On the benefits of domain adaptation techniques for quality of transmission estimation in optical networks
Journal of Optical Communications and Networking(2021)
摘要
Machine learning (ML) is increasingly applied in optical network management, especially in cross-layer frameworks where physical layer characteristics may trigger changes at the network layer due to transmission performance measurements (quality of transmission, QoT) monitored by optical equipment. Leveraging ML-based QoT estimation approaches has proven to be a promising alternative to exploiting classical mathematical methods or transmission simulation tools. However, supervised ML models rely on large representative training sets, which are often unavailable, due to the lack of the necessary telemetry equipment or of historical data. In such cases, it can be useful to use training data collected from a different network. Unfortunately, the resulting models may be uneffective when applied to the current network, if the training data (the source domain) is not well representative of the network under study (the target domain). Domain adaptation (DA) techniques aim at tackling this issue, to make possible the transfer of knowledge among different networks. This paper compares several DA approaches applied to the problem of estimating the QoT of an optical lightpath using a supervised ML approach. Results show that, when the number of samples from the target domain is limited to a few dozen, DA approaches consistently outperform standard supervised ML techniques.
更多查看译文
关键词
quality of transmission,ML-based QoT estimation approaches,supervised ML approach,optical lightpath,source domain,training data,historical data,necessary telemetry equipment,representative training sets,supervised ML models,transmission simulation tools,classical mathematical methods,optical equipment,transmission performance measurements,network layer,physical layer characteristics,cross-layer frameworks,optical network management,machine learning,domain adaptation techniques,standard supervised ML techniques,target domain
AI 理解论文
溯源树
样例
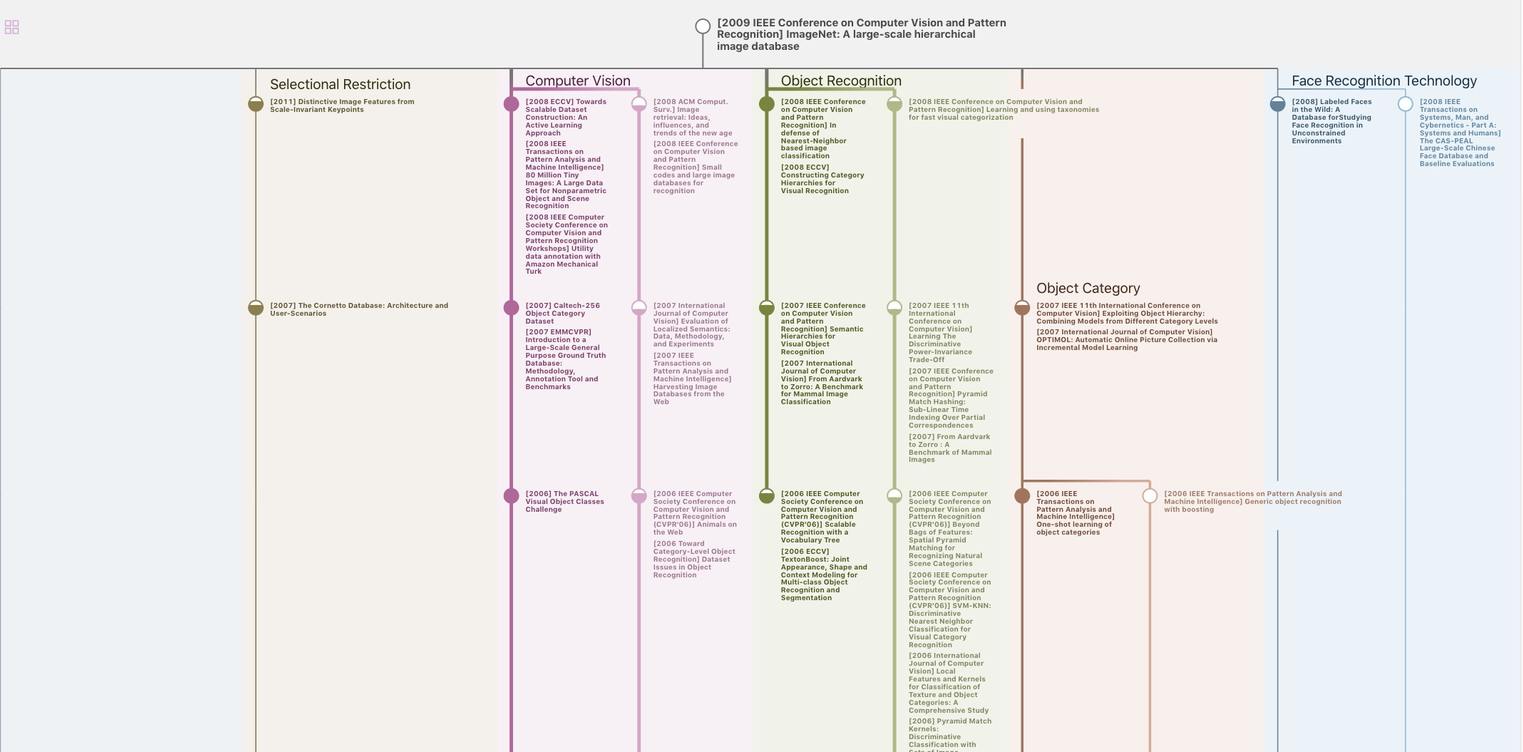
生成溯源树,研究论文发展脉络
Chat Paper
正在生成论文摘要