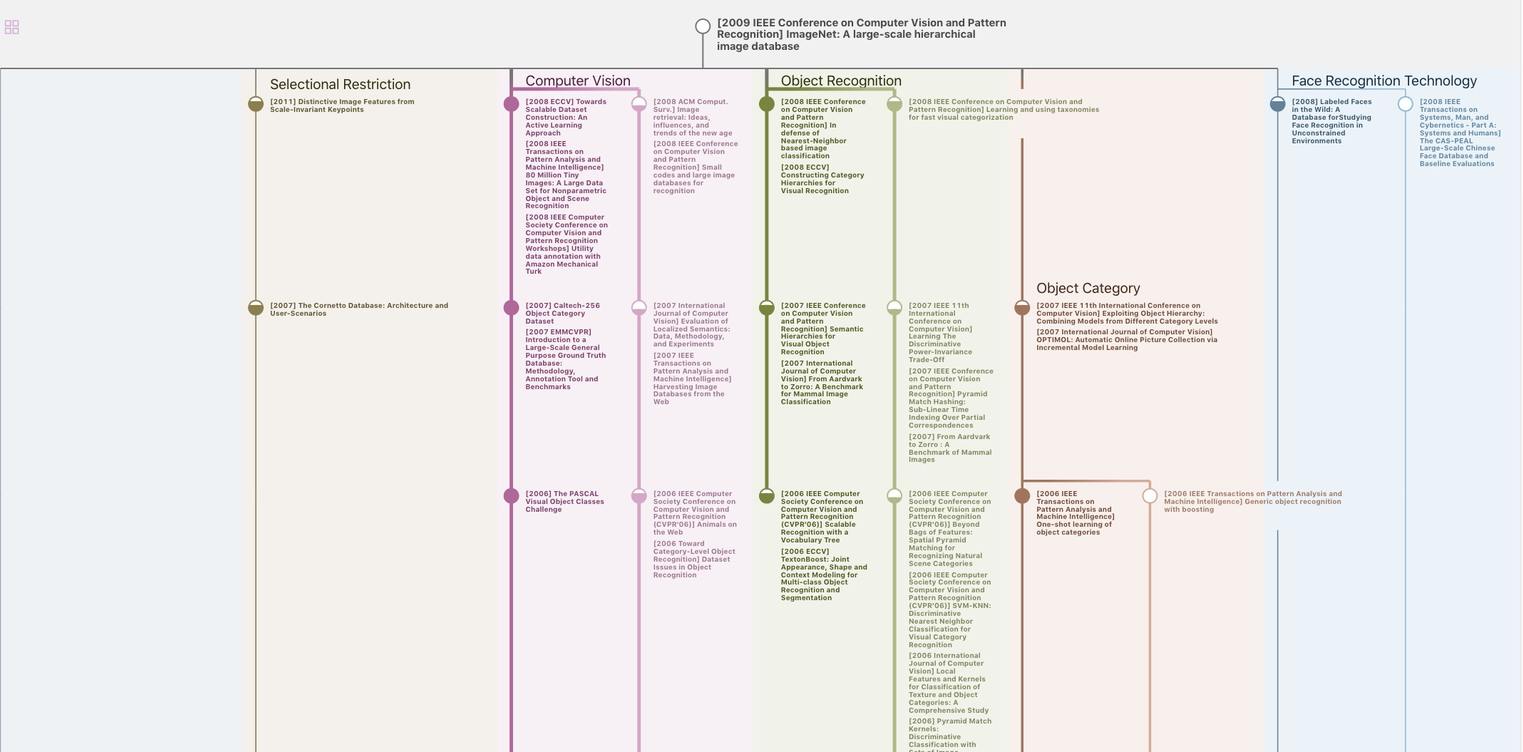
生成溯源树,研究论文发展脉络
Chat Paper
正在生成论文摘要