Unpaired salient object translation via spatial attention prior.
Neurocomputing(2021)
摘要
With only set-level constraints, unpaired image translation is challenging in discovering the correct semantic-level correspondences between two domains. This limitation often results in false positives such as significantly changing color and appearance of the background during image translation. To address this limitation, we propose the Spatial Attention-Aware Generative Adversarial Network (SAAGAN), a novel approach to jointly learn salient object discovery and translation. Specifically, our generator consists of (1) spatial attention prediction branch and (2) image translation branch. For attention branch, we extract spatial attention prior from a pre-trained classification network to provide weak supervision for object discovery. The proposed attention loss can largely stabilize the training process of attention-guided generator. For translation branch, we revise classical adversarial loss for salient object translation. Such a discriminator only distinguish the distribution of the object between two domains. What is more, we propose a fake sample augmentation strategy to provide extra spatial information for discriminator. Our approach allows simultaneously locating the attention areas in each image and translating the related areas between two domains. Extensive experiments and evaluations show that our model can achieve more realistic mappings compared to state-of-the-art unpaired image translation methods.
更多查看译文
关键词
Generative model,Adversarial learning,Image translation,Spatial attention
AI 理解论文
溯源树
样例
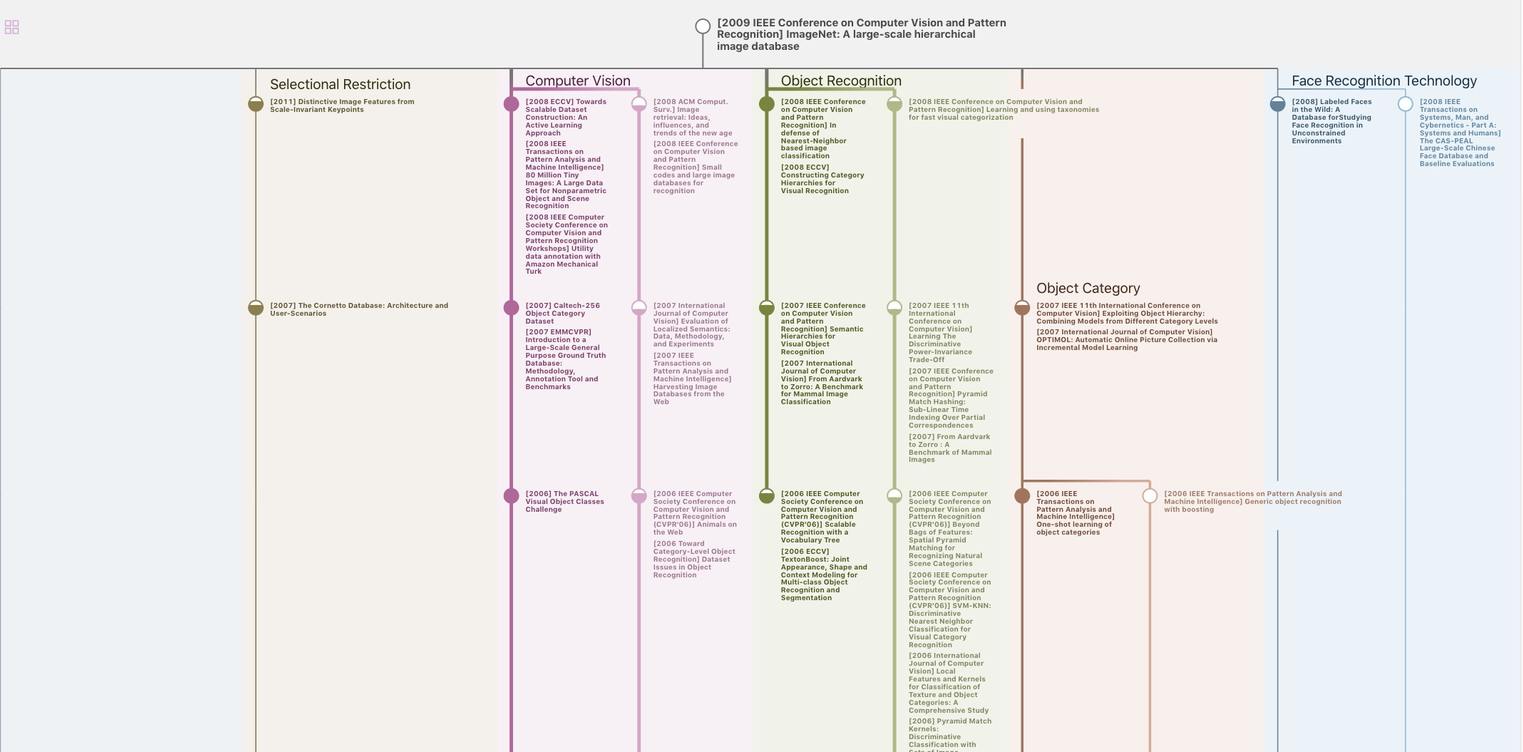
生成溯源树,研究论文发展脉络
Chat Paper
正在生成论文摘要