N-GlycoGo: Predicting Protein N-Glycosylation Sites on Imbalanced Data Sets by Using Heterogeneous and Comprehensive Strategy
IEEE ACCESS(2020)
摘要
Glycosylation is the most complex post-modification effect of proteins. It participates in many biological processes in the human body and is closely related to many disease states. Among them, N-linked glycosylation is the most contained glycosylation data. However, the current N-linked glycosylation prediction tool does not take into account the serious imbalance between positive and negative data. In this study, we used protein sequence and amino acid characteristics to construct an N-linked glycosylation prediction model called N-GlycoGo. Based on sequence, structure, and function, 11 heterogeneous features were encoded. Further, XGBoost was selected for modeling. Finally, independent testing of human and mouse prediction models showed that N-GlycoGo is superior to other tools with Matthews correlation coefficient (MCC) values of 0.397 and 0.719, respectively, which is higher than other glycosylation site prediction tools. We have developed a fast and accurate prediction tool, N-GlycoGo, for N-linked glycosylation. N-GlycoGo is available at http://ncblab.nchu.edu.tw/n-glycogo/.
更多查看译文
关键词
Proteins,Amino acids,Tools,Predictive models,Mice,Training,Encoding,Ensemble learning,machine learning,N-linked glycosylation
AI 理解论文
溯源树
样例
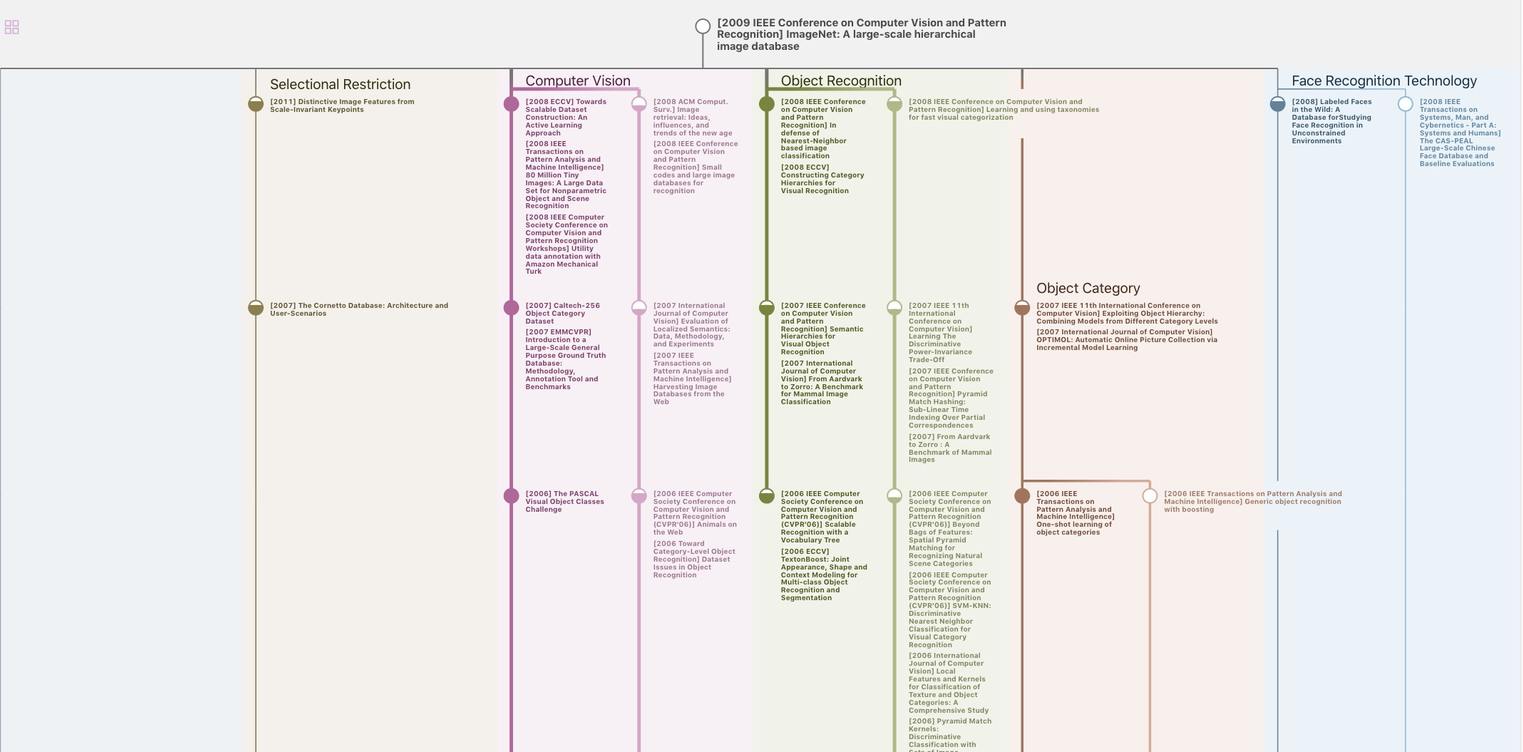
生成溯源树,研究论文发展脉络
Chat Paper
正在生成论文摘要