Towards Neuron Segmentation from Macaque Brain Images: A Weakly Supervised Approach.
medical image computing and computer-assisted intervention(2020)
摘要
The advance of microscopic imaging technology has enabled the observation of primate brain in its entirety and at single-neuron resolution. It is then an urgent need to develop means for automated analyses of these brain images, e.g. neuron segmentation. Deep learning is demonstrated an appealing approach for segmentation of natural images, but the success of deep learning is highly dependent on the large-scale and well-built training data that are costly to collect. In this paper, we take a step towards the goal of neuron segmentation from primate brain images, using a weakly supervised approach. We build – to our best knowledge – the first dual-channel three-dimensional image dataset of macaque brain for neuron segmentation. We propose two kinds of “weak” labels, i.e. central points and rough masks, to prepare training data with an affordable cost. Accordingly, we design a weakly supervised learning method for neuron instance segmentation where instances can be easily extracted from the predicted peak-shape probability maps. Experimental results have shown the effectiveness of our approach. We also verify the efficiency of the proposed method on a public nuclei dataset. Our dataset and code have been published at https://braindata.bitahub.com/.
更多查看译文
关键词
Instance segmentation, Macaque brain, Weakly supervised learning
AI 理解论文
溯源树
样例
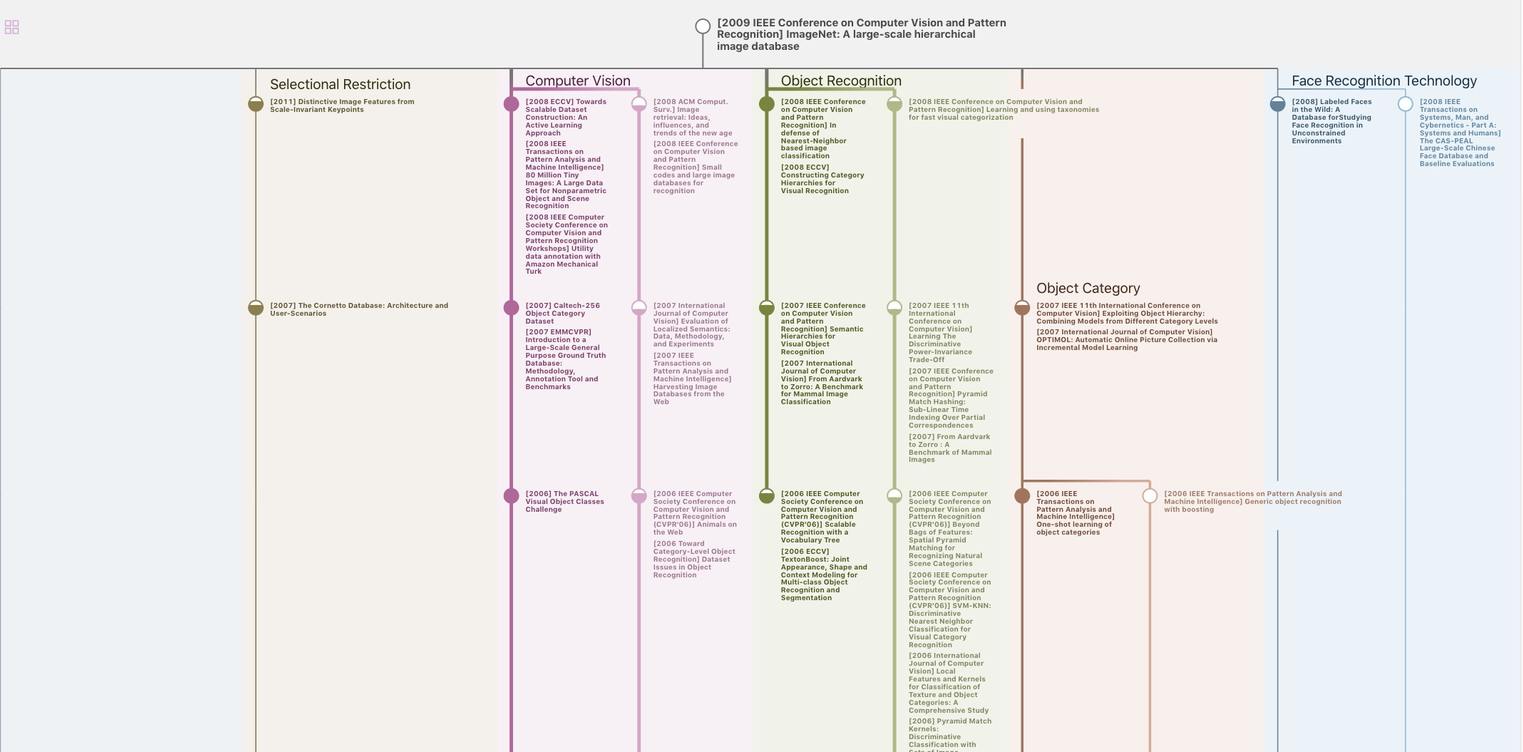
生成溯源树,研究论文发展脉络
Chat Paper
正在生成论文摘要