Sub-Daily Soil Moisture Estimate Using Dynamic Bayesian Model Averaging
JOURNAL OF HYDROLOGY(2020)
摘要
Accurate estimation of soil moisture (SM) from satellite products and model simulations at sub-daily timescale remains a challenge. This study proposes a general dynamic Bayesian model averaging (BMA) framework for merging sub-daily model products. Compared to the traditional BMA method, this study introduces adaptive weights (dynamically variant with time) for BMA members. Based on the previous evaluation work, a subset of model products is selected from eight model products as BMA members. The dynamic BMA experiment is performed for the surface SM (0-10 cm) model products at sub-daily (6-h) timescale in 2017 over the Yangtze-Huaihe river basin. The results are compared with the automatic SM observations (ASMOs) with unprecedented high spatial and temporal resolution (up to 7 stations within a 104 km(2) pixel; hourly). Because weather pattern and model performance change over time, the determination of an optimal training period is critical to obtain adaptive BMA weights for rapid weather regime changes. The sensitivity of training length (days) is then examined, and the optimum data length used in the BMA training period proves to be about 80 days. With deterministic and probabilistic verification metrics, the dynamic BMA estimated SM is comprehensively evaluated against the ASMOs, eight global model products, and the CMA's (China Meteorological Administration) regional Land Data Assimilation System (CLDAS) product. To better compare the probability distribution of different products, the cumulative distribution function (CDF) consistency histogram and a more objective metric consistency deviation (CD) are proposed to diagnose the consistency of two SM CDFs (e.g., the BMA estimated and the observed CDF). In terms of both the deterministic (the Kling-Gupta efficiency, correlation, system bias, and bias adjusted root-mean square error) and probabilistic verification methods (CD, QQ-plots, and reliability), the dynamic BMA estimated SM outperforms any BMA members and even the benchmark product CLDAS. This study demonstrates that the dynamic BMA framework provides a new solution for merging SM model products. The merged SM and the BMA combined probability distribution can be further used for drought monitoring and prediction.
更多查看译文
关键词
Soil moisture, Dynamic Bayesian model averaging, Reanalysis, Land data assimilation system, CLDAS
AI 理解论文
溯源树
样例
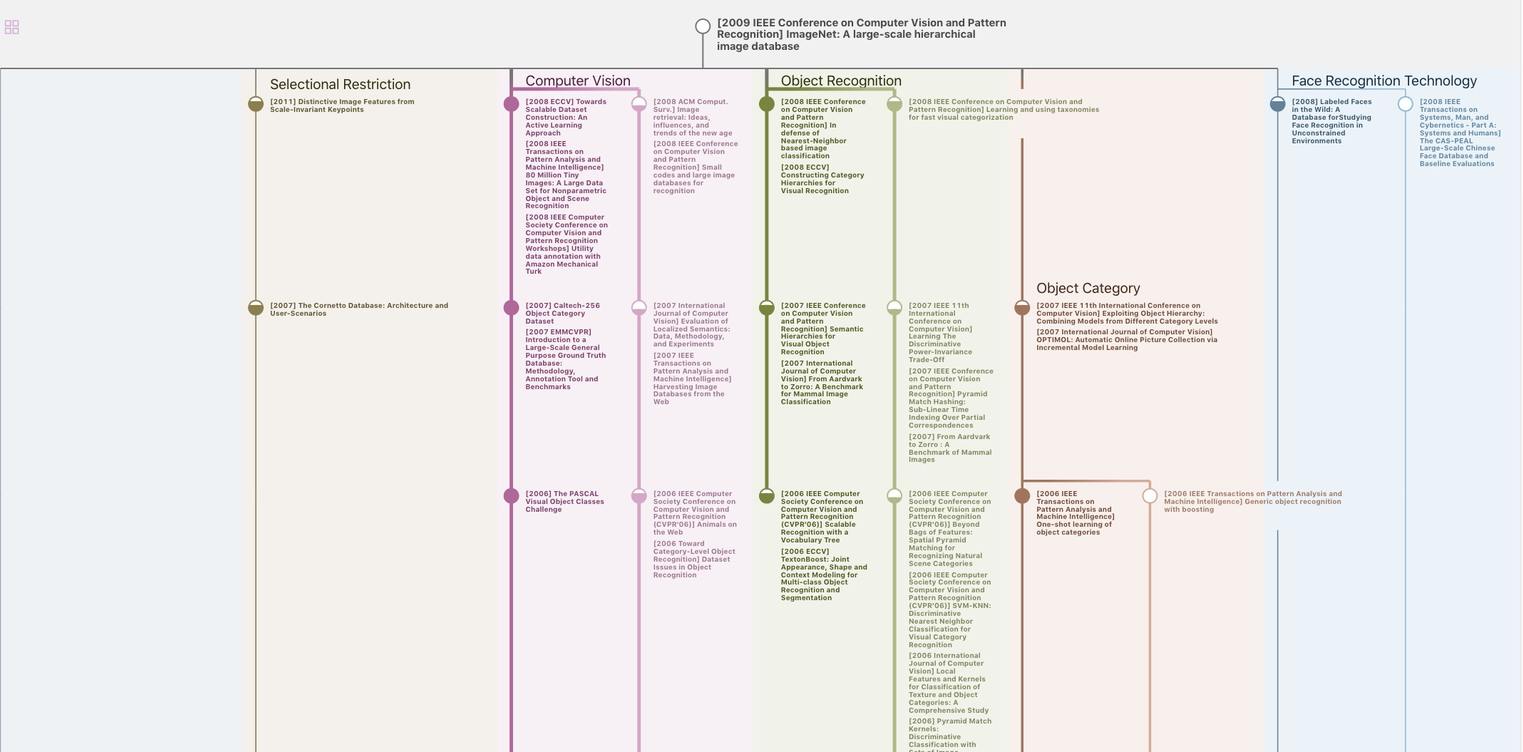
生成溯源树,研究论文发展脉络
Chat Paper
正在生成论文摘要