Texture-sensitive Prediction of Micro-Spring Performance Using Gaussian Process Models Calibrated to Finite Element Simulations
Materials & Design(2021)
摘要
This paper explores the viability and benefits of building a computationally low-cost surrogate model relating the mechanical performance of micro-springs to crystallographic texture and single-crystal elastic constants. This task is accomplished by leveraging advances in (i) computationally-expensive finite element (FE) models that explicitly incorporates the constitutive response of individual grains in to simulate the overall mechanical response of the micro-spring, (ii) efficient parametrization of the extremely large space of textures using Fourier basis (i.e., generalized spherical harmonics), (iii) sequential design of FE simulations to maximize model fidelity while also minimizing the overall computational expense incurred in generating the data, and (iv) the use of Gaussian process models for incorporating uncertainty quantification into the development of the desired surrogate model. The strategy implemented in this work proved to be remarkably efficient in producing the desired surrogate model. The utility of the surrogate model established in this work is demonstrated with a case study addressing an inverse solution for the specific crystallographic texture that maximizes the micro-spring performance for a selected material.
更多查看译文
关键词
Finite element models,Polycrystal microstructures,Texture,Generalized spherical harmonics,Gaussian process regression,Sequential design
AI 理解论文
溯源树
样例
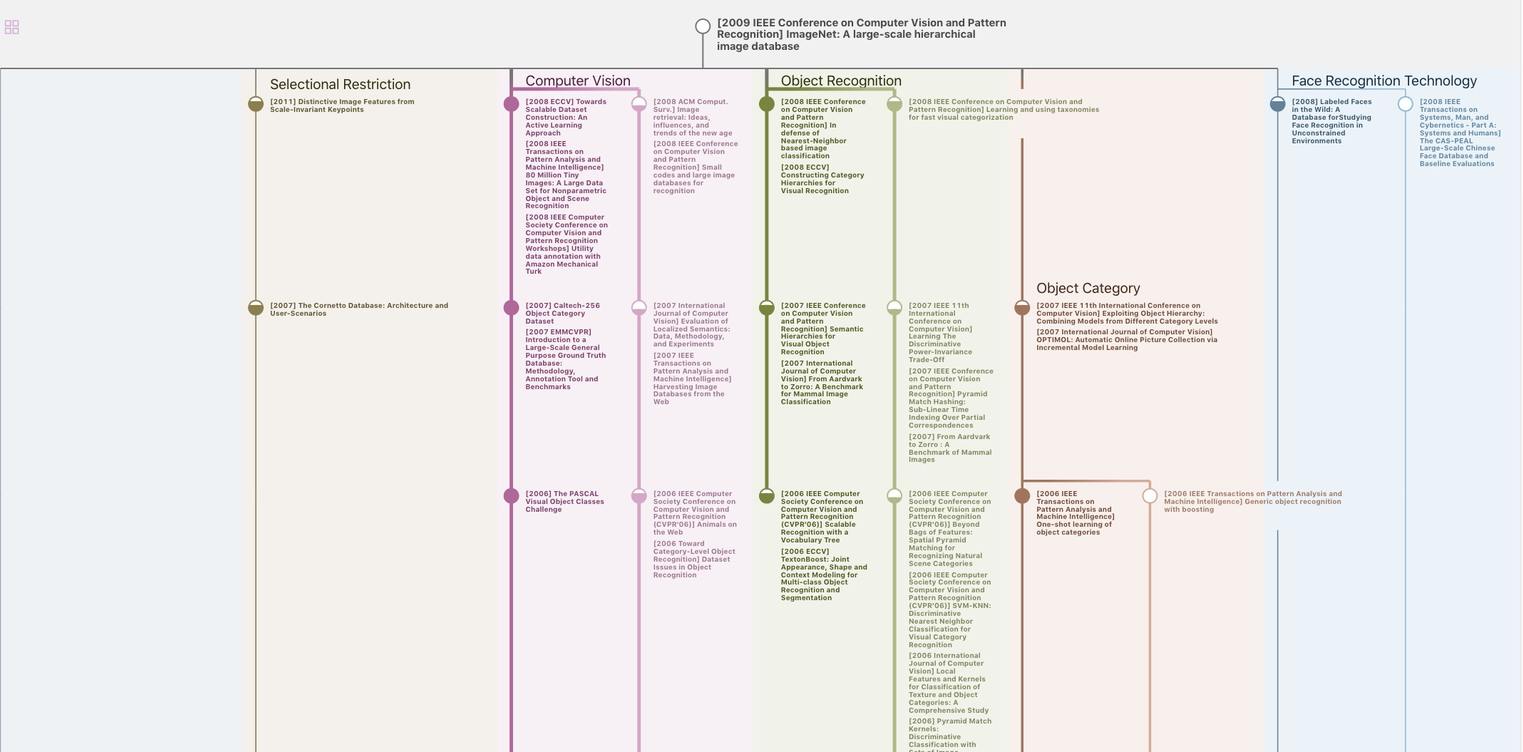
生成溯源树,研究论文发展脉络
Chat Paper
正在生成论文摘要