A stepwise interpretable machine learning framework using linear regression (LR) and long short-term memory (LSTM): City-wide demand-side prediction of yellow taxi and for-hire vehicle (FHV) service
TRANSPORTATION RESEARCH PART C-EMERGING TECHNOLOGIES(2020)
摘要
As app-based ride-hailing services have been widely adopted within existing traditional taxi markets, researchers have been devoted to understand the important factors that influence the demand of the new mobility. Econometric models (EMs) are mainly utilized to interpret the significant factors of the demand, and deep neural networks (DNNs) have been recently used to improve the forecasting performance by capturing complex patterns in the large datasets. However, to mitigate possible (induced) traffic congestion and balance utilization rates for the current taxi drivers, an effective strategy of proactively managing a quota system for both emerging services and regular taxis is still critically needed. This paper aims to systematically design an explainable deep learning model capable of assessing the quota system balancing the demand volumes between two modes. A two-stage interpretable machine learning modeling framework was developed by a linear regression (LR) model, coupled with a neural network layered by long short-term memory (LSTM). The first stage investigates the correlation between the existing taxis and on-demand ride-hailing services while controlling for other explanatory variables. The sec ond stage fulfills the long short-term memory (LSTM) network structure, capturing the residuals from the first estimation stage in order to enhance the forecasting performance. The proposed stepwise modeling approach (LR-LSTM) forecasts the demand of taxi rides, and it is implemented in the application of pick-up demand prediction using New York City (NYC) taxi data. The experiment result indicates that the integrated model can capture the inter-relationships between existing taxis and ride-hailing services as well as identify the influence of additional factors, namely, the day of the week, weather, and holidays. Overall, this modeling approach can be applied to construct an effective active demand management (ADM) for the short-term period as well as a quota control strategy between on-demand ride-hailing services and traditional taxis.
更多查看译文
关键词
On-demand ride hailing,Taxi market,Short-term demand forecasting,Long short-term memory (LSTM),Deep neural network (DNN),Active demand management (ADM)
AI 理解论文
溯源树
样例
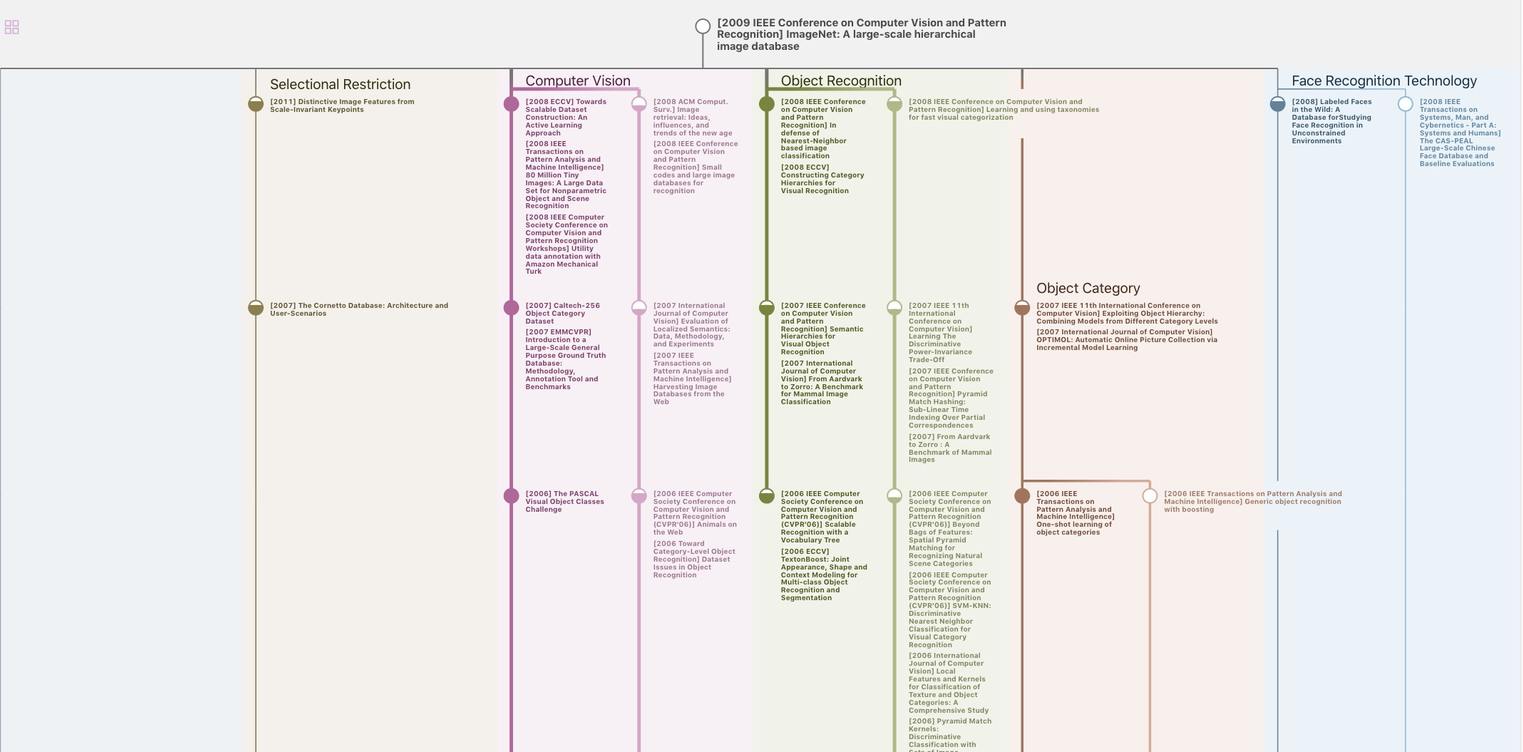
生成溯源树,研究论文发展脉络
Chat Paper
正在生成论文摘要