Variance Error Of Multi-Classification Based Anomaly Detection For Time Series Data
JOURNAL OF COMPUTATIONAL METHODS IN SCIENCES AND ENGINEERING(2021)
摘要
For abnormal detection of time series data, the supervised anomaly detection methods require labeled data. While the range of outlier factors used by the existing semi-supervised methods varies with data, model and time, the threshold for determining abnormality is difficult to obtain, in addition, the computational cost of the way to calculate outlier factors from other data points in the data set is also very large. These make such methods difficult to practically apply. This paper proposes a framework named LSTM-VE which uses clustering combined with visualization method to roughly label normal data, and then uses the normal data to train long short-term memory (LSTM) neural network for semi-supervised anomaly detection. The variance error (VE) of the normal data category classification probability sequence is used as outlier factor. The framework enables anomaly detection based on deep learning to be practically applied and using VE avoids the shortcomings of existing outlier factors and gains a better performance. In addition, the framework is easy to expand because the LSTM neural network can be replaced with other classification models. Experiments on the labeled and real unlabeled data sets prove that the framework is better than replicator neural networks with reconstruction error (RNN-RS) and has good scalability as well as practicability.
更多查看译文
关键词
Time series data, outlier detection, long short-term memory, clustering
AI 理解论文
溯源树
样例
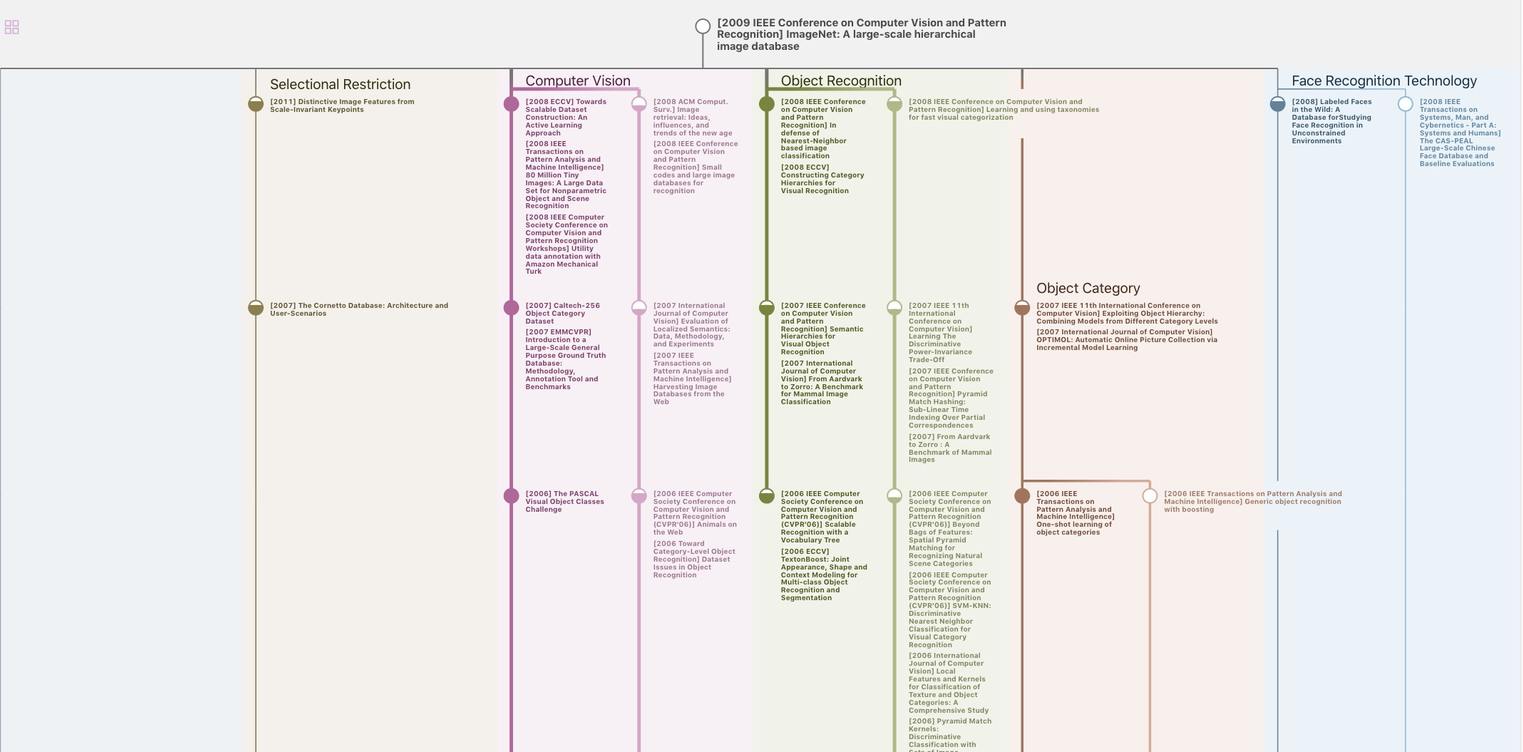
生成溯源树,研究论文发展脉络
Chat Paper
正在生成论文摘要