Deep autoencoder for false positive reduction in handgun detection
NEURAL COMPUTING & APPLICATIONS(2020)
摘要
In an object detection system, the main objective during training is to maintain the detection and false positive rates under acceptable levels when the model is run over the test set. However, this typically translates into an unacceptable rate of false alarms when the system is deployed in a real surveillance scenario. To deal with this situation, which often leads to system shutdown, we propose to add a filter step to discard part of the new false positive detections that are typical of the new scenario. This step consists of a deep autoencoder trained with the false alarm detections generated after running the detector over a period of time in the new scenario. Therefore, this step will be in charge of determining whether the detection is a typical false alarm of that scenario or whether it is something anomalous for the autoencoder and, therefore, a true detection. In order to decide whether a detection must be filtered, three different approaches have been tested. The first one uses the autoencoder reconstruction error measured with the mean squared error to make the decision. The other two use the k -NN ( k -nearest neighbors) and one-class SVMs (support vector machines) classifiers trained with the autoencoder vector representation. In addition, a synthetic scenario has been generated with Unreal Engine 4 to test the proposed methods in addition to a dataset with real images. The results obtained show a reduction in the number of false positives between 22.5
更多查看译文
关键词
Handgun detection, False positive reduction, Autoencoder, One-class classification
AI 理解论文
溯源树
样例
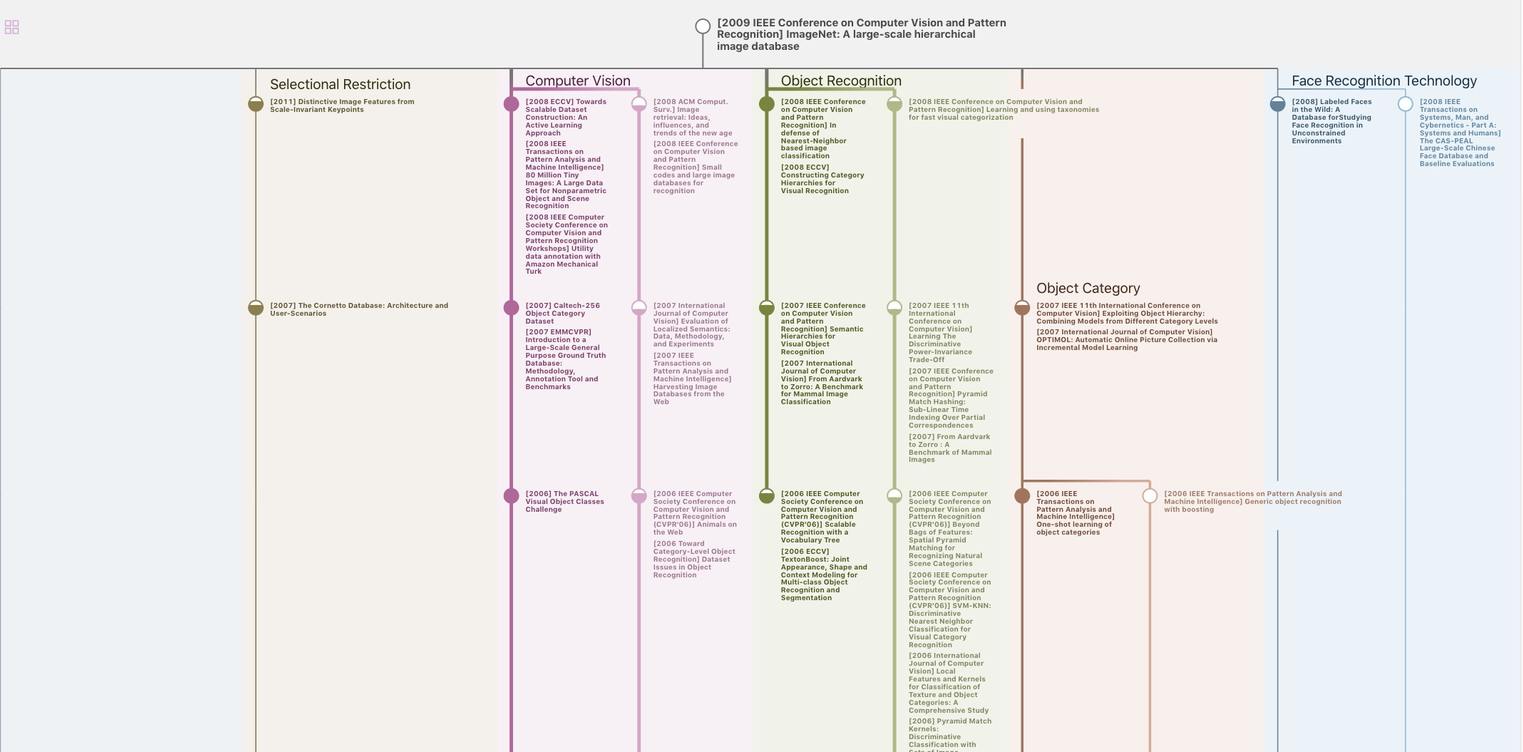
生成溯源树,研究论文发展脉络
Chat Paper
正在生成论文摘要