Wavelet-based dynamic and privacy-preserving similitude data models for edge computing
WIRELESS NETWORKS(2020)
摘要
The privacy-preserving data release is an increasingly important problem in today’s computing. As the end devices collect more and more data, reducing the amount of published data saves considerable network, CPU and storage resources. The savings are especially important for constrained end devices that collect and send large amounts of data, especially over wireless networks. We propose the use of query-independent, similitude models for privacy-preserving data release on the end devices. The conducted experiments validate that the wavelet-based similitude model maintains an accuracy compared to other state-of-the-art methods while compressing the model. Expanding on our previous work (Derbeko et al. in: Cyber security cryptography and machine learning-second international symposium, CSCML 2018, Beer Sheva, Israel, 2018) we show how wavelet-based similitude models can be combined and “subtracted” when new end devices appear or leave the system. Experiments show that accuracy is the same or improved with a model composition. This data-oriented approach allows further processing near the end devices in a fog or a similar edge computing concept.
更多查看译文
关键词
Datasets,Neural networks,Gaze detection,Text tagging,Wavelet
AI 理解论文
溯源树
样例
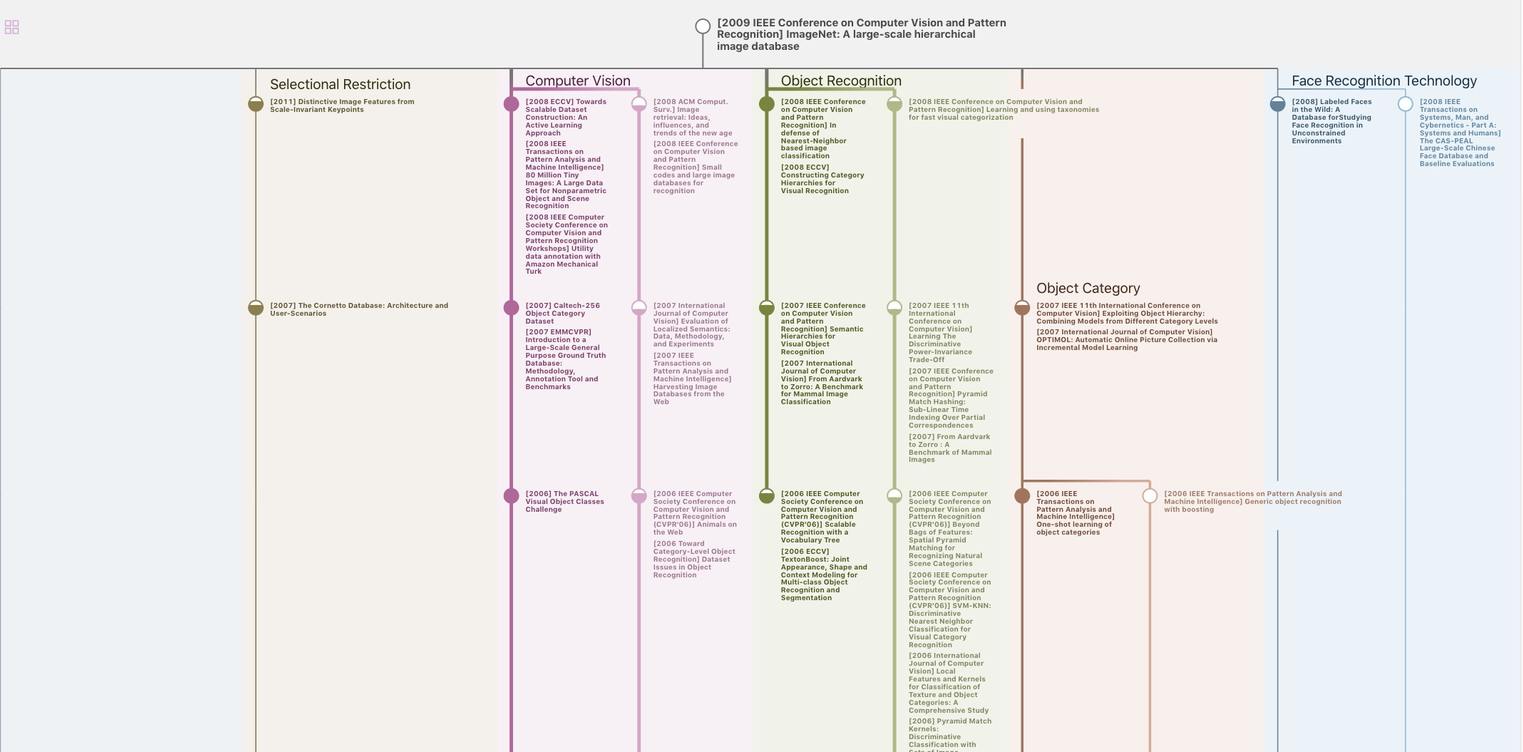
生成溯源树,研究论文发展脉络
Chat Paper
正在生成论文摘要