Hierhar: Sensor-Based Data-Driven Hierarchical Human Activity Recognition
IEEE SENSORS JOURNAL(2021)
摘要
Pervasive computing greatly advances the automatic recognition and understanding of human activities and effectively bridges the gap between the low-level sensor signals and high-level human-centric applications. The inherent complexity of human behavior, however, inevitably poses a huge challenge to the design of a robust activity recognizer, especially in classifying similar activities. In this study, we present a hierarchical framework, named HierHAR, that infers on-going activities in a multi-stage process for better distinguishing similar activities and improving the overall performance. Specifically, we propose a data-driven approach, rather than heavily rely on prior domain knowledge, to automatically determining the relationships among activities. Afterwards, we use the relationships to organize the activities into a tree structure and accordingly construct and train a tree-based activity recognition model. Furthermore, we train a graph-based model that aims to reduce the compounding errors induced by the prediction process of the tree-based model. Finally, extensive comparative experiments are conducted on public datasets and results demonstrate the power of HierHAR in facilitating the automatic organization of activities and the design of hierarchical recognizers without prior knowledge about activities. Besides, the graph-based activity recognizer generally generalizes better across different scenarios and outperforms the tree-based model.
更多查看译文
关键词
Hidden Markov models, Activity recognition, Accelerometers, Adaptation models, Training, Radiofrequency identification, Pervasive computing, activity recognition, data-driven, information fusion
AI 理解论文
溯源树
样例
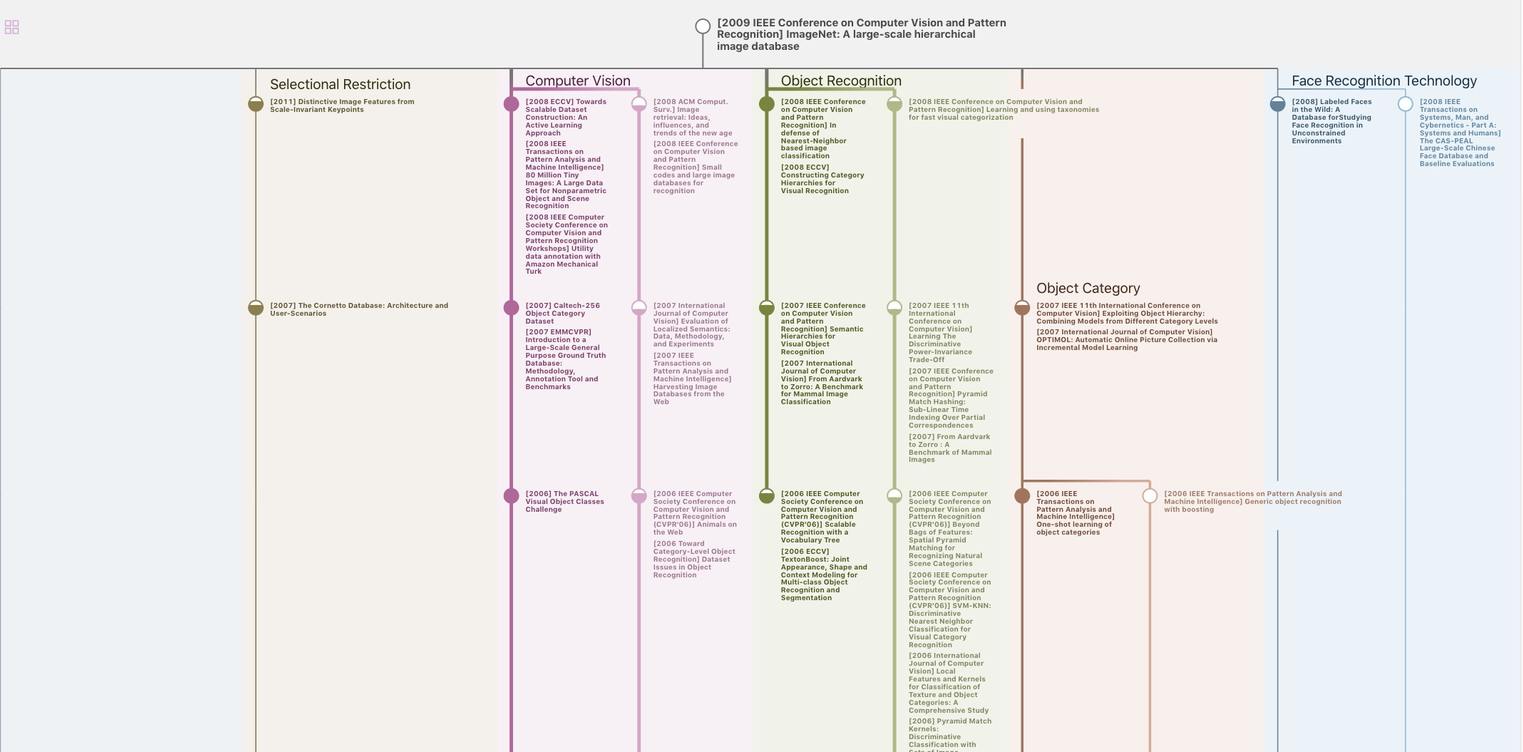
生成溯源树,研究论文发展脉络
Chat Paper
正在生成论文摘要