A residual neural network based method for the classification of tobacco cultivation regions using near-infrared spectroscopy sensors
Infrared Physics & Technology(2020)
摘要
•A novel neural network with two residual blocks is proposed to improve the feature extraction ability of high-dimensional and redundant spectral data. Due to the high dimension of tobacco spectral data, a deep neural network is used to extract the features of spectral data. Two residual blocks are added into the proposed neural network to do identity mapping, which can map the data in a shallow layer to a deep layer. The identity mapping can effectively solve the issue of gradient disappearance that can increase the feature quantity of a deep neural network.•The balance and suppression factors are added to the loss function of the proposed neural networks, which solve the issue in training unevenly distributed data. The spectral data size of tobacco collected from different tobacco cultivation regions varies. In the training process of a neural network, the balance and suppression factors add more weight to small-size data samples. Therefore, the neural network can have the same classification ability for each data sample.•A parametric rectified linear unit (PReLU) function is used to add linear factors to the negative input, which can adaptively learn parameters in the network. In the network, batch normalization (BN) operation is used to speed up the network training and improve the generalization ability of the network. In addition, the exponential decay learning rate is applied to the control of learning speed. As a deep learning optimization method, dropout is used to avoid overfitting, improve the generalization performance of network, and make the model training converge faster.
更多查看译文
关键词
Tobacco leaves,Near-infrared spectroscopy,Residual network,Focal loss,Cultivation regions
AI 理解论文
溯源树
样例
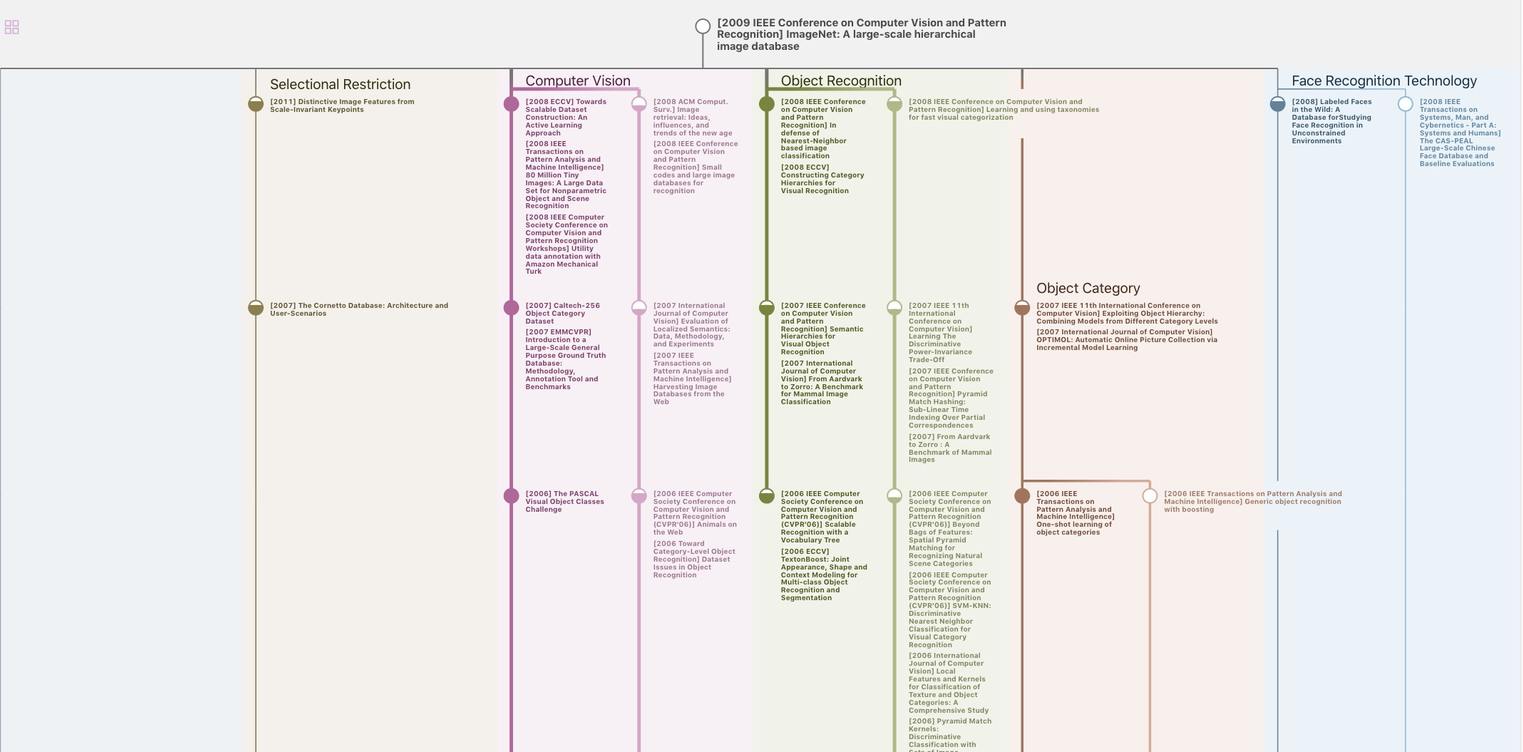
生成溯源树,研究论文发展脉络
Chat Paper
正在生成论文摘要