Using Electronic Health Records To Identify Patients With Apparent Treatment Resistant Hypertension
Hypertension(2020)
摘要
Introduction: Apparent treatment resistant hypertension (aTRH) affects 10-20% of hypertensive adults and increases risk of cardiovascular events and mortality. Fewer than half of these patients have true resistant hypertension. The majority experience pseudo-resistant hypertension due to inadequate medication adherence, white coat hypertension, and secondary causes of hypertension. We hypothesize that electronic health records can be leveraged to identify aTRH patients who would benefit from targeted counseling, medication reconciliation, and screening for secondary causes of hypertension. Methods: We studied electronic health record (EHR) data from 395 hypertensive adults in our primary care population who received longitudinal care between 2007 and 2017. Patients who met the 2008 AHA definition of resistant hypertension by chart review were considered to have aTRH. We also included 100 patients identified by heuristics targeting secondary hypertension. We extracted from the EHR demographics, vitals, laboratory results, diagnosis codes, and medications. Results outside of physiologic range were excluded and median imputation was used to handle missing data. Random forest model performance was assessed by 5-fold cross validation. Model discrimination was evaluated at an estimated positive predictive value of 75%. Results: The prevalence of aTRH in our randomly selected and full cohorts was 20.3% (n=295) and 25.8% (n=395), respectively. In cross-validation, the random forest model demonstrated a median sensitivity of 65% (IQR: 60% - 65%) and a median AUROC of 0.92 (IQR: 0.90 - 0.92). The most influential variables were related to the prescription of three or more hypertension medications; number of days on diuretics, angiotensin-converting enzyme inhibitors, or angiotensin II receptor blockers; systolic blood pressure measurements; and hypertension or diabetes diagnosis codes. Conclusion: EHR data can be used to accurately identify patients with aTRH. We expect the implementation of a clinical decision support system leveraging such models could lead to the improved care for aTRH patients.
更多查看译文
AI 理解论文
溯源树
样例
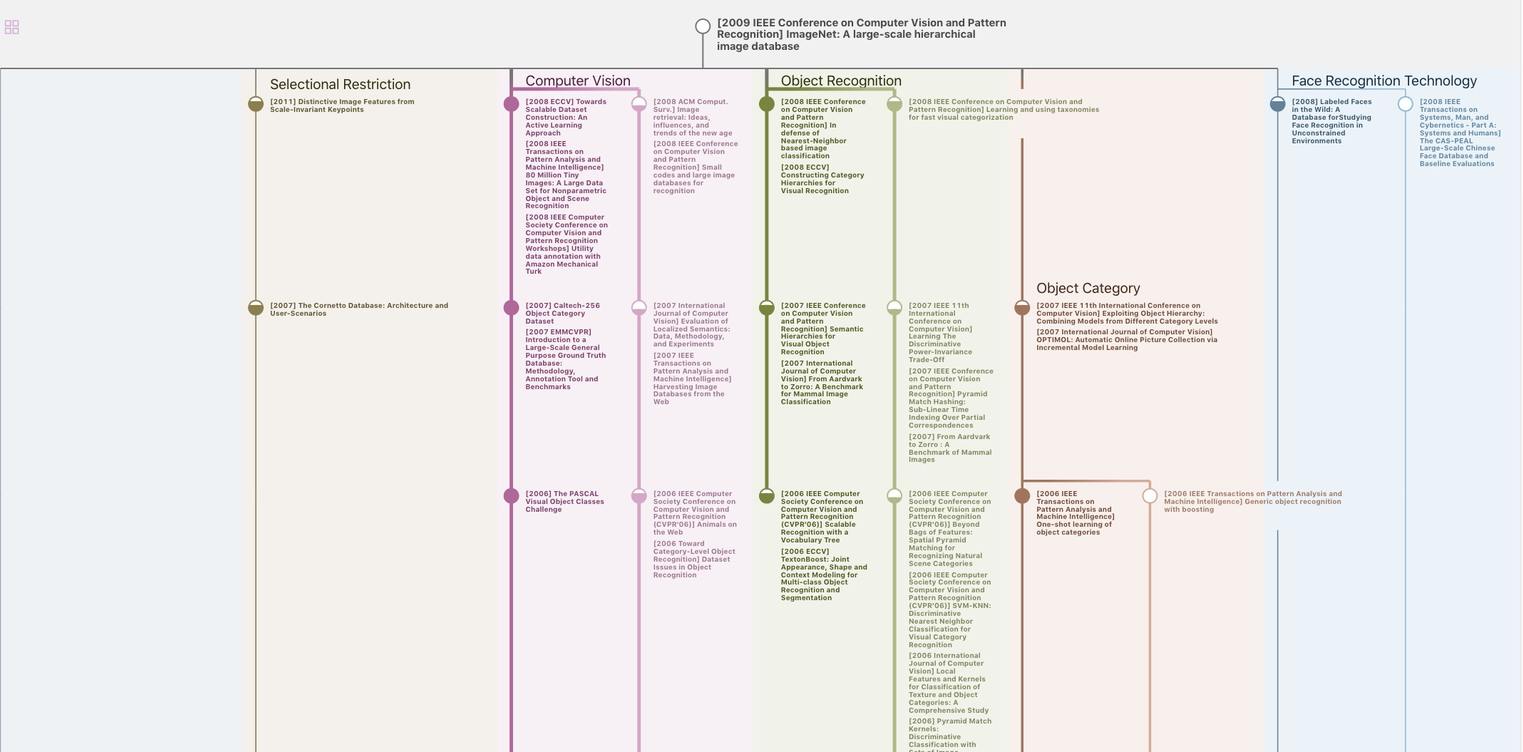
生成溯源树,研究论文发展脉络
Chat Paper
正在生成论文摘要