Deep Learning For Lidar Waveforms With Multiple Returns
28TH EUROPEAN SIGNAL PROCESSING CONFERENCE (EUSIPCO 2020)(2021)
摘要
We present LiDARNet, a novel data driven approach to LiDAR waveform processing utilising convolutional neural networks to extract depth information. To effectively leverage deep learning, an efficient LiDAR toolchain was developed, which can generate realistic waveform datasets based on either specific experimental parameters or synthetic scenes at scale. This enables us to generate a large volume of waveforms in varying conditions with meaningful underlying data. To validate our simulation approach, we model a super resolution benchmark and cross-validate the network with real unseen data. We demonstrate the ability to resolve peaks in close proximity, as well as to extract multiple returns from waveforms with low signal-to-noise ratio simultaneously with over 99% accuracy. This approach is fast, flexible and highly parallelizable for arrayed imagers. We provide explainability in the deep learning process by matching intermediate outputs to a robust underlying signal model.
更多查看译文
关键词
LiDAR, Deep Learning, Convolutional Neural Networks, Super-Resolution, Time-of-Flight Imaging
AI 理解论文
溯源树
样例
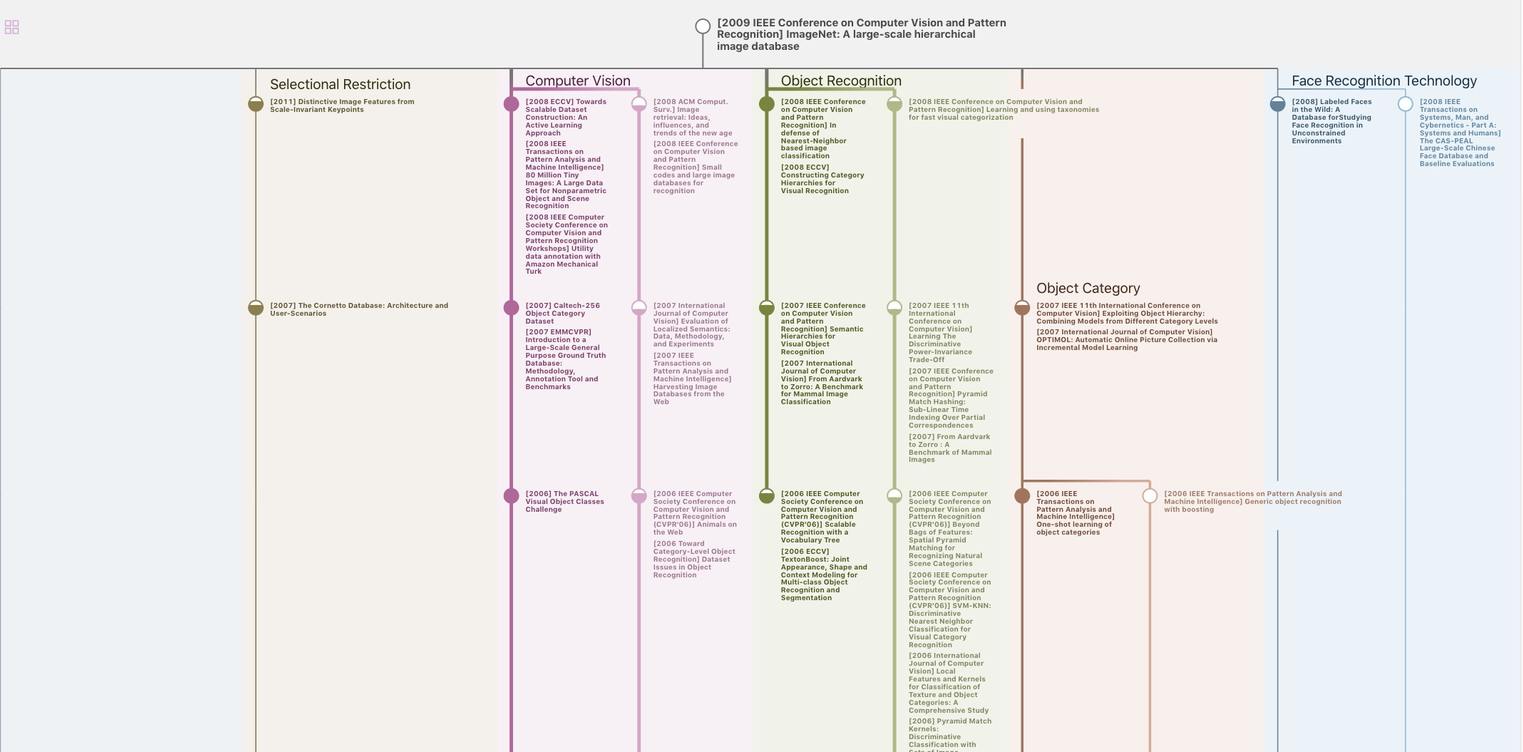
生成溯源树,研究论文发展脉络
Chat Paper
正在生成论文摘要