An Adversarial Learning Model for Intrusion Detection in Real Complex Network Environments.
WASA (1)(2020)
摘要
Network intrusion detection plays an important role in network security. With the deepening of machine learning research, especially the generative adversarial networks (GAN) proposal, the stability of the anomaly detector is put forward for higher requirements. The main focus of this paper is on the security of machine learning based anomaly detectors. In order to detect the robustness of the existing advanced anomaly detection algorithm, we propose an anomaly detector attack framework MACGAN (maintain attack features based on the generative adversarial networks). The MACGAN framework consists of two parts. The first part is used to analyze the attack fields manually. Then, the learning function of GAN in the second part is used to bypass the anomaly detection. Our framework is tested on the latest Kitsune2018 and CICIDS2017 data sets. Experimental results demonstrate the ability to bypass the state-of-the-art machine learning algorithms. This greatly helps the network security researchers to improve the stability of the detector.
更多查看译文
关键词
adversarial learning model,intrusion detection,real complex network environments
AI 理解论文
溯源树
样例
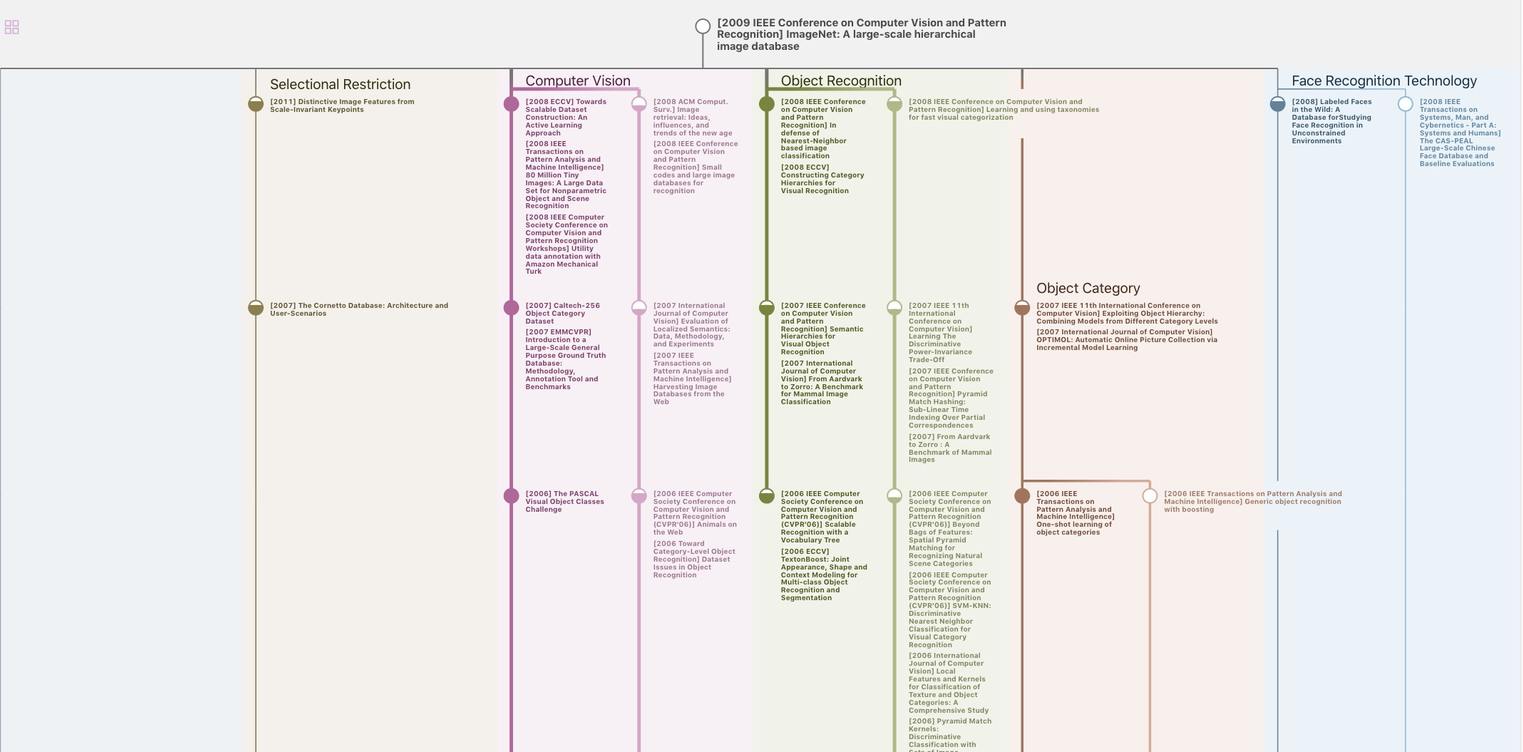
生成溯源树,研究论文发展脉络
Chat Paper
正在生成论文摘要