Multi-Dimensional Prediction Model For Cell Traffic In City Scale
INTERNATIONAL JOURNAL OF PATTERN RECOGNITION AND ARTIFICIAL INTELLIGENCE(2021)
摘要
Traffic prediction is a classical time series prediction which has been investigated in different domains, but most existing models are proposed based on limited time or spatial scale. Mobile cellular network traffic prediction is of paramount importance for quality-of-service (QoS) and power management of the cellular base stations, especially in the 5G era. Through the statistical analysis of the real historical traffic data obtained in a city scale spanning across multiple months, this paper makes an in-depth study of the temporal characteristics and behavior rules of the model data traffic. Considering that the time series data show different changing rules under the different time dimensions, spatial dimensions and independent dimensions, a multi-dimensional recurrent neural network (MDRNN) prediction model is established to predict the future cell traffic volume over various temporal and spatial dimensions. The data of this paper are trained and tested over real data of a city, and the granularity of the proposed prediction model can be drilled down to the cell level. Compared with the traditional trend fitting method, the proposed model achieves mean absolute percentage error (MAPE) reduction of 6.56%, and provides guidance for energy efficiency optimization and power consumption reduction of base stations in various temporal and spatial dimensions.
更多查看译文
关键词
Cell traffic, Multi-dimension, prediction model, MDRNN
AI 理解论文
溯源树
样例
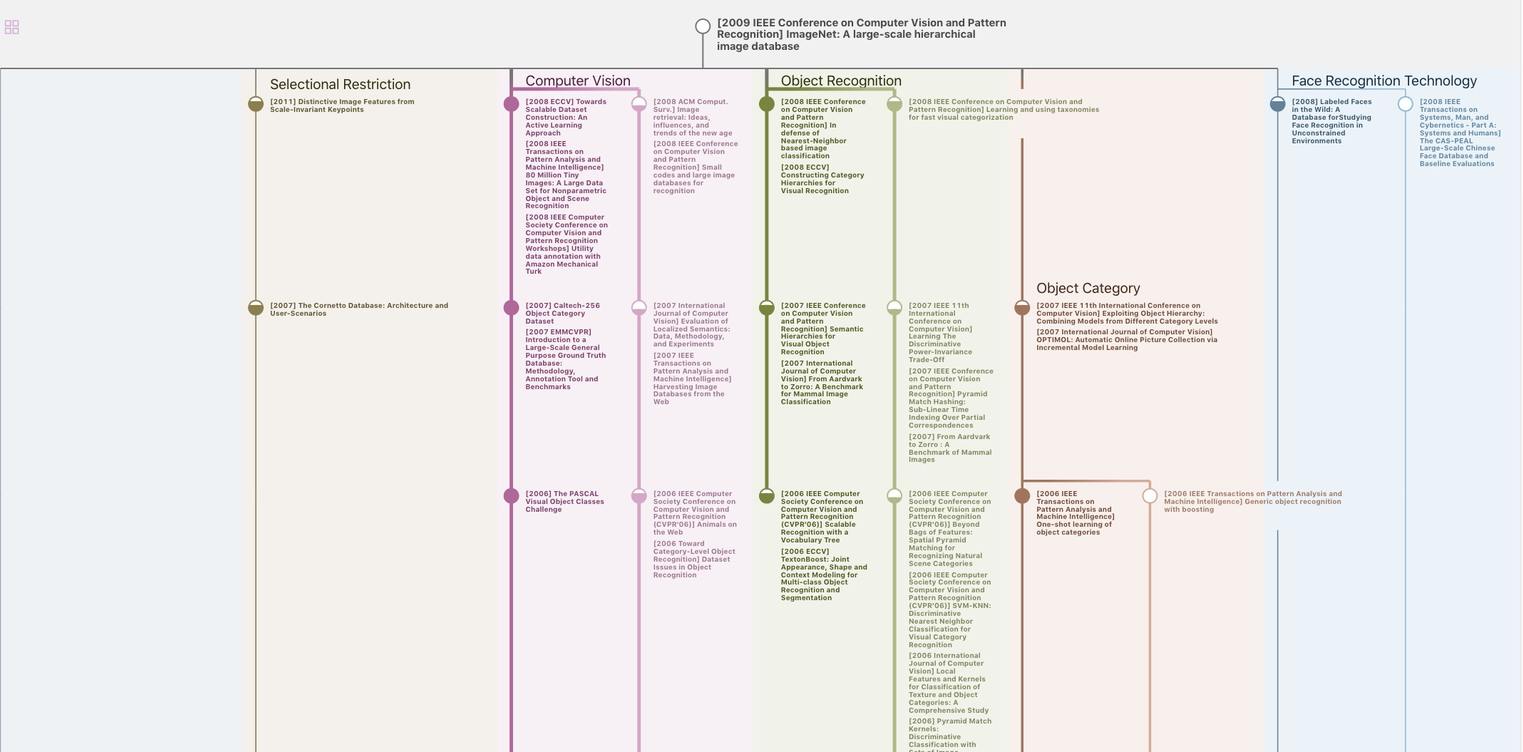
生成溯源树,研究论文发展脉络
Chat Paper
正在生成论文摘要