A CycleGAN Accelerator for Unsupervised Learning on Mobile Devices
ISCAS(2020)
摘要
Cycle-consistent generative adversarial networks (CycleGANs) have been commonly used for unsupervised-learning applications, especially for image-to-image translation. A CycleGAN has more complex dataflow since it features two generator-discriminator pairs. Massive external memory access also results in a long latency for both training and inference. Data structure for transposed convolution also needs to be tailored. This paper presents the first dedicated CycleGAN accelerator for energy-constrained mobile applications. The numbers of external and internal memory accesses are reduced by 98.3% and 68.3% through spatial data reuse, input feature map reuse, and local data reuse. The computational complexity is reduced by 79.4% by skipping zeros in the transposed convolutional layers. An architecture with two processing cores is proposed to improve the utilization by 2x. Designed in a 40-nm CMOS technology, the proposed CycleGAN accelerator dissipates 445 mW at 227 MHz from a 0.9-V supply. It achieves a 38x higher throughput-to-area ratio and 127x higher energy efficiency than a GPU.
更多查看译文
关键词
unsupervised-learning applications,image-to-image translation,complex dataflow,generator-discriminator pairs,massive external memory access,data structure,transposed convolution,energy-constrained mobile applications,external memory accesses,internal memory accesses,spatial data reuse,input feature map reuse,local data reuse,computational complexity,transposed convolutional layers,unsupervised learning,mobile devices,cycle-consistent generative adversarial networks,CycleGAN accelerator,CMOS technology,power 445.0 mW,frequency 227.0 MHz,voltage 0.9 V,size 40 nm
AI 理解论文
溯源树
样例
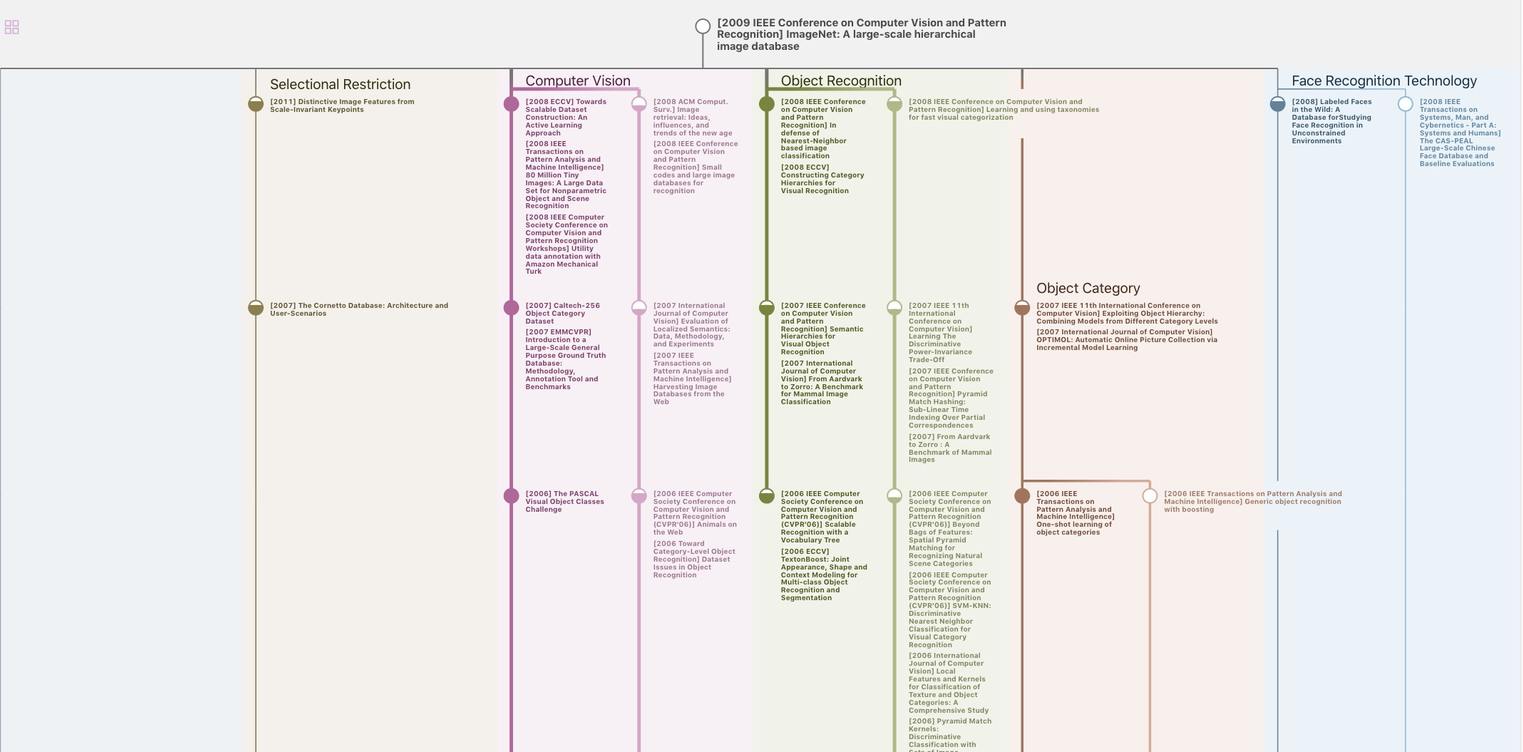
生成溯源树,研究论文发展脉络
Chat Paper
正在生成论文摘要