Construction Of Feedforward Neural Networks With Simple Architectures And Approximation Abilities
MATHEMATICAL METHODS IN THE APPLIED SCIENCES(2021)
摘要
The universal approximation property of feedforward neural networks (FNNs) is the basis for all FNNs applications. In almost all existing FNN approximation studies, it is always assumed that the activation function of the network satisfies certain conditions, such as sigmoid or bounded continuity. This paper focuses on building simple architecture FNNs with approximation ability by constructing an activation function. First, for any continuous function defined on[0, 1]and an arbitrary accuracy epsilon > 0, an FNN is constructed, which has only one neuron and a fixed weight 1 and can approximate the function with an accuracy of epsilon. Thereafter, we study the representation of FNNs for polynomial functions by constructing a proper activation function. It is proved that any algebraic polynomial with the degreencan be represented by an FNN. Further, a piecewise function is constructed as an activation function of an FNN such that the FNN represents the famous Bernstein polynomials.
更多查看译文
关键词
activation function, approximation, feedforward neural networks, representation
AI 理解论文
溯源树
样例
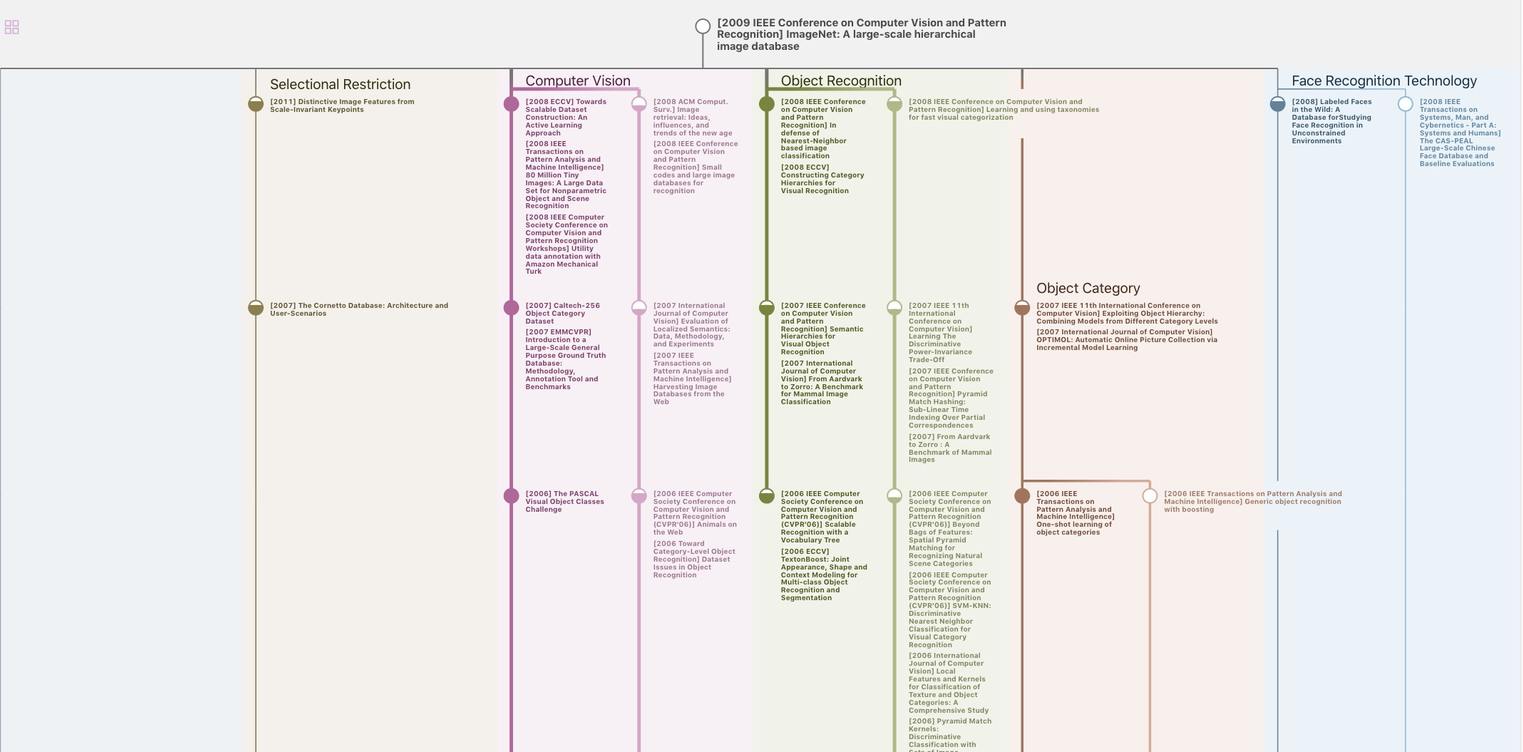
生成溯源树,研究论文发展脉络
Chat Paper
正在生成论文摘要