Surgical phase recognition by learning phase transitions
Current Directions in Biomedical Engineering(2020)
摘要
Abstract Automatic recognition of surgical phases is an important component for developing an intra-operative context-aware system. Prior work in this area focuses on recognizing short-term tool usage patterns within surgical phases. However, the difference between intra- and inter-phase tool usage patterns has not been investigated for automatic phase recognition. We developed a Recurrent Neural Network (RNN), in particular a state-preserving Long Short Term Memory (LSTM) architecture to utilize the long-term evolution of tool usage within complete surgical procedures. For fully automatic tool presence detection from surgical video frames, a Convolutional Neural Network (CNN) based architecture namely ZIBNet is employed. Our proposed approach outperformed EndoNet by 8.1% on overall precision for phase detection tasks and 12.5% on meanAP for tool recognition tasks.
更多查看译文
关键词
cnn,endoscopic videos,rnn,state-preserving lstm,surgical workflow,surgical phase recognition
AI 理解论文
溯源树
样例
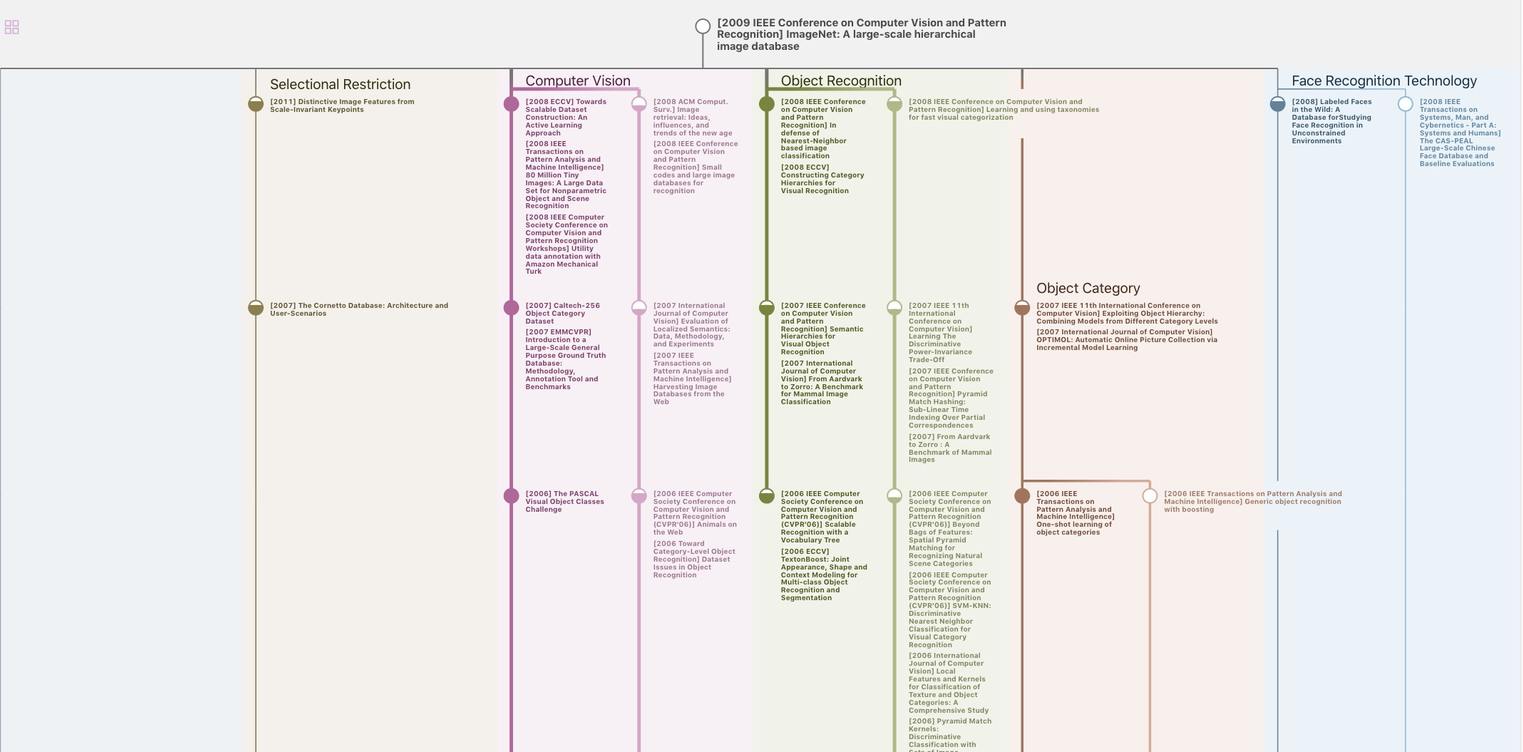
生成溯源树,研究论文发展脉络
Chat Paper
正在生成论文摘要