Data Multi-Scale Decomposition Strategies For Air Pollution Forecasting: A Comprehensive Review
JOURNAL OF CLEANER PRODUCTION(2020)
摘要
Currently, the increasingly serious air quality has attached great significance to the forecasting of air pollution. Data decomposition technology can decompose the original data into multiple frequency components, and its adoption significantly improves the performance of forecasting accuracy and robustness of the hybrid models. In recent years, many scholars have applied data decomposition technology to air pollution forecasting, and have achieved good forecasting results. This review classifies and summarizes the hybrid models based on data decomposition in the field of air pollution forecasting from the perspective of four data decomposition methods: empirical mode decomposition (EMD), variational mode decomposition (VMD), wavelet decomposition (WD), and secondary decomposition (SD). The purpose, usage methods, and characteristics of various decomposition methods are described comprehensively. This review considers their combination with models such as artificial neural networks (ANN), support vector machines (SVM), and extreme learning machines (ELM), and evaluates them from the perspective of usage frequency, accuracy improvement, advantages, limitations, and model development. In the existing data decomposition methods, the use of VMD and SD is relatively small, and they can be further studied in the future. In addition, some existing application challenges and future research directions of data decomposition in air pollution forecasting are given. (C) 2020 Elsevier Ltd. All rights reserved.
更多查看译文
关键词
Air pollution forecasting, Data decomposition, Hybrid model
AI 理解论文
溯源树
样例
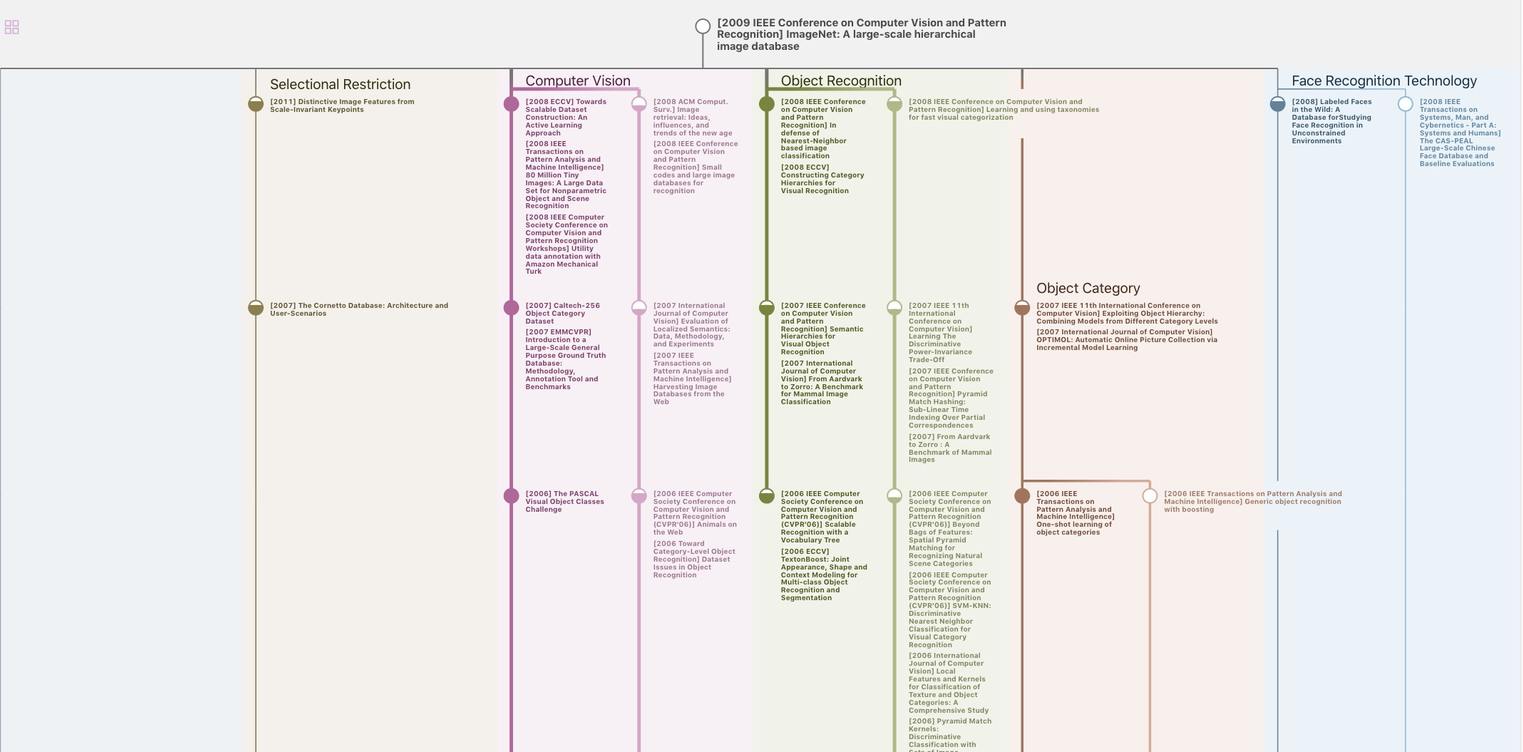
生成溯源树,研究论文发展脉络
Chat Paper
正在生成论文摘要