Nonlinear Predictability of Stock Returns? Parametric Versus Nonparametric Inference in Predictive Regressions
JOURNAL OF BUSINESS & ECONOMIC STATISTICS(2022)
摘要
Nonparametric test procedures in predictive regressions havelimiting null distributions under both low and high regressor persistence, but low local power compared to misspecified linear predictive regressions. We argue that IV inference is better suited (in terms of local power) for analyzing additive predictive models with uncertain predictor persistence. Then, a two-step procedure is proposed for out-of-sample predictions. For the current estimation window, one first tests for predictability; in case of a rejection, one predicts using a nonlinear regression model, otherwise the historic average of the stock returns is used. This two-step approach performs better than competitors (though not by a large margin) in a pseudo-out-of-sample prediction exercise for the S&P 500.
更多查看译文
关键词
Chi-square distribution, Endogeneity, Nonlinear regression function, Predictive regression, Time-varying variance, Unknown persistence
AI 理解论文
溯源树
样例
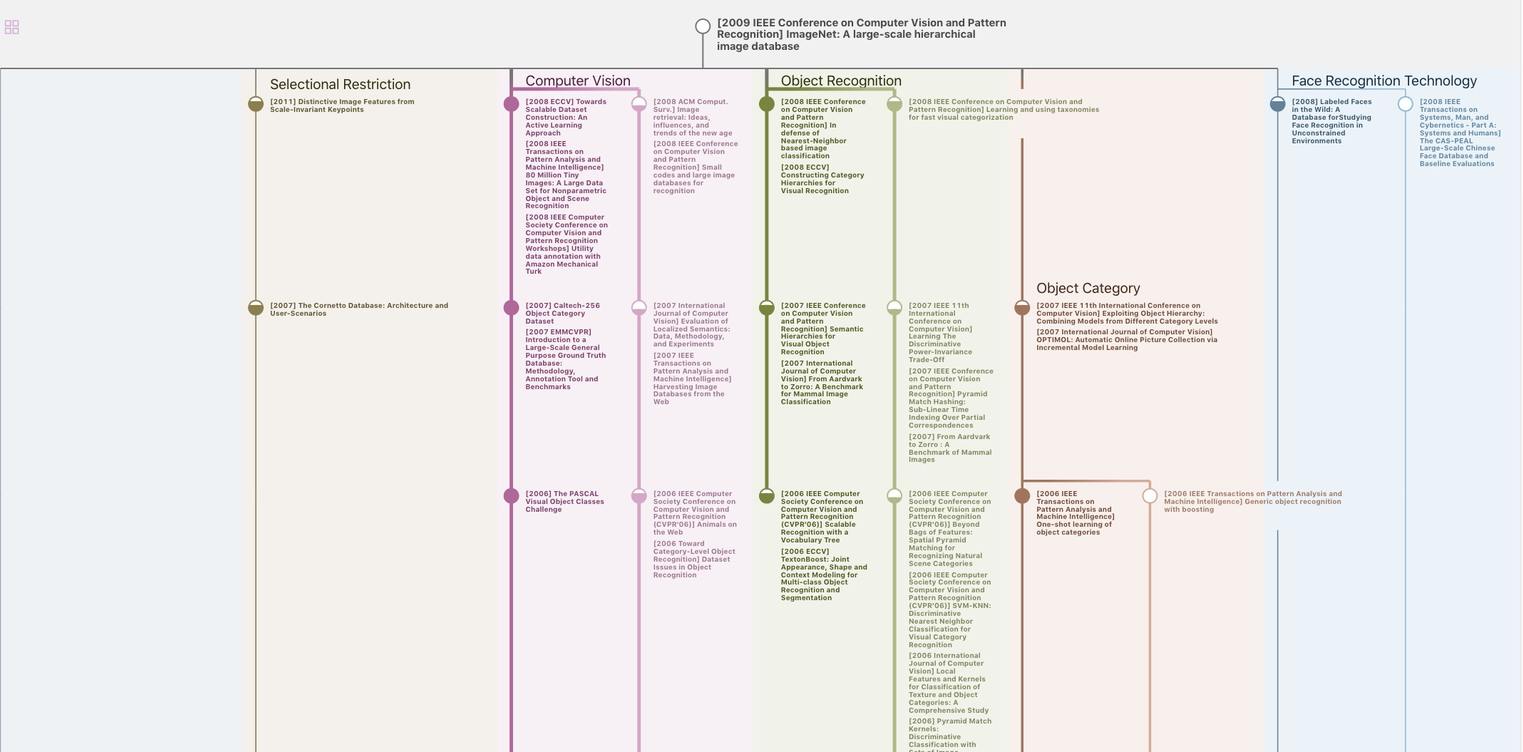
生成溯源树,研究论文发展脉络
Chat Paper
正在生成论文摘要