Thermal Imaging Combined with Predictive Machine Learning Based Model for the Development of Thermal Stress Level Classifiers
Livestock science(2020)
摘要
Thermal stress in dairy cows has been studied to improve production efficiency and animal welfare. Several authors have verified the potential of infrared thermography (IRT) as noninvasive tool for monitoring the surface temperature of animals. In this study, machine learning-based models for the individual assessment of thermal stress levels in dairy cows (Holstein) were established and evaluated considering both weather and animal factors. An experiment was performed with 26 lactating cows, which were monitored during summer and winter (40 nonconsecutive days in total; three times a day) to acquire the weather and physiological data including the rectal temperature (RT), respiration rate (RR), and body surface temperature (BST), measured by IRT in different body area. The data were analyzed with the Pearson correlation coefficient to determine the ideal body part (front, ocular area, rib, and flank regions) for computational modeling. In addition, a data analysis with linear regression was performed to enhance the parameters choices. The models based on artificial neural networks (ANNs) were developed based on the defined weather and physiological variables. The ANN-based models for predicting the RR (ANN-RR) and RT (ANN-RT) were established with Perceptron, feedforward, and multilayered architectures. The model responses were used as classifiers for the thermal stress levels (comfort, alert, danger, and emergency). The classification efficiency was assessed with metrics extracted from the confusion matrices (accuracy) and compared with the results of traditional classification methods for thermal stress levels: the Temperature-Humidity Index (THI) and Black Globe-Humidity Index (BGHI). The ANN models provided better predictions of the RR and RT with coefficient of determination (R-2) of 0.74 (ANN-RR) and 0.71 (ANN-RT) than the linear regression models. With regard to thermal stress levels, the ANN-based models demonstrated a good predictive ability compared with the BGHI and THI classifications. The best thermal stress accuracy predictions with ANN-RR and ANN-RT were 83% and 84%, respectively; the best accuracies of the BGHI- and THI-based classifiers were 68% and 55%, respectively. In addition, the ANN-based classifier enables an individual assessment of the thermal stress levels of animals.
更多查看译文
关键词
Precision livestock farming,Data mining,Thermal radiation,Animal welfare,Noninvasive measurement
AI 理解论文
溯源树
样例
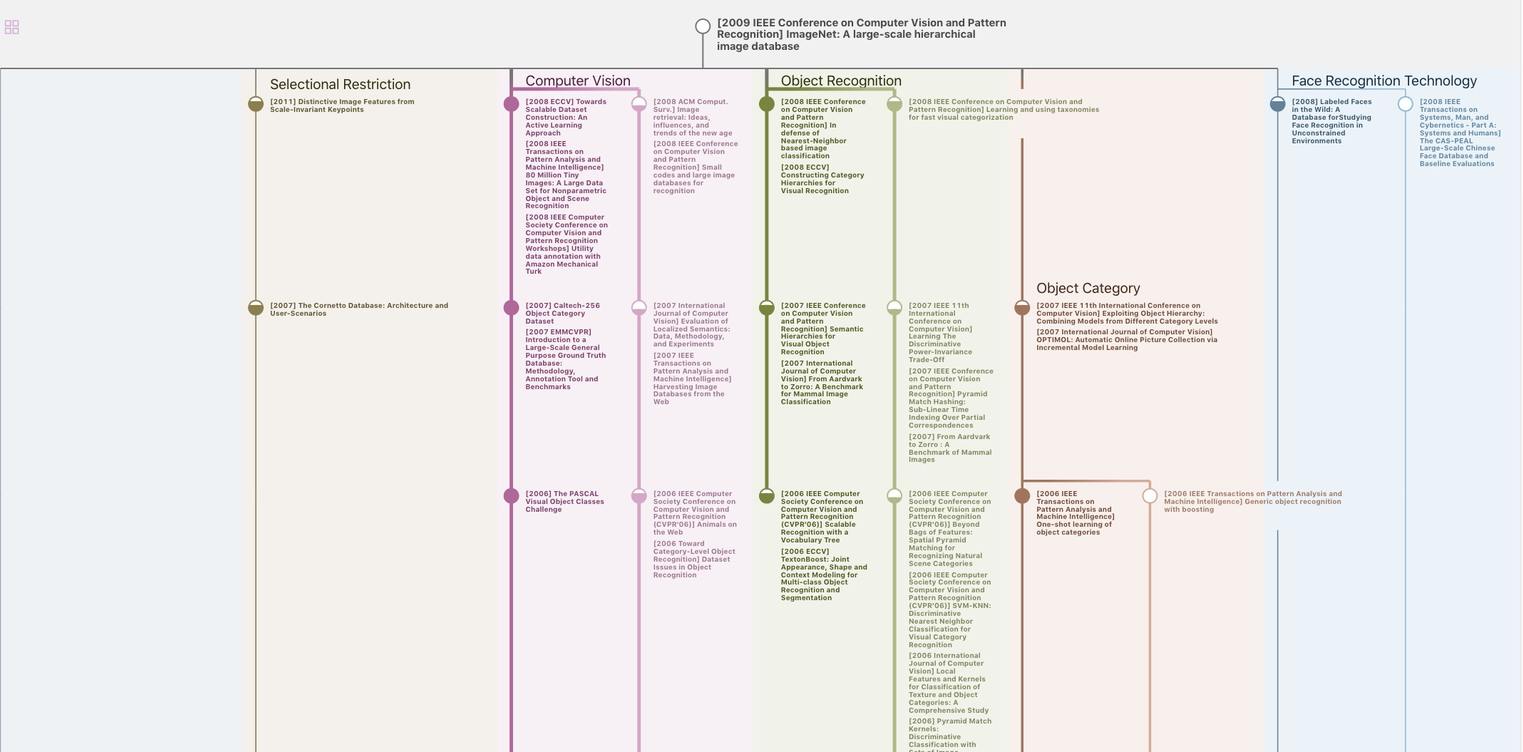
生成溯源树,研究论文发展脉络
Chat Paper
正在生成论文摘要