Analysis of activation maps through global pooling measurements for texture classification
Information Sciences(2021)
摘要
We analyzed the effects of global pooling measurements on extracting relevant texture information from a given set of activation maps. Initially, using a layer-by-layer approach (GP-CNN), we experimentally demonstrated that layers at various depth levels could provide high-quality texture information. Based on this finding, we developed RankGP-CNN, a method that performs multi-layer feature extraction. More specifically, RankGP-CNN treats every CNN model as a vast collection of deep composite functions, where each function computes a 2D activation map for every input image. A feature ranking approach then assigns a score to each deep composite function by processing the activation maps generated for a particular dataset bank. Eventually, RankGP-CNN uses the top-ranked deep composite functions to compute feature vectors for different texture datasets. Experiments on a dedicated classifier showed that RankGP-CNN achieves good results and can adapt to different texture problems. Finally, we present RankGP-3M-CNN as the version of RankGP-CNN that considers multiple CNN models. Overall, RankGP-3M-CNN achieves promising results with the advantage of only using the default scale of the input images.
更多查看译文
关键词
Convolutional neural networks,Transfer learning,Texture analysis
AI 理解论文
溯源树
样例
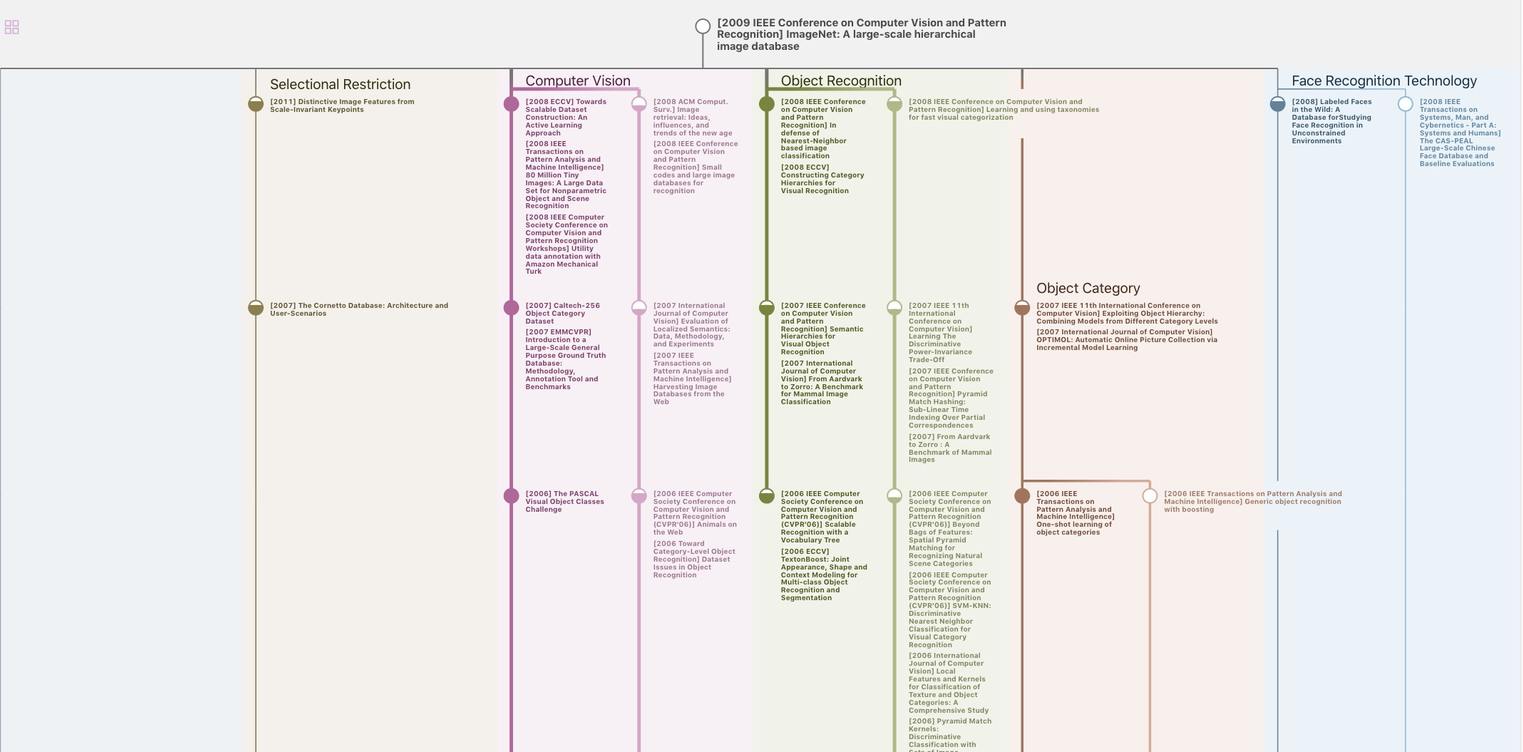
生成溯源树,研究论文发展脉络
Chat Paper
正在生成论文摘要