Multi-Scale Feature Enhanced Domain Adaptive Object Detection For Power Transmission Line Inspection
IEEE ACCESS(2020)
摘要
Domain adaptive object detection aims to build an object detector for the unlabeled target domain by transferring knowledge from a well-labeled source domain, which can alleviate the problem of cumbersome labeling of object detection in cross-scene power transmission line inspection. Remarkable advances are made recently by mitigating distributional shifts via hierarchical domain feature alignment training of detection networks. However, domain adaptive object detection is still limited in learning the invariance representation of multi-scale features. Specifically, the scale of objects varies in the scenes of aerial inspection, which hinders the knowledge transfer from the labeled source domain. In this paper, we propose a multi-scale feature enhanced domain adaptation method for cross-domain object detection of power transmission lines inspection. The proposed method consists of two components: 1) Multi-Scale Fusion Feature Alignment module (MSFA) to strengthen similar representation characteristics of different scales object in domain adaptive by utilizing context information conveyed from other levels; 2) Multi-Scale Consistency Regularization module (MSCR) to jointly optimize the multi-scale feature learning of each level, which promotes domain invariant feature learning at each level. Experimental results demonstrate that our method significantly increases the performance of the object detector in several cross-scene transmission line inspection tasks.
更多查看译文
关键词
Object detection,Inspection,Feature extraction,Power transmission lines,Detectors,Polymers,Task analysis,Domain adaptation,object detection,deep learning,power transmission lines inspection,fault detection,insulator
AI 理解论文
溯源树
样例
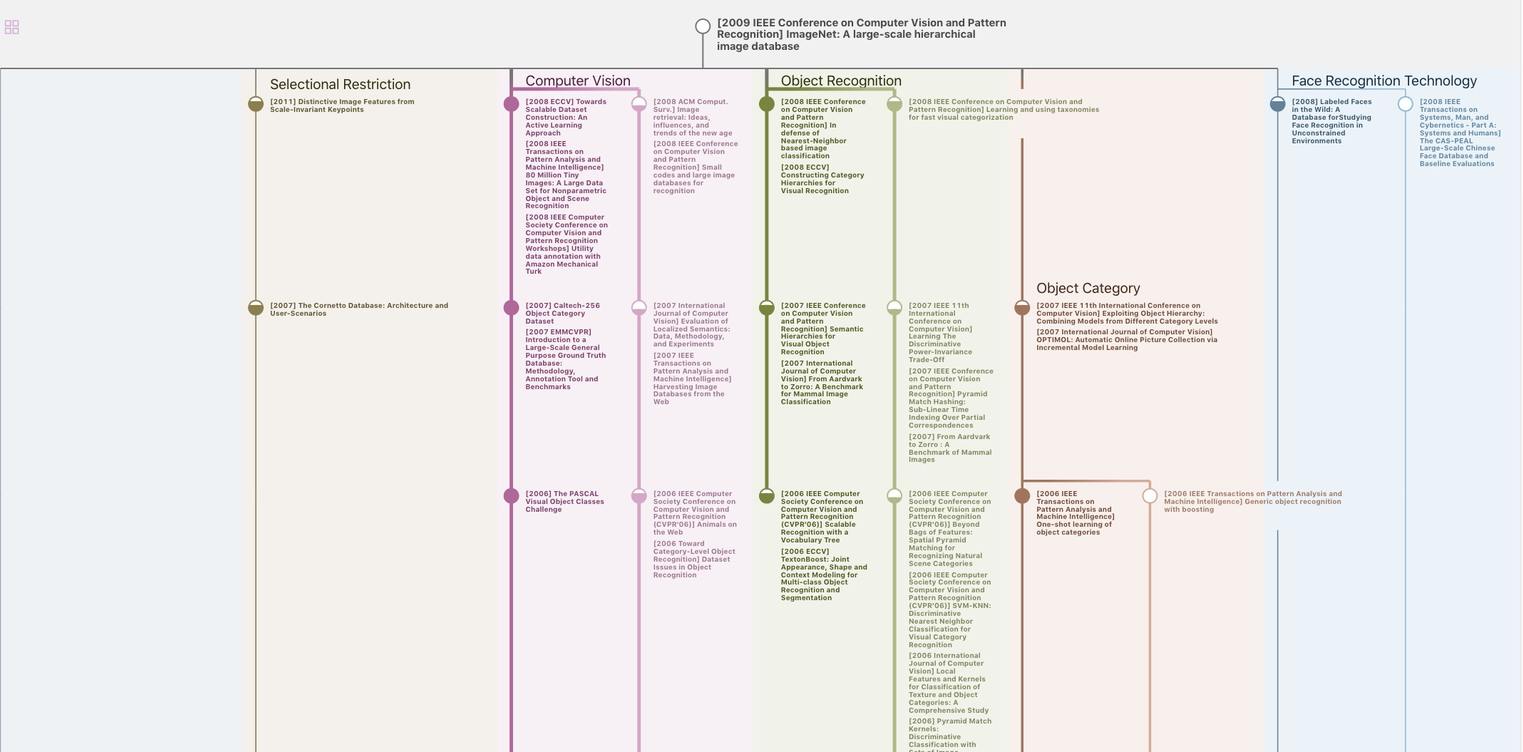
生成溯源树,研究论文发展脉络
Chat Paper
正在生成论文摘要