Tri-FeatureNet: An Adversarial Learning-Based Invariant Feature Extraction for Sleep Staging using Single-Channel EEG
ISCAS(2020)
摘要
The difference in EEG data among subjects and sessions is an essential factor affecting the accuracy in neural signal analysis. This paper proposed a Tri-FeatureNet, an adversarial learning-based feature extraction algorithm to learn representations invariant to subjects and sessions for sleep staging. The proposed work improves the algorithm's robustness to individual differences. The invariant features are combined with the subject-specified features and the temporal features to further compensate for the loss of sleep information during adversarial training. The proposed model makes use of the temporal information in EEG signals and sleep staging sequences at the same time. This is the first time that adversarial training was proposed to extract the task-related features for different subjects and different sessions in sleep staging. The proposed algorithm was implemented in a portable sleep staging system. Experimental results illustrated an 82.9% ACC in sleep staging task with a single-channel EEG signal.
更多查看译文
关键词
temporal information,staging sequences,adversarial training,task-related features,different sessions,portable sleep staging system,sleep staging task,single-channel EEG signal,Tri-FeatureNet,adversarial learning-based invariant feature extraction,neural signal analysis,adversarial learning-based feature extraction algorithm,invariant features,subject-specified features,temporal features,sleep information
AI 理解论文
溯源树
样例
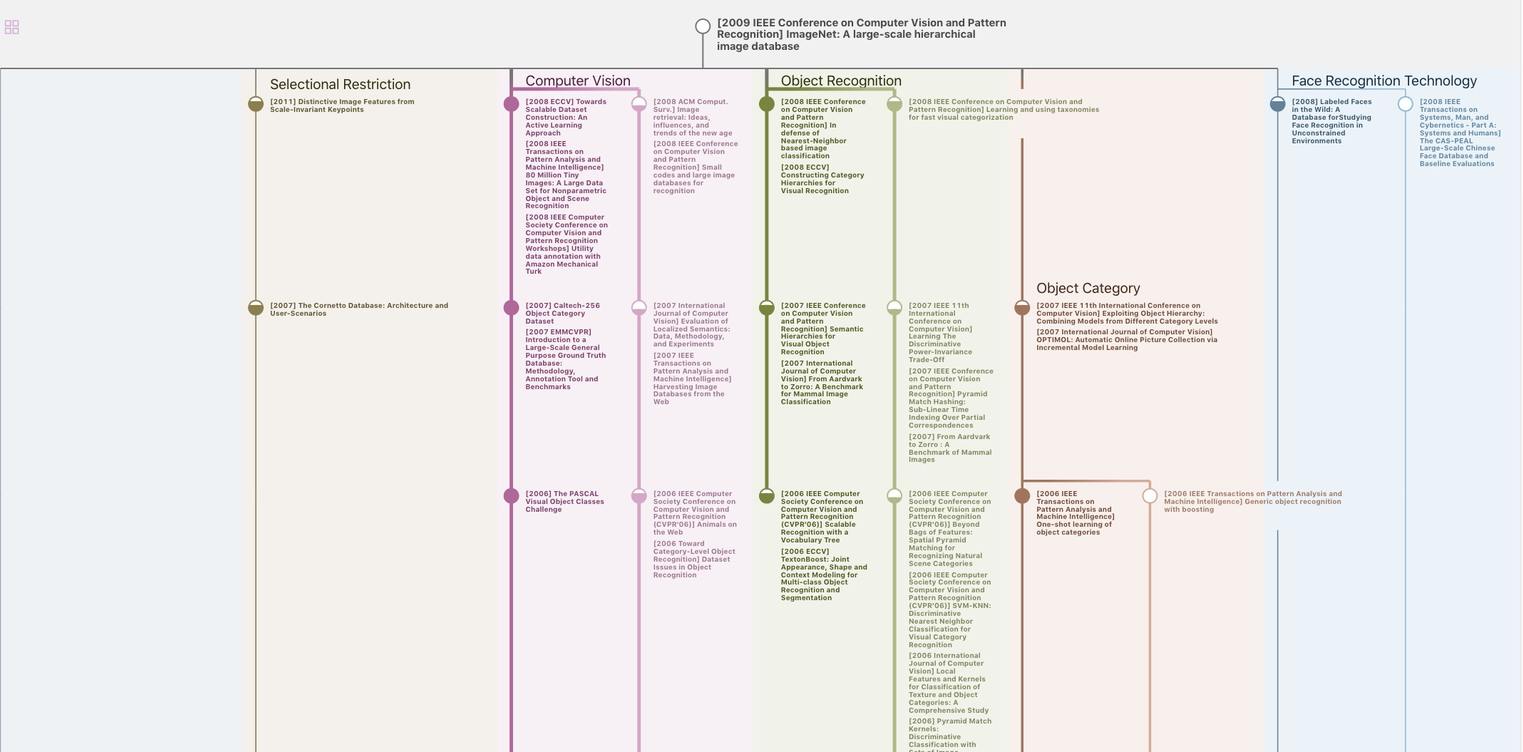
生成溯源树,研究论文发展脉络
Chat Paper
正在生成论文摘要