Detection Of Anomalous Vehicles Using Physics Of Traffic
VEHICULAR COMMUNICATIONS(2021)
摘要
The world is embracing the presence of connected autonomous vehicles which are expected to play a major role in the future of intelligent transport systems. Given such connectivity, vehicles in the networks are vulnerable to making incorrect decisions due to anomalous data. No sophisticated attacks are required; just a vehicle reporting anomalous speeds would be enough to disrupt the entire traffic flow. Detection of such anomalies is vital to ensure the security of a vehicular network. We propose the use of traffic flow theory for anomalous data detection in vehicular networks, by evaluating the consistency of microscopic parameters which are derived by traffic flow theory (i.e. speed and space-headway) with macroscopic views of traffic under different traffic conditions. Though a little attention has been given to using traffic flow properties to determine anomalous basic safety message (BSM) data, the fundamental nature of traffic flow properties makes it a robust assessment tool. Usually, traffic flow data are determined through roadside units (RSUs) such as cameras and loop detectors; they are financially impractical to roll out on an entire network. Therefore, the method proposed in this study establishes traffic flow data that are used as "ground truth" through RSUs if available, or by the vehicles' own sensor systems. The numerical results indicate that the proposed method provides extremely reliable and consistent predictions of anomalous BSM data. The more the road segment is congested, the higher the accuracy of the anomalous space-headway detection. The anomalous speed detection performs robustly well across all the traffic conditions. The study also finds that both global and local ground truths provide consistent results. (C) 2020 Elsevier Inc. All rights reserved.
更多查看译文
关键词
Anomalous nodes, Traffic flow theory, VANET security
AI 理解论文
溯源树
样例
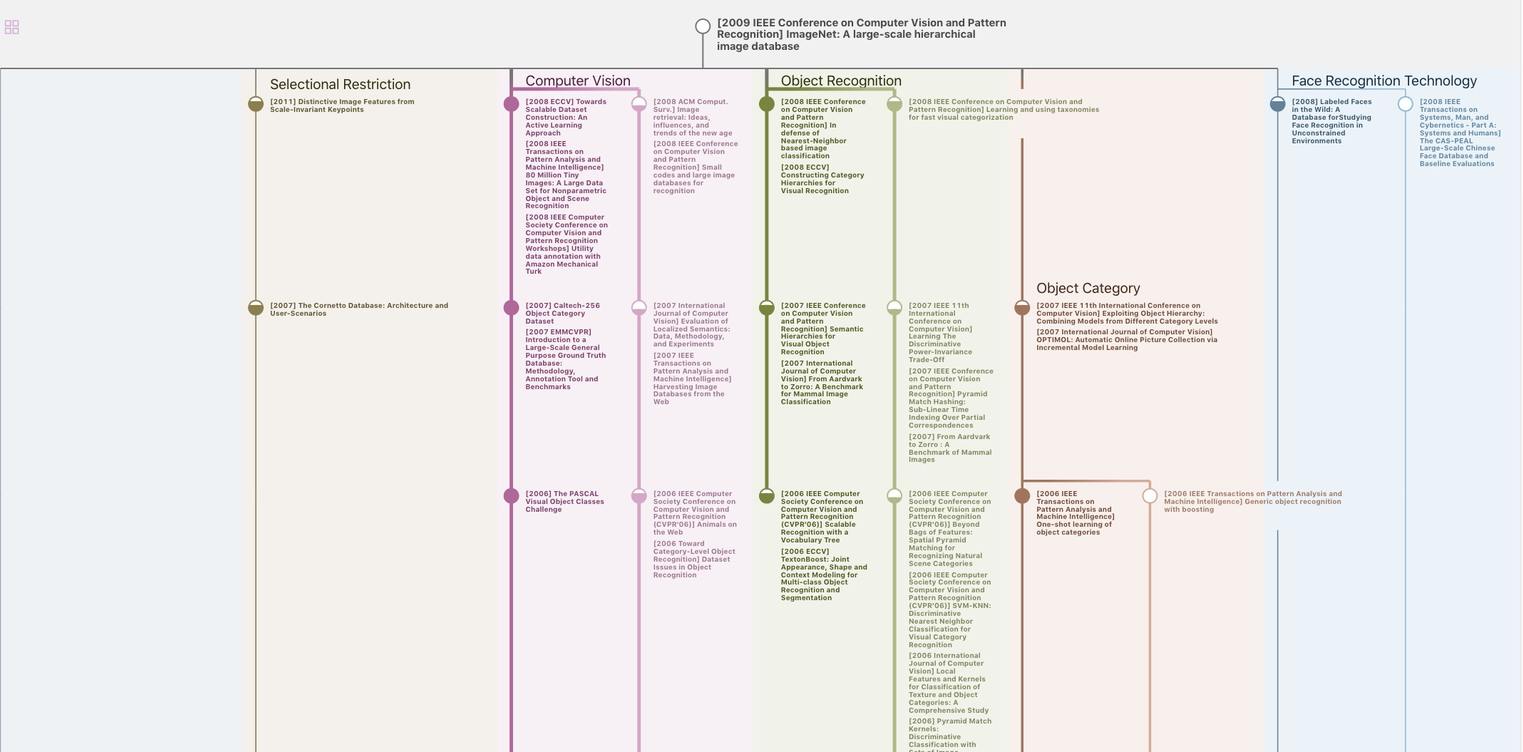
生成溯源树,研究论文发展脉络
Chat Paper
正在生成论文摘要