A Framework for Automatic Calibration of SWMM Considering Input Uncertainty
Hydrology and Earth System Sciences Discussions(2020)
摘要
Abstract. A new Bayesian framework for automatic calibration of SWMM, which simultaneously considers both parameter uncertainty and input uncertainty, was developed. The framework coupled an optimization algorithm DREAM and the Storm Water Management Model (SWMM) by newly developed API functions used to obtain and adjust parameter values of the model. Besides, a rainfall error model was integrated into the framework to consider systematic rainfall errors. A case study in Guangzhou, China was conducted to demonstrate the use of the framework. The calibration capability of the framework was tested and the impacts of rainfall uncertainty on model parameter estimations and simulated runoff boundaries were identified in the study area. the results show that calibration considering both parameter uncertainty and rainfall uncertainty captures peak flow much better and is more robust in terms of the Nash Sutcliffe index than that only considering parameter uncertainty.
更多查看译文
AI 理解论文
溯源树
样例
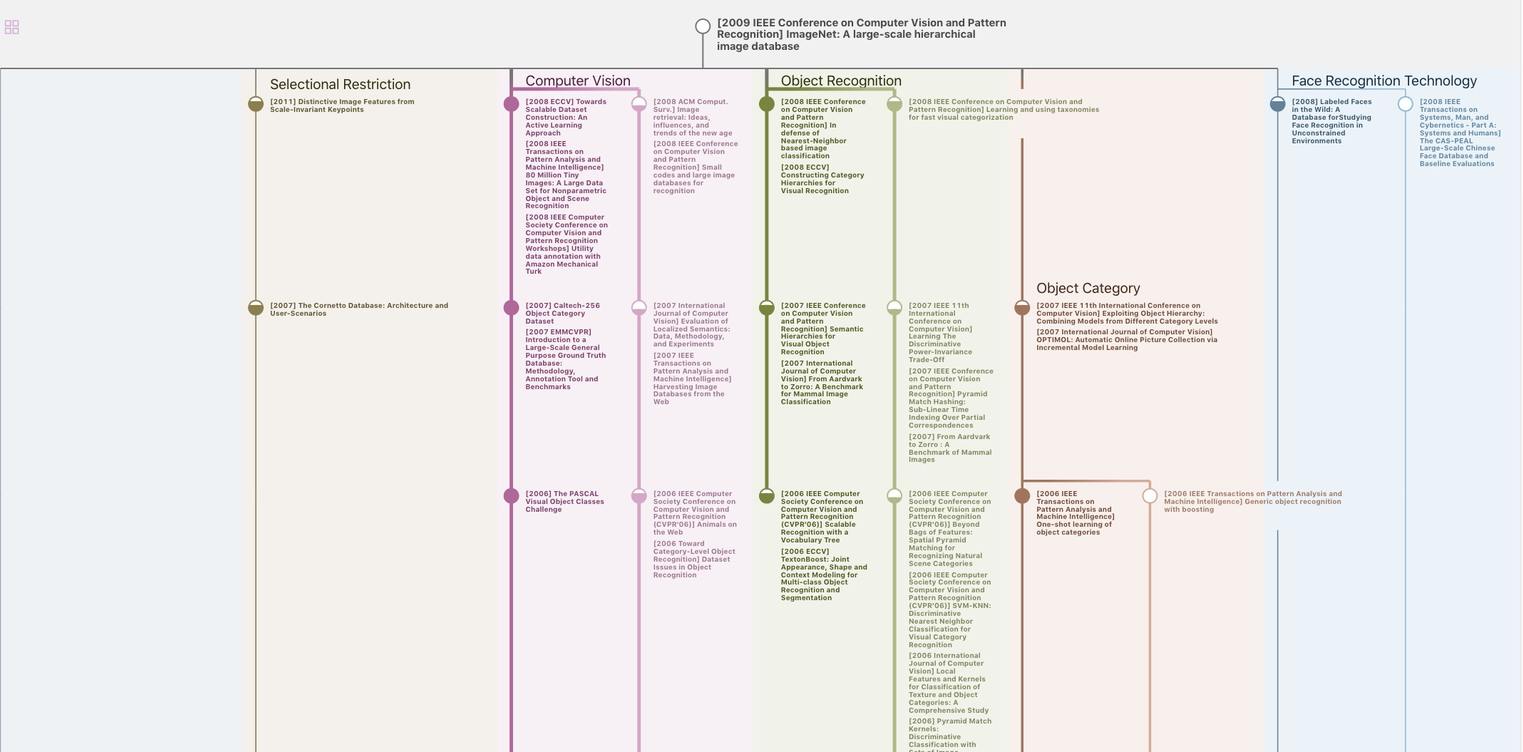
生成溯源树,研究论文发展脉络
Chat Paper
正在生成论文摘要