Detecting Excessive Load-Carrying Tasks Using A Deep Learning Network With A Gramian Angular Field
AUTOMATION IN CONSTRUCTION(2020)
摘要
Manual load carrying without sufficient rest may cause work-related musculoskeletal disorders (WMSDs) and needs to be monitored at construction sites. While previous studies have been able to predict load-carrying modes using multiple wearable inertial measurement unit (IMU) sensors, wearing multiple sensors obtrudes on workers during various construction tasks. In this context, by using a single IMU sensor, this research proposes an automatic detecting technique for excessive carrying -load (DeTECLoad) to predict load-carrying weights and postures simultaneously. DeTECLoad converts the IMU data into image data using a Gramian Angular Field, and then uses a hybrid Convolutional Neural Network-Long Short-Term Memory to classify load-carrying modes from the image data. DeTECLoad provides 92.46% and 96.33% accuracies for the load-carrying weight and posture classifications, respectively. By exploiting DeTECLoad, a construction worker's excessive load-carrying tasks could be managed in situ, helping to prevent construction site WMSDs.
更多查看译文
关键词
Construction safety, Overexertion, Worker monitoring, Wearable computing, Load carrying
AI 理解论文
溯源树
样例
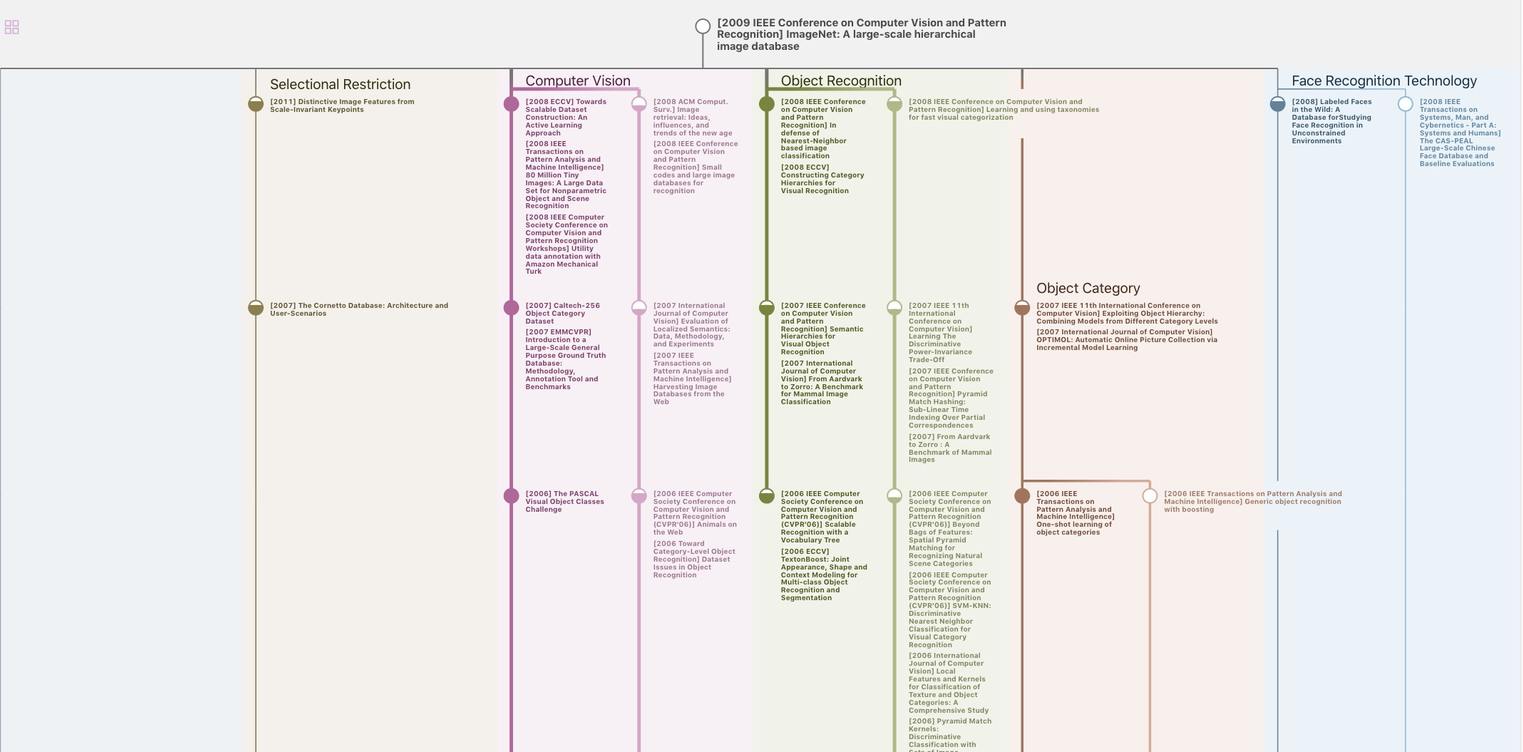
生成溯源树,研究论文发展脉络
Chat Paper
正在生成论文摘要