Text Keyword Extraction Based on Multi-dimensional Features.
WISA(2020)
摘要
Keyword extraction is a fundamental task of text mining, so extracting high-quality keywords is of great significance. Typical keyword extraction algorithms usually rely on the statistical features, but lack of the semantic information. At the same time, the supervised keyword extraction algorithms rely too much on sample labeling. Therefore, in this paper, an unsupervised keyword extraction algorithm based on multi-dimensional features called MDFKE is proposed, which combines statistical features, external knowledge-based features and semantic features. MDFKE mainly studies the semantic information of candidate keywords. LDA model is used to obtain text topic, and Word2vec word embedding is used to generate word vectors. Based on these, the similarity between candidate keyword and text topic is quantified as semantic feature. Nine specific features are extracted from five aspects: term frequency, length, position, external knowledge base, and semantics. Finally, this paper clusters on feature vectors to obtain the final keyword set. The experiment turns out that, compared with traditional keyword extraction algorithms based on statistical features, MDFKE can significantly improve extraction performance, and can also make up for the shortage of supervised learning overly relying on labels.
更多查看译文
关键词
text keyword extraction,features,multi-dimensional
AI 理解论文
溯源树
样例
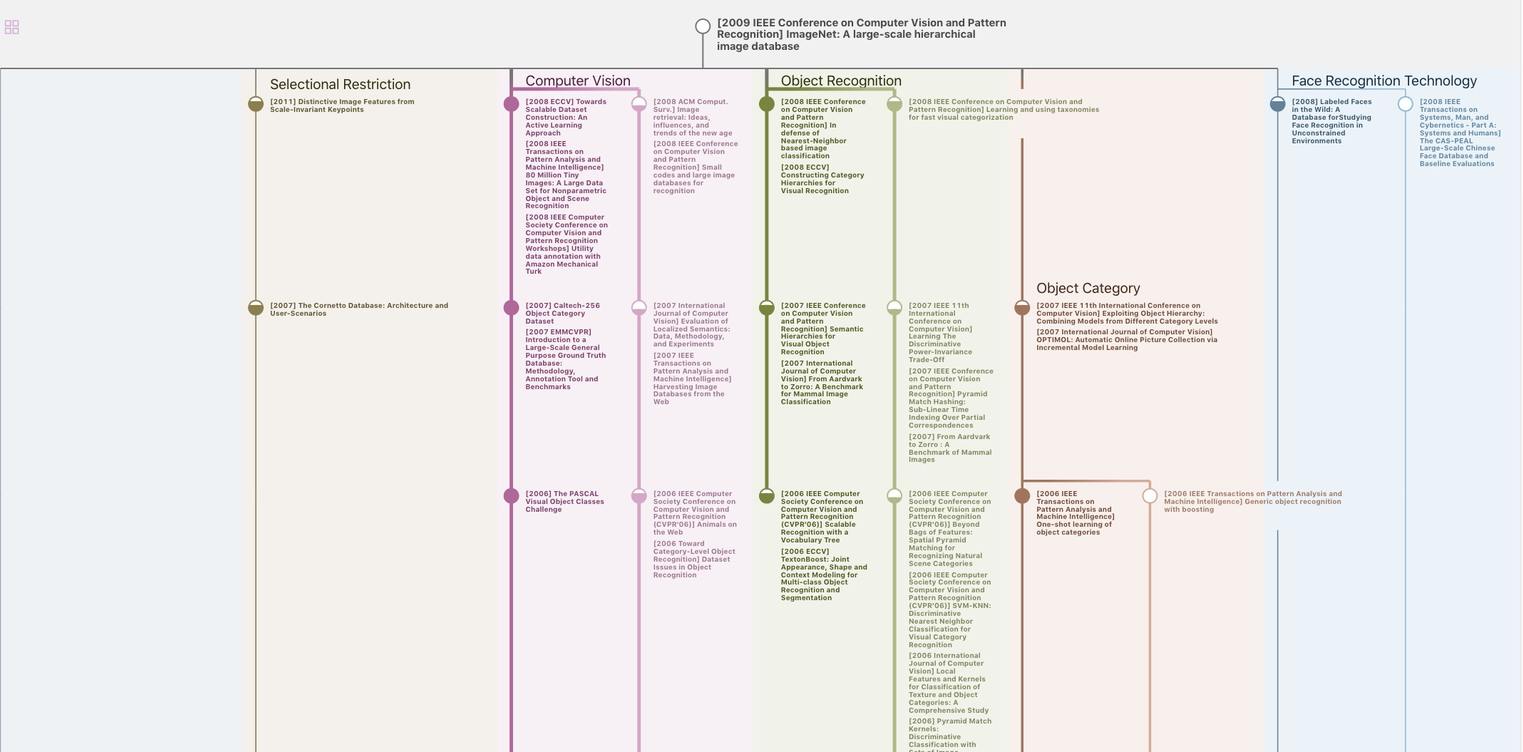
生成溯源树,研究论文发展脉络
Chat Paper
正在生成论文摘要