Automatic Detection of Negative Symptoms in Schizophrenia via Acoustically Measured Features Associated With Affective Flattening
IEEE Transactions on Automation Science and Engineering(2021)
摘要
Among the characteristic symptoms of schizophrenia, negative symptoms are a category. The existence of negative symptoms results in a diminution of normal behaviors and functions for schizophrenic patients. In this research, we focus on the affective flattening of negative symptoms to explore potential biomarkers to achieve automatic diagnosis. This work proposes an automatic procedure for detecting the negative symptoms of schizophrenic patients based on speech signal processing. In this procedure, three features are initially proposed: the symmetric spectral difference level (SSDL), quantization error and vector angle (QEVA), and standard dynamic volume value (SDVV). The SSDL feature is designed with the aim of emphasizing spectral differences that contribute to the evaluation of emotional richness. The QEVA is proposed to reflect the variations in tone using one cumulative error indicator and one variation evaluation indicator. The SDVV feature aims to represent the modulation of speech intensity, considering the speaking behavior of schizophrenic patients. Experiments evaluating the discriminative capabilities of the three features are conducted using a speech database collected from 56 participants (28 schizophrenic patients and 28 healthy controls). The classifier employed in these experiments is a simple decision tree. Three other binary classifiers [linear discrimination (LD), logistic regression (LR), and a support vector machine (SVM)] are also tested to compare their performances with the decision tree in this work. Based on the decision tree classifier, the discrimination accuracy levels of schizophrenic patients and control subjects using the SDLL, QEVA, and SDVV features are in the range of 80.5%-83%, 65%-73%, and 87%- 91.5%, respectively. When the three features are combined, the best discrimination accuracy of schizophrenic patients and control subjects is 98.2%, with an area under the receiver operations characteristic curve (AUC) value of 98%. Note to Practitioners-This article is motivated by the problems of the diagnosis of negative symptoms in schizophrenia and the timely monitoring of schizophrenic patients. In clinic, there exists a high patient-to-clinician ratio and the diagnostic result depends on the subjective experience of the clinician. It is necessary to develop an automatic and objective procedure for the diagnosis and monitoring of schizophrenic patients. Speech disorders are among the salient characteristics of negative symptoms. In this work, an automatic procedure for detecting the negative symptoms based on speech signal processing is proposed. This automatic procedure is achieved based on three newly presented acoustic features involving the characteristics of schizophrenic speech, which consider the speaking behavior of schizophrenic patients. This automatic procedure and the information of the three acoustic features may serve as an aid to clinicians and could potentially help them in providing better monitoring of schizophrenic patients.
更多查看译文
关键词
Affective flattening,intensity modulation,negative symptoms,quantification of tone variations,schizophrenia,symmetrical spectral differences
AI 理解论文
溯源树
样例
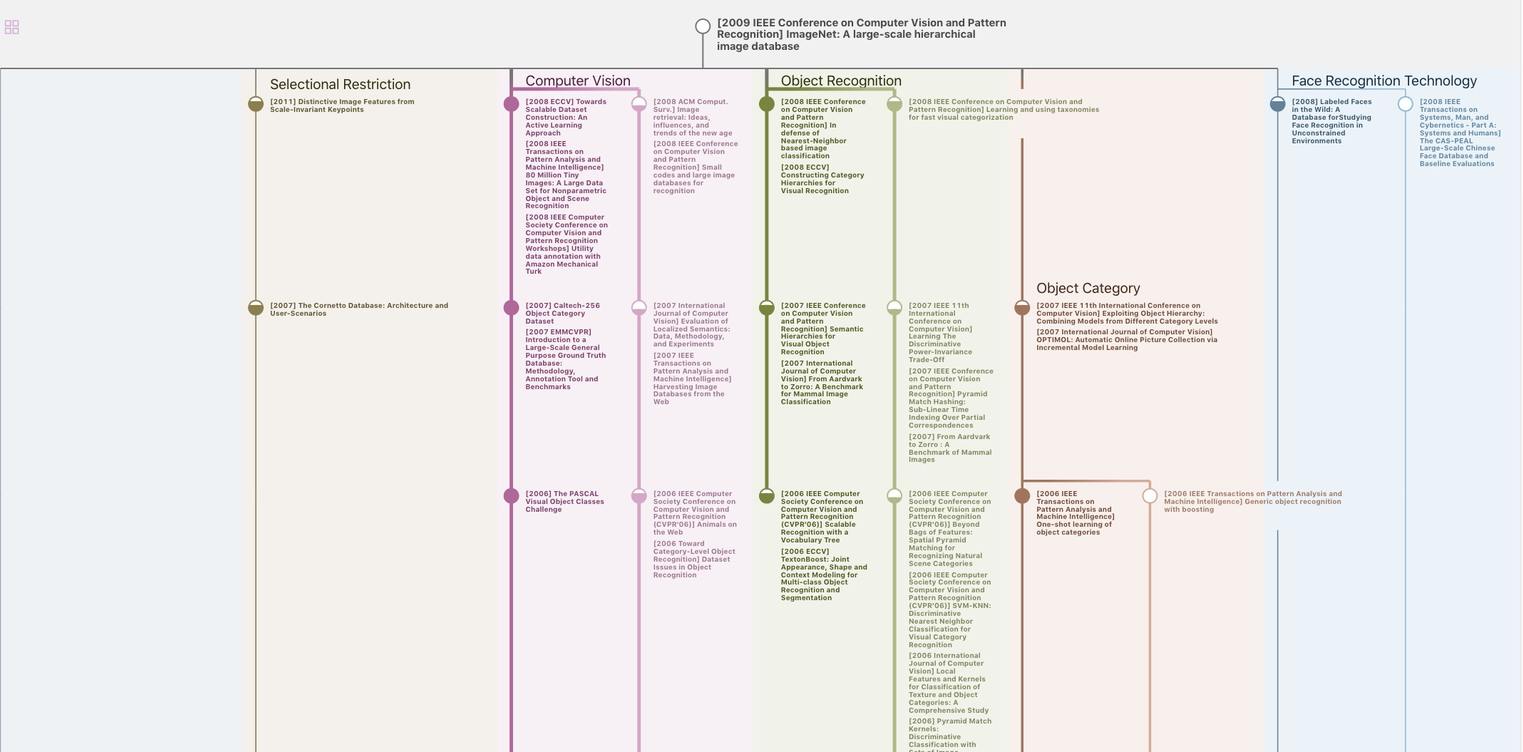
生成溯源树,研究论文发展脉络
Chat Paper
正在生成论文摘要