A new multi-task learning framework for fuel cell model outputs in high-dimensional spaces
Journal of Power Sources(2021)
摘要
In this paper we develop a multi-output, multi-task framework for approximating the outputs of complex physics-based computer models of fuel cells and batteries. For applications that require real-time or a high number of runs, such as optimization and control, the original computer model will be impractical, making such approximations necessary. It is normally the case that there are several quantities of interest (different tasks) from the model, such as the potential, reactant distributions and the cell voltage charge–discharge profile. We overcome several challenges in this paper: dealing with very high dimensional outputs (e.g., a distribution defined at many grid points), accounting for correlations between tasks, and accounting for noise in the outputs or missing information. We combine a multivariate Gaussian process (GP) model based on dimension reduction with a linear model of coregionalisation (LMC) to account for the between-task covariances. The LMC applies to coefficients in a low-dimensional subspace (each coefficient corresponding to a particular task), which results in highly-efficient training. We test the framework on two different 3-d hydrogen fuel cell models and compare the results to several alternative multivariate GP approaches. The results reveal that our framework is more accurate and also more computationally efficient.
更多查看译文
关键词
Fuel cells,Computer model,Multi-task,Machine learning,Gaussian process model,Linear model of coregionalisation
AI 理解论文
溯源树
样例
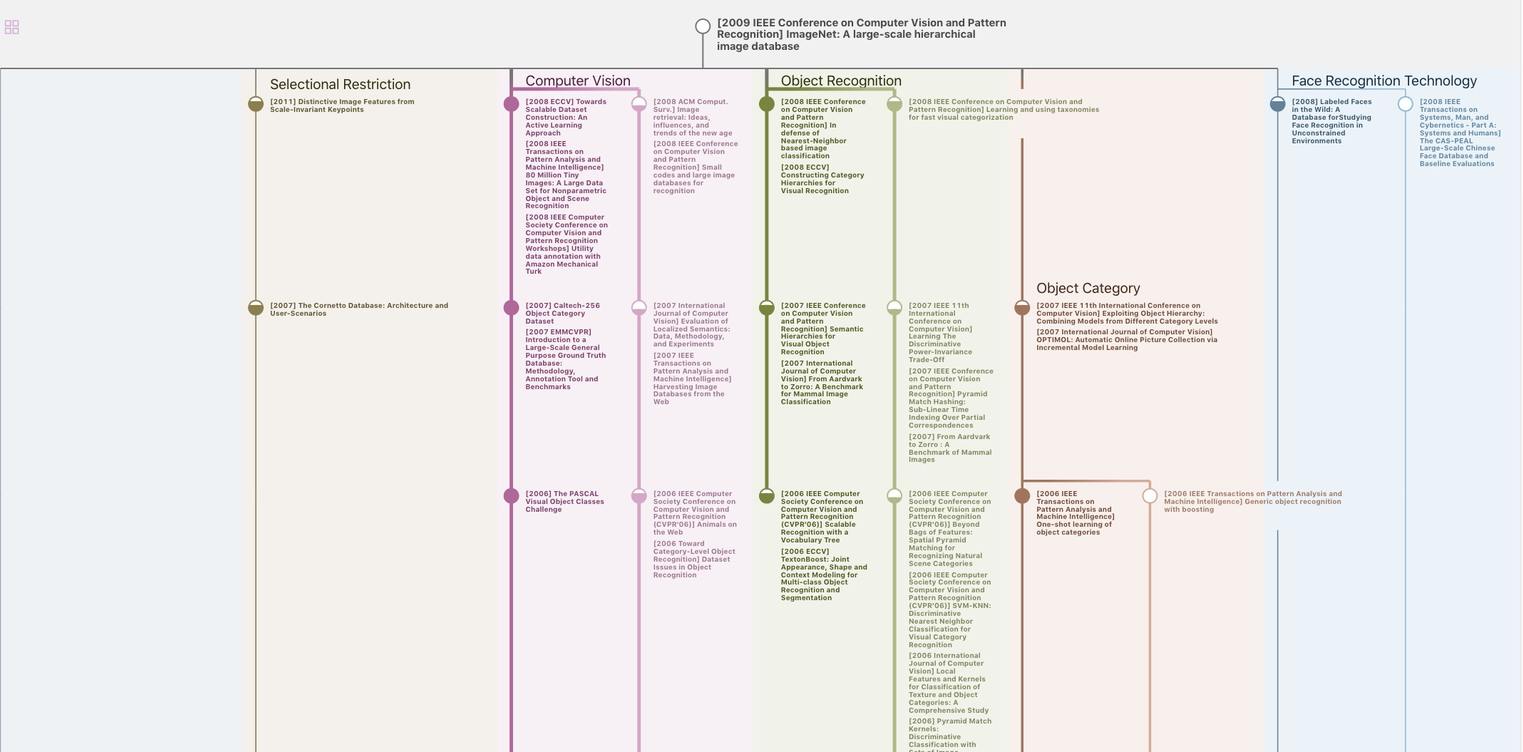
生成溯源树,研究论文发展脉络
Chat Paper
正在生成论文摘要