Trace-SRL: A Framework for Analysis of Microlevel Processes of Self-Regulated Learning From Trace Data
IEEE Transactions on Learning Technologies(2020)
摘要
The recent focus on learning analytics (LA) to analyze temporal dimensions of learning holds the promise of providing insights into latent constructs, such as learning strategy, self-regulated learning (SRL), and metacognition. These methods seek to provide an enriched view of learner behaviors beyond the scope of commonly used correlational or cross-sectional methods. In this article, we present a methodological sequence of techniques that comprises: 1) the strategic clustering of learner types; 2) the use of microlevel processing to transform raw trace data into SRL processes; and 3) the use of a novel process mining algorithm to explore the generated SRL processes. We call this the “Trace-SRL” framework. Through this framework, we explored the use of microlevel process analysis and process mining (PM) techniques to identify optimal and suboptimal traits of SRL. We analyzed trace data collected from online activities of a sample of nearly 300 computer engineering undergraduate students enrolled on a course that followed a flipped class-room pedagogy. We found that using a theory-driven approach to PM, a detailed account of SRL processes emerged, which could not be obtained from frequency measures alone. PM, as a means of learner pattern discovery, promises a more temporally nuanced analysis of SRL. Moreover, the results showed that more successful students regularly engage in a higher number of SRL behaviors than their less successful counterparts. This suggests that not all students are sufficiently able to regulate their learning, which is an important finding for both theory and LA, and future technologies that support SRL.
更多查看译文
关键词
First-order Markov models (FOMMs),learning analytics (LA),microlevel process analysis,process mining (PM),self-regulated learning (SRL).
AI 理解论文
溯源树
样例
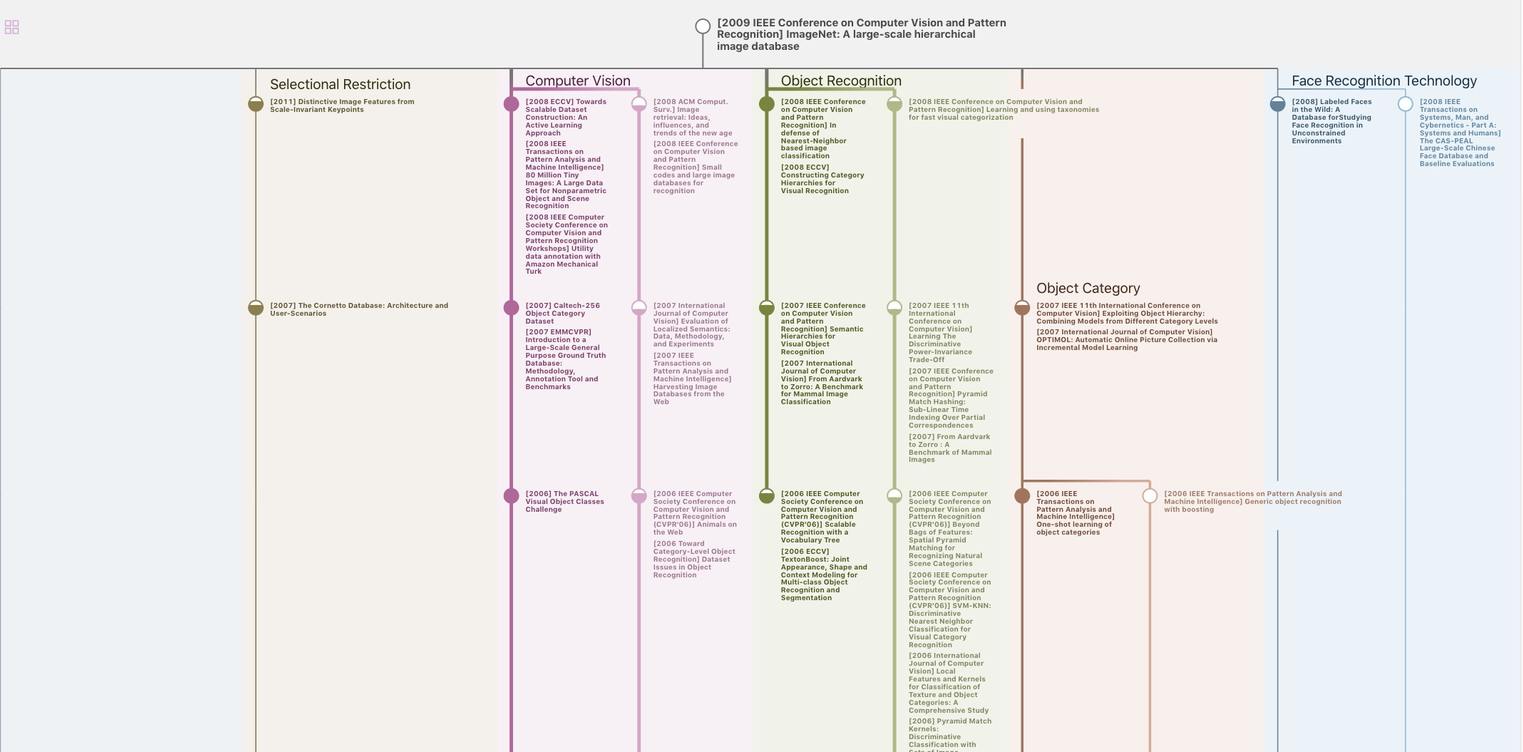
生成溯源树,研究论文发展脉络
Chat Paper
正在生成论文摘要