A Novel Conversion Method for Spiking Neural Network using Median Quantization
ISCAS(2020)
摘要
Artificial Neural Networks (ANNs) have achieved great success in the field of computer vision and language understanding. However, it is difficult to deploy these deep learning models on mobile devices because of its massive energy consumption and memory occupation. For another way, highly inspired from biological brain, spiking neural networks (SNNs), are often referred to as the 3-th generation of neural network for its potential superiority in cognitive learning and energy efficiency. Nevertheless, training a deep SNN remains a big challenge. In this paper, we propose a quantized training algorithm for ANNs to minimize spike approximation error, and provide two (temporally or spatially) rate-based conversion methods for SNNs, both of which can be easily mapped to specific neuromorphic platforms. Besides, this novel method can be generalized to various network architectures and adapted to dynamic quantization demand. Experimental results on MNIST and CIFAR-10 dataset demonstrate that the proposed deep spiking neural networks yield the state-of-the-art classification accuracy and need much less operations compared with their ANN counterparts. Our source code will be available upon request for the academic purpose.
更多查看译文
关键词
Artificial Neural Networks (ANNs),Spiking Neural Networks (SNNs),Dynamic Quantization,Neuromorphic Hardware
AI 理解论文
溯源树
样例
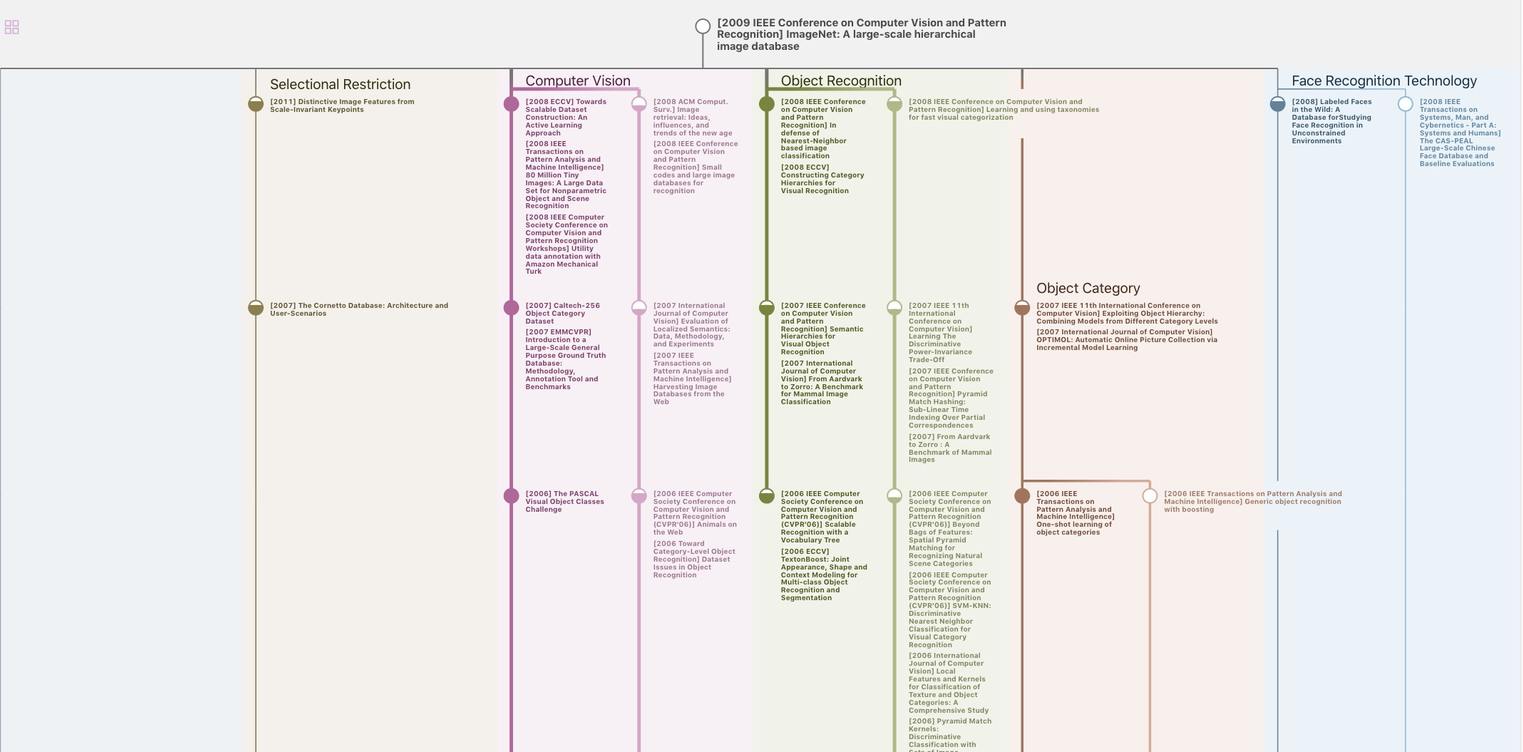
生成溯源树,研究论文发展脉络
Chat Paper
正在生成论文摘要