An Approach for Handling Uncertainties Related to Behaviour and Vehicle Mixes in Traffic Simulation Experiments with Automated Vehicles
JOURNAL OF ADVANCED TRANSPORTATION(2020)
摘要
The introduction of automated vehicles is expected to affect traffic performance. Microscopic traffic simulation offers good possibilities to investigate the potential effects of the introduction of automated vehicles. However, current microscopic traffic simulation models are designed for modelling human-driven vehicles. Thus, modelling the behaviour of automated vehicles requires further development. There are several possible ways to extend the models, but independent of approach a large problem is that the information available on how automated vehicles will behave is limited to today's partly automated vehicles. How future generations of automated vehicles will behave will be unknown for some time. There are also large uncertainties related to what automation functions are technically feasible, allowed, and actually activated by the users, for different road environments and at different stages of the transition from 0 to 100% of automated vehicles. This article presents an approach for handling several of these uncertainties by introducing conceptual descriptions of four different types of driving behaviour of automated vehicles (Rail-safe, Cautious, Normal, and All-knowing) and presents how these driving logics can be implemented in a commonly used traffic simulation program. The driving logics are also linked to assumptions on which logic that could operate in which environment at which part of the transition period. Simulation results for four different types of road facilities are also presented to illustrate potential effects on traffic performance of the driving logics. The simulation results show large variations in throughput, from large decreases to large increases, depending on driving logic and penetration rate.
更多查看译文
关键词
Driver Behavior,Microscopic Simulation,Traffic Assignment,Simulation Models,Crash Prediction Models
AI 理解论文
溯源树
样例
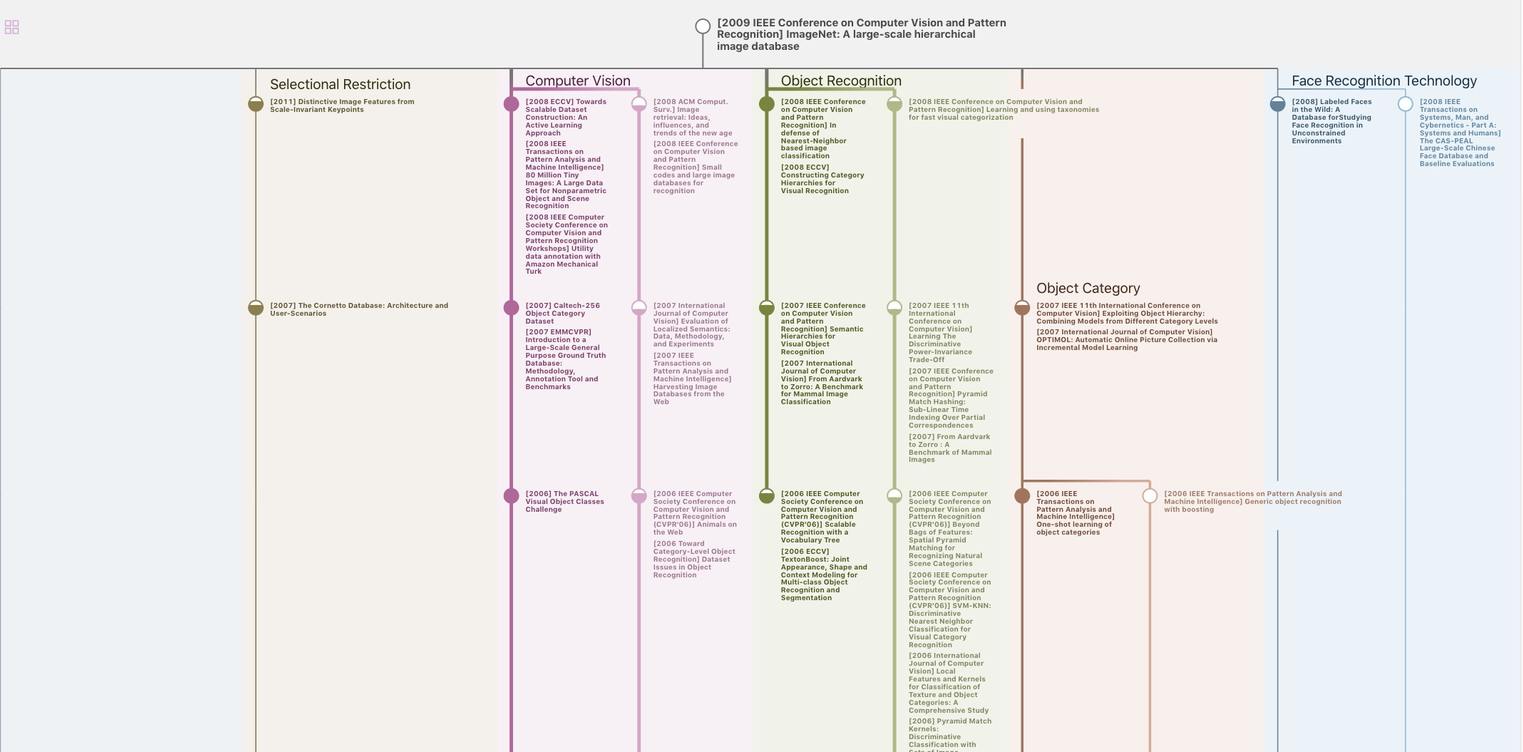
生成溯源树,研究论文发展脉络
Chat Paper
正在生成论文摘要