Deep or Simple Models for Semantic Tagging? It Depends on your Data.
Proc. VLDB Endow.(2020)
摘要
Semantic tagging, which has extensive applications in text mining, predicts whether a given piece of text conveys the meaning of a given semantic tag. The problem of semantic tagging is largely solved with supervised learning and today, deep learning are widely perceived to be better for semantic tagging. However, there is no comprehensive study supporting the popular belief. Practitioners often have to train different types of for each semantic tagging task to identify the best model. This process is both expensive and inefficient.We embark on a systematic study to investigate the following question: Are deep the best performing model for all semantic tagging tasks? To answer this question, we compare deep against models over datasets with varying characteristics. Specifically, we select three prevalent deep (i.e. CNN, LSTM, and BERT) and two simple (i.e. LR and SVM), and compare their performance on the semantic tagging task over 21 datasets. Results show that the size, the label ratio, and the label cleanliness of a dataset significantly impact the quality of semantic tagging. Simple achieve similar tagging quality to deep on large datasets, but the runtime of simple is much shorter. Moreover, simple can achieve better tagging quality than deep when targeting datasets show worse label cleanliness and/or more severe imbalance. Based on these findings, our study can systematically guide practitioners in selecting the right learning model for their semantic tagging task.
更多查看译文
AI 理解论文
溯源树
样例
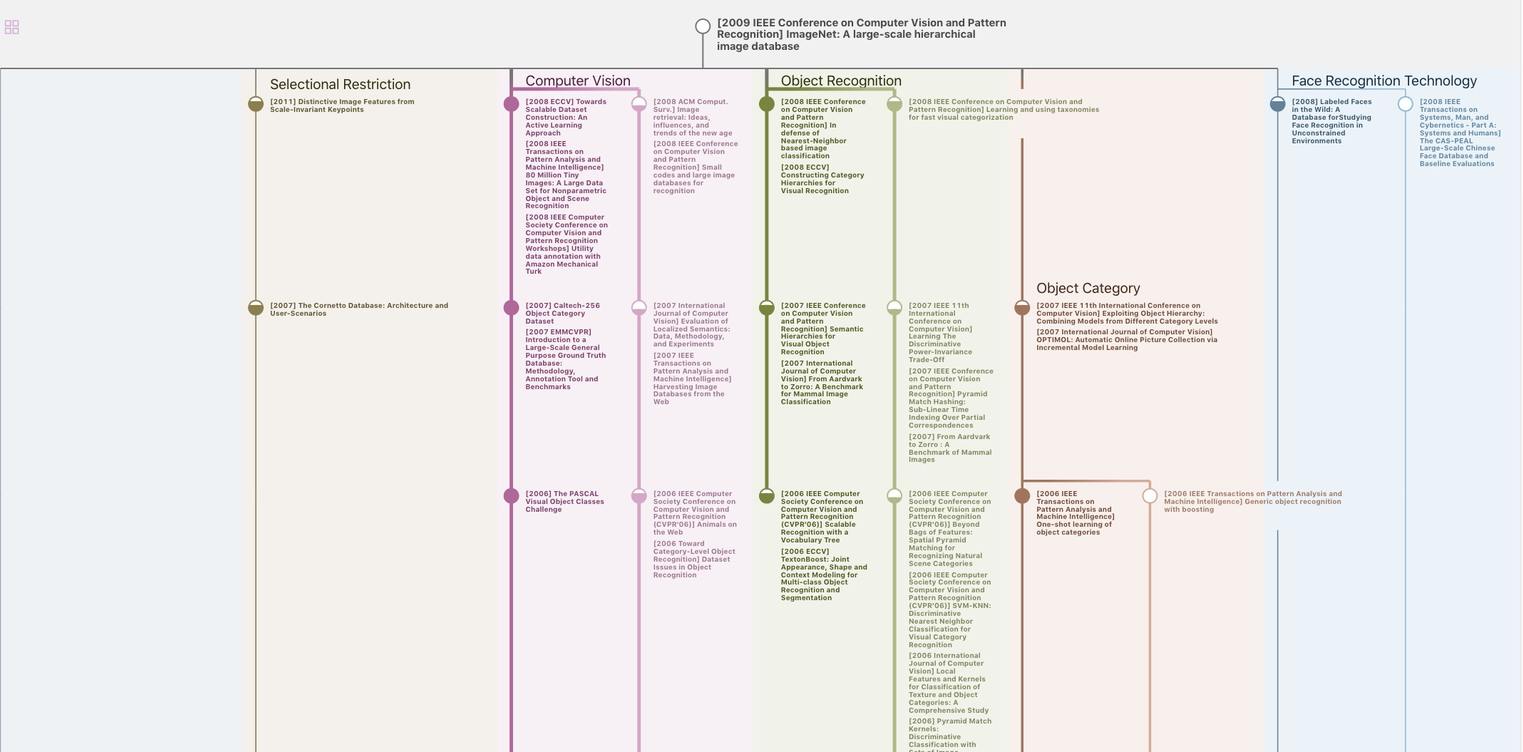
生成溯源树,研究论文发展脉络
Chat Paper
正在生成论文摘要