MA-Net: A Multi-Scale Attention Network for Liver and Tumor Segmentation
IEEE access(2020)
摘要
Automatic assessing the location and extent of liver and liver tumor is critical for radiologists, diagnosis and the clinical process. In recent years, a large number of variants of U-Net based on Multi-scale feature fusion are proposed to improve the segmentation performance for medical image segmentation. Unlike the previous works which extract the context information of medical image via applying the multi-scale feature fusion, we propose a novel network named Multi-scale Attention Net (MA-Net) by introducing self-attention mechanism into our method to adaptively integrate local features with their global dependencies. The MA-Net can capture rich contextual dependencies based on the attention mechanism. We design two blocks: Position-wise Attention Block (PAB) and Multi-scale Fusion Attention Block (MFAB). The PAB is used to model the feature interdependencies in spatial dimensions, which capture the spatial dependencies between pixels in a global view. In addition, the MFAB is to capture the channel dependencies between any feature map by multi-scale semantic feature fusion. We evaluate our method on the dataset of MICCAI 2017 LiTS Challenge. The proposed method achieves better performance than other state-of-the-art methods. The Dice values of liver and tumors segmentation are 0.960 ± 0.03 and 0.749 ± 0.08 respectively.
更多查看译文
关键词
CT,liver tumor segmentation,deep learning,attention mechanism,context information
AI 理解论文
溯源树
样例
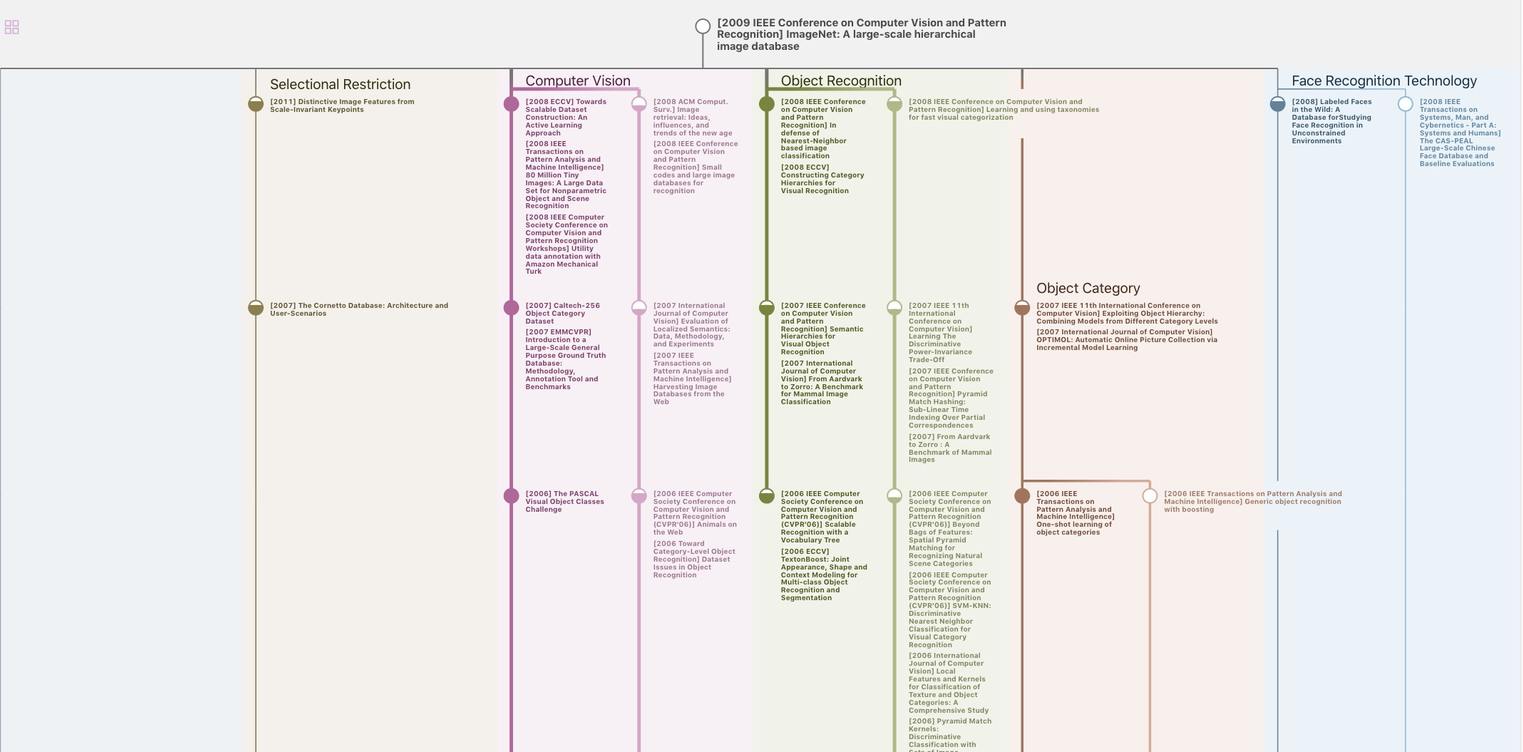
生成溯源树,研究论文发展脉络
Chat Paper
正在生成论文摘要